MultiModal AI Integration: Beyond Text-Based Interactions
Explore how businesses are leveraging multimodal AI to transform customer experiences in 2025. Learn about text, voice, image, and video integration strategies, real-world applications, and implementation best practices.
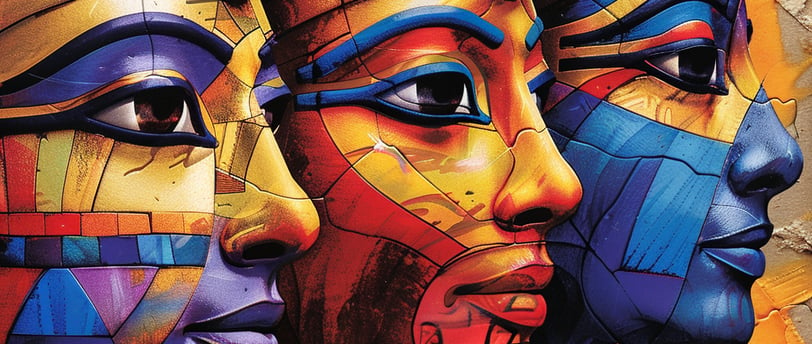
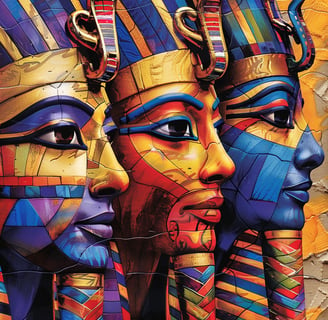
Imagine a world where you can show your AI assistant a photo of ingredients in your refrigerator, ask verbally what you could cook, and receive not just a text recipe but a holographic demonstration of cooking techniques. This isn't science fiction—it's the reality of multimodal AI in 2025. The landscape of artificial intelligence has evolved dramatically over the past few years, moving far beyond the simple text-based interactions that once defined our relationship with AI systems. Today's multimodal AI integrations represent a fundamental shift in how we communicate with technology, enabling machines to understand, interpret, and generate content across multiple sensory channels simultaneously. As businesses race to implement these advanced systems, we're witnessing a transformation in customer experiences, operational efficiencies, and creative possibilities. In this comprehensive guide, we'll explore the current state of multimodal AI integration, examine its real-world applications, and look ahead to what the future might hold for this revolutionary technology.
Understanding Multimodal AI: The Convergence of Senses
Multimodal AI refers to artificial intelligence systems that can process and generate information across multiple forms of communication or "modes"—including text, images, audio, video, and even tactile feedback. Unlike traditional AI models that specialized in single-mode interactions, today's advanced systems can seamlessly blend these different modalities to create more natural, intuitive user experiences. The foundation of multimodal AI lies in its ability to understand context across different types of input, creating connections between what we say, what we see, and how we interact with the world around us. This technological evolution represents a significant leap forward in making AI more accessible and valuable for everyday users and businesses alike. Modern multimodal systems utilize sophisticated neural networks and deep learning architectures that can recognize patterns across different forms of data, breaking down the traditional silos that once separated text analysis from image recognition or voice processing. The resulting integrated experience more closely mimics human cognitive abilities, where our brains naturally process multiple sensory inputs simultaneously to form comprehensive understanding.
The Evolution from Unimodal to Multimodal AI
The journey toward multimodal AI began with single-mode, or unimodal, systems that specialized in processing specific types of data. Early AI applications were primarily text-based, with natural language processing (NLP) driving advancements in everything from translation services to customer support chatbots. As computer vision technologies developed in parallel, image recognition systems became increasingly sophisticated, enabling applications from facial recognition to medical diagnostics. Similarly, voice recognition and audio processing technologies evolved to power virtual assistants and transcription services. The breakthrough came when researchers began developing frameworks to combine these specialized systems, allowing AI to make connections between different types of data and unlock new capabilities. Early multimodal systems often relied on separate models for processing different modes, with another layer of technology to integrate the results. Today's more advanced architectures are designed from the ground up to process multiple modalities simultaneously, creating more efficient and effective systems. This evolution has accelerated rapidly in recent years, with the introduction of foundation models that can be fine-tuned for specific applications while maintaining their multimodal capabilities.
