Multimodal AI Models: Features and Applications
Explore a detailed comparison of leading multimodal AI models, highlighting their modalities, key features, and practical applications. Discover how these models are transforming industries through advanced data integration and analysis.
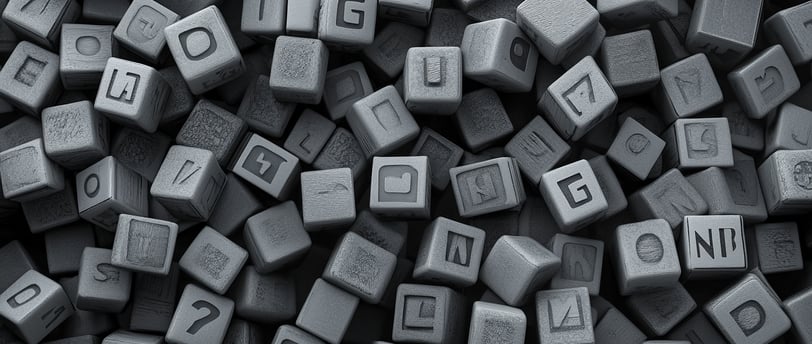

Multimodal AI is revolutionizing how businesses operate by integrating various data types to provide comprehensive insights. This article explores the concept of multimodal AI, its applications across different industries, and the potential benefits it offers. We will delve into real-world use cases, the technical aspects of implementing multimodal AI, and the future trends shaping this technology.
What is Multimodal AI?
Multimodal AI refers to artificial intelligence systems capable of processing and integrating information from multiple modalities, such as text, images, audio, and video. Unlike traditional AI models that focus on a single data type, multimodal AI combines these inputs to generate more accurate and contextually rich outputs. This capability mimics the human brain's ability to process multiple sensory inputs simultaneously, making multimodal AI a powerful tool for various applications12.
Applications of Multimodal AI
Multimodal AI is transforming various industries by enabling more intuitive and context-aware interactions. Here are some key applications:
Customer Service: Multimodal AI helps customer service teams better understand a customer's feelings and intentions by analyzing their voice tone, facial expressions, and written words. This allows for more personalized and effective interactions, improving customer satisfaction1.
Document Transcription/Extraction: Generative multimodal AI automates the conversion of various document types—like scanned images, PDFs, and handwritten notes—into structured, usable data. This technology combines advanced optical character recognition (OCR) with natural language processing (NLP) to transcribe text and also understand its context, making the data more useful1.
Retail: In retail, multimodal AI is used to offer more personalized shopping experiences. It looks at a customer’s previous purchases, browsing history, and social media activity to suggest products that they are more likely to buy1.
Security: Security systems use multimodal AI to analyze both video and audio data to better detect threats. It helps identify unusual behavior and stressed voices, enabling quicker and more accurate responses to security incidents1.
Manufacturing: In manufacturing, multimodal AI monitors equipment using visual and sensor data. This helps predict when machines might break down, allowing for timely maintenance that keeps production lines running smoothly1.
How Multimodal AI Works
Multimodal AI systems typically consist of three main components:
Input Module: This module is made up of several unimodal neural networks. Each network handles a different type of data, collectively making up the input module1.
Fusion Module: After the input module collects the data, the fusion module takes over. This module processes the information coming from each data type1.
Output Module: This final component delivers the results based on the integrated data1.
Trends in Multimodal AI
Multimodal AI is a rapidly evolving field, with several key trends shaping its development and application:
Unified Models: Models like OpenAI’s GPT-4 V(ision) and Google’s Gemini are designed to handle text, images, and other data types within a single architecture. These models can understand and generate multimodal content seamlessly2.
Enhanced Cross-Modal Interaction: Advanced attention mechanisms and transformers are being used to better align and fuse data from different formats, leading to more coherent and contextually accurate outputs2.
Real-Time Multimodal Processing: Applications in autonomous driving and augmented reality require AI to process and integrate data from various sensors in real-time to make instantaneous decisions2.
Multimodal Data Augmentation: Researchers are generating synthetic data that combines various modalities to augment training datasets and improve model performance2.
Open Source and Collaboration: Initiatives like Hugging Face and Google AI are providing open-source AI tools, fostering a collaborative environment for researchers and developers to advance the field2.