The Core Components of Modern Multimodal Systems
Modern multimodal AI systems comprise several key components working in concert to deliver seamless experiences. At their foundation are large language models (LLMs) that provide a common framework for understanding and generating human language. These models serve as the backbone for interpreting user intent and coordinating responses across different modalities. Visual perception modules enable the system to process images and video, identifying objects, scenes, actions, and even emotional expressions. Voice and audio processing components handle speech recognition, voice biometrics, and environmental sound analysis, translating audio inputs into structured data the system can understand. Integration layers manage the complex task of aligning information across these different modalities, ensuring that the system understands the relationships between what it sees and what it hears. Finally, response generation modules determine the most appropriate way to communicate back to the user, whether through text, synthesized speech, visual displays, or some combination of these modes. The sophistication of these components and how well they work together determines the overall capability and naturalness of the multimodal AI system.
Transformative Applications of Multimodal AI in 2025
Enhanced Customer Service Experiences
Customer service has been revolutionized by multimodal AI implementations that allow for more comprehensive problem resolution. Modern support systems can simultaneously analyze a customer's verbal description of a problem while examining photos or videos they share, leading to faster and more accurate diagnoses of issues. For example, in the telecommunications industry, customers can show their router setup via video while explaining the connectivity problems they're experiencing, and the AI can identify misconfigurations immediately. Retail companies have implemented virtual shopping assistants that can process photos of products customers are interested in, analyze their verbal preferences, and recommend alternatives while displaying them visually in real-time. In healthcare, patient support systems can interpret symptoms described verbally while analyzing images of physical symptoms, providing more accurate preliminary assessments before connecting patients with healthcare providers. These implementations have significantly reduced resolution times and increased customer satisfaction scores across industries, with many companies reporting 40-60% improvements in first-contact resolution rates.
Accessibility and Inclusive Design
Multimodal AI has become a powerful force for improving accessibility and inclusive design across digital platforms. Systems that can translate between different modes of communication have opened up digital experiences to users with various disabilities. For users with visual impairments, AI systems can convert visual content into detailed audio descriptions or tactile feedback, while simultaneously processing voice commands to navigate interfaces. Deaf and hard-of-hearing users benefit from real-time transcription and translation of audio content into text or sign language avatars, with the ability to respond through text or gesture recognition. People with motor impairments can use voice commands or eye tracking as alternative input methods, with the AI adapting its response mode based on the user's preferences and needs. These multimodal accessibility features are increasingly being built into mainstream products rather than offered as specialized solutions, reflecting a growing commitment to universal design principles. According to recent studies, organizations implementing multimodal accessibility solutions have seen up to 30% increases in user engagement from previously underserved populations.
Immersive Learning and Training
Education and corporate training have been transformed by multimodal AI systems that create more engaging and effective learning experiences. Modern e-learning platforms leverage multimodal AI to adapt content presentation based on individual learning styles and preferences. Visual learners receive more diagrams, videos, and interactive simulations, while auditory learners might get the same concepts presented through spoken explanations and discussions. Complex concepts can be explained through multiple complementary channels simultaneously—verbal explanations paired with animated visualizations that respond in real-time to student questions. In corporate settings, training simulations use multimodal AI to create realistic scenarios where employees can practice skills through natural conversation and interaction, receiving immediate multimodal feedback on their performance. Medical training programs use these systems to simulate patient interactions, with AI playing the role of patients who can display visual symptoms, describe their feelings verbally, and react appropriately to the trainee's questions and examinations. Educational institutions implementing multimodal learning systems have reported 25-40% improvements in knowledge retention and significantly higher student engagement scores.
Advanced Healthcare Diagnostics
Healthcare has embraced multimodal AI to enhance diagnostic accuracy and improve patient outcomes. Modern diagnostic systems combine analysis of medical images like X-rays, MRIs, and CT scans with patient verbal descriptions of symptoms, medical history data, and even real-time vital signs to form comprehensive assessments. Dermatology applications allow patients to upload photos of skin conditions while describing their symptoms and medical history, with the AI providing preliminary assessments that help dermatologists prioritize urgent cases. Mental health applications analyze combinations of speech patterns, facial expressions, and written communications to identify potential signs of conditions like depression or anxiety, supporting earlier intervention. Remote monitoring systems use multimodal inputs to track patient recovery at home, processing visual cues from video check-ins alongside reported symptoms and data from connected medical devices. These integrated approaches have significantly improved diagnostic accuracy, with some studies showing 15-30% reductions in misdiagnosis rates when multimodal systems support healthcare providers. The ability to process multiple streams of complementary information mimics the way experienced clinicians naturally integrate different types of observations.
Implementation Strategies and Best Practices
Designing Human-Centered Multimodal Experiences
Creating effective multimodal AI experiences requires a deep understanding of human cognitive processes and communication preferences. Successful implementations start with comprehensive user research to understand which modalities are most appropriate for different types of interactions and user contexts. Designers must consider cognitive load carefully, ensuring that multiple modes enhance rather than overwhelm the user experience. For instance, presenting compatible information across modes (like highlighting visual elements that correspond to what's being described verbally) can reduce cognitive load, while contradictory information across modes can create confusion. Providing users with control over which modalities are active is essential, allowing them to choose the combination that works best for their current situation, preferences, and accessibility needs. Organizations should implement consistent interaction patterns across modalities to create intuitive experiences, while also leveraging the unique strengths of each mode. The most successful multimodal implementations create experiences that feel natural and effortless, where the technology seems to anticipate user needs and adapt accordingly. This human-centered approach requires ongoing testing and refinement based on user feedback and behavior analysis.
Technical Infrastructure and Integration Challenges
Building robust multimodal AI systems presents significant technical challenges that organizations must address. These systems require substantially more computational resources than single-mode AI, necessitating scalable cloud infrastructure or powerful edge computing solutions depending on the application requirements. Latency management becomes critical when synchronizing multiple modes in real-time, as even slight delays between visual and audio responses can disrupt the natural feel of interactions. Data pipeline architecture must support the efficient ingestion and processing of diverse data types, from high-resolution images to audio streams and text inputs. Legacy system integration often presents hurdles, as many existing enterprise systems were not designed with multimodal interactions in mind. Organizations successful in implementing multimodal AI typically adopt microservices architectures that allow different modality processing components to evolve independently while maintaining smooth integration. Privacy and security considerations become more complex with multimodal systems, as they often process more sensitive personal data across multiple channels simultaneously. Implementing proper data encryption, anonymization techniques, and strict access controls is essential to protect user information while complying with evolving regulatory requirements.
Ethical Considerations and Responsible Deployment
As multimodal AI systems become more sophisticated and prevalent, ethical considerations have moved to the forefront of implementation strategies. These systems raise important questions about privacy, consent, and data ownership that organizations must address proactively. Transparency about when and how multimodal data is being collected and processed is essential for maintaining user trust, with clear opt-in mechanisms for sensitive modes like video or biometric data. Bias mitigation presents unique challenges in multimodal systems, as biases can manifest differently across modalities and even amplify when multiple modes interact. Organizations must implement rigorous testing frameworks to identify and address biases in how the system responds to different user groups across all supported modalities. Accessibility must be a core design principle rather than an afterthought, ensuring that users with disabilities can benefit from multimodal systems rather than being further excluded by them. Responsible deployment also includes establishing clear accountability structures for multimodal AI decisions, especially in high-stakes domains like healthcare or financial services. Leading organizations are establishing ethics committees and governance frameworks specifically for multimodal AI, recognizing that these systems present novel ethical challenges that require dedicated attention and oversight.
Statistics & Future Outlook
Measuring the Impact of Multimodal AI Implementation
Organizations implementing multimodal AI solutions have reported significant improvements across key performance indicators. According to a 2025 industry survey of over 500 enterprises, companies using multimodal AI in customer service have seen average customer satisfaction scores increase by 32% compared to traditional single-channel approaches. Efficiency metrics are equally impressive, with average handle times decreasing by 24% as agents leverage multimodal systems to resolve issues more quickly. Employee productivity has seen substantial gains, with knowledge workers reporting an average 27% reduction in time spent on routine tasks when using multimodal AI assistants. These productivity improvements translate directly to bottom-line benefits, with organizations reporting an average 22% decrease in operational costs for processes enhanced by multimodal AI. The impact on accessibility has been particularly notable, with websites and applications implementing multimodal interfaces seeing an average 38% increase in engagement from users with disabilities. Perhaps most significantly, businesses using multimodal AI for product development have reported bringing new offerings to market 31% faster than competitors relying on traditional approaches. These quantifiable benefits help explain why multimodal AI has moved from experimental technology to mainstream implementation so quickly.