Popular Multimodal AI Models
Here are some of the most notable multimodal AI models:
Applications of Multimodal AI
Customer Service
In customer service, multimodal AI enhances interactions by analyzing voice tones, facial expressions, and written words. This comprehensive analysis allows for more personalized and effective customer support, improving satisfaction and loyalty. For instance, AI-powered chatbots can understand and respond to customer queries more accurately by integrating visual and contextual information34.
Document Transcription/Extraction
Generative multimodal AI automates the conversion of various document types, such as scanned images, PDFs, and handwritten notes, into structured, usable data. This technology combines advanced optical character recognition (OCR) with natural language processing (NLP) to transcribe text and understand its context, making the data more valuable and actionable56.
Retail
In the retail sector, multimodal AI offers more personalized shopping experiences by analyzing a customer’s previous purchases, browsing history, and social media activity. This data integration helps retailers suggest products that customers are more likely to buy, enhancing the overall shopping experience54.
Security
Security systems utilize multimodal AI to analyze both video and audio data for threat detection. By identifying unusual behavior and stressed voices, these systems enable quicker and more accurate responses to security incidents, ensuring better protection and safety4.
Manufacturing
In manufacturing, multimodal AI monitors equipment using visual and sensor data. This helps predict equipment failures, allowing for timely maintenance and preventing production downtime. By integrating various data inputs, manufacturers can keep production lines running smoothly and efficiently64.
How Multimodal AI Works
Multimodal AI systems typically consist of three main components:
Input Module: This module comprises several unimodal neural networks, each handling a different type of data, such as text, images, or audio. These networks collectively make up the input module, processing and preparing the data for further analysis57.
Fusion Module: After the input module collects the data, the fusion module processes the information from each data type. This module integrates the data, ensuring that the system can analyze and understand the relationships between different data inputs57.
Output Module: This final component delivers the results based on the integrated data. The output module generates comprehensive insights and predictions, leveraging the combined power of multiple data modalities57.
Trends in Multimodal AI
Several key trends are shaping the development and application of multimodal AI:
Unified Models: Models like OpenAI’s GPT-4 V(ision) and Google’s Gemini are designed to handle text, images, and other data types within a single architecture. These unified models can understand and generate multimodal content seamlessly, providing a more coherent and contextually accurate output57.
Enhanced Cross-Modal Interaction: Advanced attention mechanisms and transformers are being used to better align and fuse data from different formats. This enhancement leads to more coherent and contextually accurate outputs, making multimodal AI more effective in various applications57.
Real-Time Multimodal Processing: Applications in autonomous driving and augmented reality require AI to process and integrate data from various sensors in real time. This capability allows for instantaneous decision-making, crucial for safety and efficiency in these fields57.
Multimodal Data Augmentation: Researchers are generating synthetic data that combines various modalities to augment training datasets. This augmentation improves model performance by providing more diverse and comprehensive training data57.
Open Source and Collaboration: Initiatives like Hugging Face and Google AI are providing open-source AI tools, fostering a collaborative environment for researchers and developers to advance the field of multimodal AI57.
Implementing Multimodal AI in Business
Implementing multimodal AI in a business setting requires careful planning and execution. Here are the key steps to incorporate multimodal AI into your business operations:
Understand Your Data: Begin by understanding the types of data your business generates and processes. This comprehension will guide you in choosing the right combination of AI models to analyze your data effectively12.
Choose the Right Platform: Determine the best multimodal AI platform or solution that suits your business needs. Consider factors like scalability, integration capabilities, and support for different data modalities12.
Develop a Roadmap: Create a roadmap for implementation that includes resource allocation, timelines, and expected milestones. This roadmap will help you stay on track and ensure that the implementation process is smooth and efficient12.
Monitor and Adjust: Continuously monitor and adjust the system to optimize its performance and align it with your business needs. Multimodal AI implementation is an ongoing process that requires regular updates and improvements12.
Technical Requirements and Data Integration
Implementing multimodal AI requires robust computational capabilities and large amounts of data storage. Additionally, it necessitates proficient data scientists and engineers experienced in AI development, capable of managing the complex interplay of different AI modalities12.