Future Directions and Emerging Capabilities
The future of multimodal AI promises even more sophisticated capabilities as research continues to advance rapidly. Emerging emotional intelligence capabilities will allow systems to better understand and respond to human emotional states by analyzing subtle cues across facial expressions, voice intonation, word choice, and even physiological indicators. Hybrid reality experiences that seamlessly blend physical and digital environments are being enhanced by multimodal AI that can understand and respond to the physical world while overlaying contextually relevant digital information. The integration of tactile feedback systems represents the next frontier in multimodality, creating experiences where users can "feel" virtual objects or receive haptic guidance for physical tasks. Cross-cultural and multilingual capabilities are advancing quickly, with systems that can translate not just words but culturally-specific gestures, expressions, and contexts across different languages and cultural frameworks. Personalization is becoming increasingly sophisticated, with systems that learn individual preferences for different modalities in different contexts and adapt accordingly. Perhaps most significantly, autonomous multimodal agents that can persist and operate independently across digital environments are beginning to emerge, promising to transform how we think about digital assistance and representation. These developments suggest that we're still in the early stages of the multimodal revolution, with many more transformative capabilities on the horizon.
Case Studies of Successful Implementations
Retail Revolution: MultiSense Shopping Platform
One of the most successful implementations of multimodal AI comes from the retail sector, where the MultiSense platform has transformed the shopping experience for millions of customers. This innovative system allows shoppers to interact through any combination of voice, text, image, and gesture, creating a seamless experience across online and in-store environments. Customers can take photos of products they see in the real world and ask detailed questions about them verbally, receiving comprehensive information through their preferred output modality. The platform's visual search capabilities allow shoppers to find products based on images even when they don't know the correct terminology, while its voice interface supports natural conversation about complex product comparisons. In physical stores, interactive displays respond to both voice commands and gestures, allowing customers to explore product catalogs intuitively while receiving personalized recommendations based on their purchase history and stated preferences. Since implementing the MultiSense platform, the retail group has reported a 43% increase in conversion rates and a 28% increase in average order value. Perhaps most impressively, customer loyalty metrics have improved dramatically, with a 37% increase in repeat purchase rates attributed directly to the improved shopping experience.
Healthcare Transformation: Multimodal Diagnostic Assistant
In the healthcare sector, the implementation of a Multimodal Diagnostic Assistant has demonstrated the life-saving potential of this technology. This sophisticated system supports healthcare providers by simultaneously analyzing patient electronic health records, diagnostic images, lab results, and transcribed patient interviews to identify patterns that might be missed by even experienced clinicians. During patient consultations, the system can process real-time audio of the patient describing symptoms while simultaneously analyzing visual cues from video feeds, comparing findings to the patient's medical history, and suggesting potential diagnoses or additional tests that should be considered. For radiologists, the assistant can highlight areas of concern in medical images while providing verbal explanations of its reasoning, significantly accelerating the diagnostic process. The system continually learns from feedback provided by healthcare professionals, improving its accuracy across all modalities over time. In a large-scale validation study involving 12 major hospitals, the Multimodal Diagnostic Assistant helped identify critical conditions that were initially missed in 14% of complex cases, potentially saving numerous lives. Equally important, the system reduced unnecessary testing by 22%, leading to significant cost savings while improving patient experience by reducing wait times and anxiety.
Conclusion
The integration of multimodal AI represents one of the most significant technological shifts in how humans interact with machines since the introduction of the graphical user interface. By bridging the gap between how we naturally communicate with each other and how we interact with technology, multimodal systems are creating more intuitive, accessible, and powerful experiences across countless applications. The statistics and case studies highlighted in this article demonstrate that multimodal AI is delivering measurable benefits for businesses and users alike, from improved customer experiences to life-saving healthcare applications. As we look to the future, the continued evolution of this technology promises even more transformative capabilities, from enhanced emotional intelligence to seamless hybrid reality experiences. For organizations that have not yet begun exploring multimodal AI integration, now is the time to develop a strategic approach. Those who successfully implement these technologies will gain significant competitive advantages in customer engagement, operational efficiency, and innovative capabilities. The multimodal revolution is well underway, and its impact will only grow as the technology continues to mature and evolve beyond what we can imagine today.