Data integration plays a crucial role in the success of multimodal AI systems. Different data types need to be seamlessly integrated to generate richer and more comprehensive insights. Proper data integration can help businesses unlock the full potential of multimodal AI, enabling them to extract more valuable insights from their data and make better-informed decisions12.
Overcoming Barriers to Implementation
While adopting multimodal AI can pose challenges such as steep learning curves, data security concerns, and high implementation costs, these can be overcome with strategic planning and the right resources. Training your team, partnering with experienced AI companies, and ensuring strict data governance can help mitigate these barriers and ensure a successful implementation12.
Specific Use Cases for Multimodal AI
Supply Chain Management
Multimodal AI can significantly improve operational efficiency in supply chain management. By integrating data sets and data streams from sensors, GPS, and inventory systems, businesses can gain a comprehensive and real-time understanding of their supply chain dynamics. This integration allows for informed decision-making, streamlined processes, and swift responses to changing demands or disruptions2.
Sports Analytics
In sports analytics, multimodal AI provides new ways to dissect and comprehend sports performance. By integrating video footage, player tracking systems, and performance metrics, teams can gain profound insights into athletes' capabilities and strategic gameplay. This multimodal approach offers a comprehensive understanding of the game, enabling teams to make strategic decisions grounded in data2.
The Future of Multimodal AI
As technology continues to evolve, multimodal AI is poised to transform various industries and create tangible business value. In the future, we can expect to see more sophisticated multimodal AI systems capable of processing and integrating a broader spectrum of data types. These advancements will make multimodal AI more intuitive, accurate, and efficient, revolutionizing business processes, customer experiences, and decision-making frameworks12.
Conclusion
Multimodal AI represents a significant leap forward in artificial intelligence, offering businesses the ability to integrate and analyze multiple data modalities for more accurate and contextually rich insights. By understanding the applications, technical requirements, and future trends of multimodal AI, businesses can harness its power to drive growth, innovation, and competitiveness. As multimodal AI continues to evolve, it will undoubtedly play a crucial role in shaping the future of various industries, from healthcare to retail and beyond.
FAQ Section
What is multimodal AI?
Multimodal AI refers to artificial intelligence systems that can process and integrate information from multiple modalities, such as text, images, audio, and video, to generate more accurate and contextually rich outputs.
How does multimodal AI differ from traditional AI?
Unlike traditional AI models that focus on a single data type, multimodal AI combines multiple data inputs to provide a more comprehensive understanding and generate more accurate insights.
What are the key components of a multimodal AI system?
The key components of a multimodal AI system include the input module, fusion module, and output module. The input module processes different data types, the fusion module integrates this data, and the output module delivers the results.
What are some real-world applications of multimodal AI?
Real-world applications of multimodal AI include customer service, document transcription, retail, security, and manufacturing. These applications leverage multimodal AI's ability to integrate and analyze multiple data types for more accurate and contextually rich insights.
What are the benefits of implementing multimodal AI in business?
Implementing multimodal AI in business can drive innovation, decision-making, and revenue growth. It can help businesses anticipate customer needs, improve product design, and enhance marketing strategies, ultimately leading to increased competitiveness.
What are the technical requirements for implementing multimodal AI?
Implementing multimodal AI requires robust computational capabilities, large amounts of data storage, and proficient data scientists and engineers experienced in AI development. Proper data integration is also crucial for the success of multimodal AI systems.
What are some challenges in implementing multimodal AI?
Challenges in implementing multimodal AI include steep learning curves, data security concerns, and high implementation costs. These challenges can be overcome with strategic planning, training, and partnerships with experienced AI companies.
How can businesses prepare for the future of multimodal AI?
Businesses can prepare for the future of multimodal AI by staying informed about the latest trends, investing in AI training for their teams, building a data-driven culture, and continuously adapting their AI strategies to exploit the evolving capabilities of multimodal AI.
What industries can benefit from multimodal AI?
Multimodal AI can benefit various industries, including healthcare, retail, finance, manufacturing, and sports analytics. Its ability to integrate and analyze multiple data types makes it a valuable tool for enhancing business processes and customer experiences.
What are some emerging trends in multimodal AI?
Emerging trends in multimodal AI include unified models, enhanced cross-modal interaction, real-time multimodal processing, multimodal data augmentation, and open-source collaboration. These trends are shaping the development and application of multimodal AI across different industries.