Frequently Asked Questions
What exactly is multimodal AI and how does it differ from traditional AI systems?
Multimodal AI refers to artificial intelligence systems that can process and generate information across multiple forms of communication or "modes"—such as text, images, audio, and video—simultaneously. Unlike traditional AI systems that typically specialized in a single mode (text only or image only), multimodal AI can understand connections between different types of input and create integrated experiences that more closely resemble human cognitive processes.
What industries are seeing the biggest impact from multimodal AI implementation?
Healthcare, retail, customer service, education, and manufacturing are currently seeing the most significant impacts. These industries benefit from AI's ability to process complex information across multiple channels, whether it's analyzing medical images alongside patient histories or helping customers shop through a combination of visual and voice interactions.
What are the main technical challenges in implementing multimodal AI systems?
Key challenges include managing the substantial computational resources required, synchronizing different modalities with minimal latency, creating efficient data pipelines for diverse data types, integrating with legacy systems, and establishing robust privacy and security measures for sensitive multimodal data. Organizations also face challenges in designing intuitive user experiences that leverage multiple modalities effectively.
How does multimodal AI improve accessibility for users with disabilities?
Multimodal AI creates more accessible experiences by offering alternative ways to interact with technology. For example, visually impaired users can use voice commands and receive audio feedback, while deaf users can interact through text and visual elements. The system adapts to individual needs, translating between modalities as needed and providing multiple channels for both input and output.
What ethical concerns should organizations consider when implementing multimodal AI?
Important ethical considerations include transparency about data collection across multiple modalities, obtaining proper consent, mitigating biases that can manifest differently across modalities, ensuring equitable access to multimodal experiences, and establishing clear accountability for AI decisions. Organizations should develop comprehensive ethics frameworks specifically for multimodal AI implementation.
How can businesses measure the ROI of multimodal AI implementations?
Key metrics to track include customer satisfaction scores, efficiency improvements like reduced handling times, employee productivity gains, operational cost reductions, increased engagement from diverse user groups, and accelerated time-to-market for new products or services. Most organizations see improvements across multiple metrics simultaneously.
What skills are needed for teams implementing multimodal AI projects?
Successful implementation requires a diverse team with expertise in machine learning, UX design, accessibility, data engineering, cloud infrastructure, and domain-specific knowledge. Increasingly important are skills in multimodal interaction design, which requires understanding how different modes can complement each other effectively.
How does multimodal AI handle different languages and cultures?
Advanced multimodal AI systems can process and generate content in multiple languages, translating not just words but cultural contexts, gestures, and expressions. This capability is increasingly important for global organizations seeking to provide consistent experiences across different regions and cultural contexts.
What infrastructure is typically needed to support multimodal AI systems?
Most implementations require robust cloud computing resources to handle the intensive processing demands, though some applications leverage edge computing for latency-sensitive features. Organizations typically need scalable data storage solutions, high-bandwidth networks to handle multiple data streams, and specialized hardware accelerators for real-time processing of modalities like video and audio.
What does the future of multimodal AI look like beyond 2025?
The future will likely bring more seamless integration of physical and digital experiences through advanced multimodal systems, enhanced emotional intelligence capabilities, more sophisticated personalization, greater autonomous functionality, and integration of emerging modalities like tactile feedback and spatial computing. We can expect increasingly natural interactions that make the technology itself feel invisible.
Additional Resources
Multimodal AI Integration Guide - Our comprehensive guide to implementing multimodal AI in business environments.
Understanding Context Windows in Large Language Models - Learn how context windows enable large language models to manage multimodal inputs effectively.
Voice-Enabled AI Interactions - Explore the audio component of multimodal systems in depth.
ChatGPT's Impact on Virtual Reality and Augmented Reality - Discover how multimodal AI is transforming immersive technologies.
Multimodal AI Models: Features and Applications - A detailed exploration of current multimodal AI architectures and use cases.