SearchGPT vs. Traditional Search: The Future of Information Discovery
Discover how SearchGPT is revolutionizing information retrieval compared to traditional search engines. Explore the key differences, benefits, limitations, and future trajectory of search technology in this comprehensive analysis.
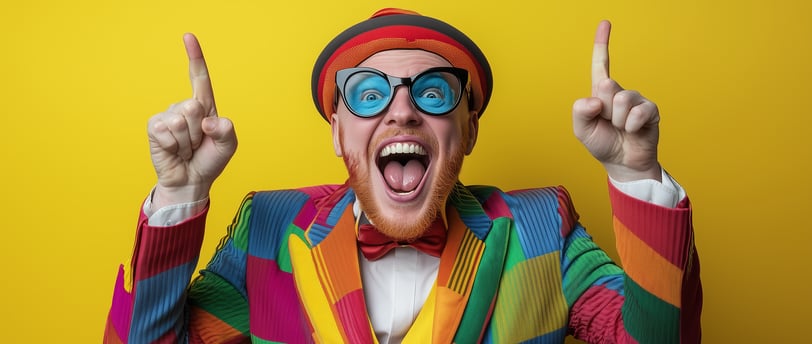
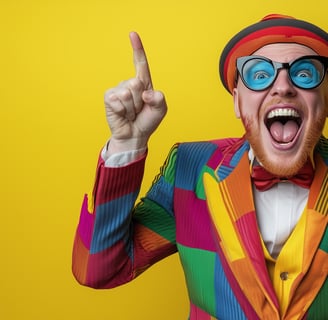
Remember the days when finding information online meant sifting through pages of barely relevant search results? For decades, traditional search engines have been our gateway to the digital world's vast knowledge repository. Yet, despite their sophistication, these systems still require us to think like computers – choosing precise keywords and modifying queries until we find what we need. Enter SearchGPT, a revolutionary approach to information discovery that promises to understand not just our words, but our intentions. The emergence of large language models has fundamentally changed how we interact with information, bringing us closer to the seamless, intuitive experience we've always wanted. As we stand at this technological crossroads, one question becomes increasingly relevant: Will AI-powered search solutions like SearchGPT replace traditional search engines, or will they evolve together to create something entirely new? This article examines the strengths, limitations, and future potential of both approaches as we navigate the rapidly changing landscape of information discovery.
The Evolution of Search Technology
The journey of search technology began in the early 1990s with simple directory-based systems that relied on manual categorization of websites. These primitive tools quickly gave way to more sophisticated keyword-based search engines like AltaVista, which introduced automated web crawling and indexing at scale. The true revolution came in 1998 when Google introduced PageRank, transforming search by evaluating the quality and relevance of pages based on their link structures. This advancement marked the beginning of the modern search era, where complex algorithms determine which results deserve the top spots in response to our queries. Throughout the 2000s and 2010s, search engines continued to evolve, incorporating semantic understanding, voice recognition, and mobile optimization to better serve user needs. Each iteration brought incremental improvements, yet the fundamental model remained largely unchanged: users input keywords, and algorithms retrieve matching documents.
The integration of machine learning into search marked another significant milestone, allowing systems to learn from user behavior and improve results over time. Search engines began to understand synonyms, recognize entities, and even predict what users might be looking for based on their search history. These improvements, while substantial, still operated within the confines of the keyword-matching paradigm that defined traditional search. The true paradigm shift arrived with the development of large language models like GPT, which approach information retrieval from an entirely different angle. Rather than matching keywords to indexed documents, these models leverage deep neural networks trained on vast corpora of text to generate responses directly. This fundamental difference in approach sets the stage for our comparison between traditional search engines and SearchGPT-like systems.
The acceleration of AI capabilities in recent years has been nothing short of remarkable, with models growing from millions to billions of parameters in just a few years. This exponential growth in model size and capability has enabled systems like SearchGPT to achieve levels of language understanding that would have seemed impossible just a decade ago. Today's models can comprehend context, recognize nuance, and generate human-like responses that address the intent behind a query rather than simply matching keywords. As we examine this evolution, it becomes clear that we're witnessing not just an improvement in search technology, but potentially a complete reimagining of how humans interact with information. With this historical context in mind, we can better understand both the strengths and limitations of traditional search engines and their AI-powered counterparts.
How Traditional Search Engines Work
Traditional search engines operate on a three-step process that has remained fundamentally unchanged for decades: crawling, indexing, and ranking. The process begins with web crawlers – automated bots that systematically browse the internet, following links from page to page and collecting information about each document they encounter. These digital explorers continuously traverse the web, discovering new content and revisiting existing pages to check for updates. The scale of this operation is staggering, with Google alone processing billions of pages daily to maintain its understanding of the web. Once content is discovered, it enters the indexing phase, where search engines analyze and categorize each page based on its content, meta information, and structural elements. This massive index serves as the foundation for all search operations, allowing for rapid retrieval when users submit queries.
The ranking phase is where the real magic happens, as search engines employ sophisticated algorithms to determine which results best match a user's query. Modern ranking systems consider hundreds of factors, including keyword relevance, page authority, user location, search history, and device type. The goal is to present the most useful information at the top of the results page, saving users from having to dig through irrelevant content. Major search engines like Google regularly update their algorithms to improve result quality and combat manipulation techniques. These updates, with names like Panda, Penguin, and BERT, reflect the ongoing arms race between search providers striving for relevance and content creators attempting to game the system. Despite their sophistication, traditional search engines remain fundamentally reactive – they wait for users to input specific queries before retrieving pre-indexed information.
The business model behind traditional search has also shaped how these systems work and what they prioritize. Search engines generate revenue primarily through advertising, creating an inherent tension between serving user needs and satisfying commercial interests. This economic reality influences everything from page design to the types of results that receive prominence. While search engines strive to provide value to users, they must simultaneously generate revenue to sustain their operations. This balancing act becomes particularly evident in competitive commercial queries, where paid results often dominate the most visible positions. The advertising-based model has enabled search engines to offer their services for free, contributing to their near-universal adoption, but it has also introduced biases and limitations that affect the user experience.
The technical architecture of traditional search requires massive infrastructure investments, with data centers around the world storing and processing vast indexes of the web. Google's index alone is estimated to be hundreds of petabytes in size, requiring enormous computational resources to maintain and query. This centralized approach allows for efficient scaling but also creates bottlenecks and vulnerabilities. The need to constantly crawl, index, and rank the web consumes significant bandwidth and energy, raising questions about the environmental sustainability of this model as the internet continues to grow. Despite these challenges, traditional search engines have proven remarkably adaptable, continuously evolving to accommodate new content types, user behaviors, and technological capabilities while maintaining their fundamental approach to information retrieval.
Understanding SearchGPT and AI-Powered Search
SearchGPT represents a fundamentally different approach to information discovery, leveraging the power of large language models (LLMs) to generate responses rather than simply retrieve pre-indexed content. At its core, SearchGPT is built on transformer-based neural networks trained on vast amounts of text data, allowing it to develop a nuanced understanding of language and knowledge. Unlike traditional search engines that match keywords to documents, SearchGPT processes queries as conversational inputs and generates natural language responses that directly address user questions. This generative approach means that instead of providing a list of links that might contain the answer, SearchGPT attempts to synthesize information and provide complete answers within the interface itself. The model's training enables it to understand context, recognize entities, follow complex instructions, and even reason through multi-step problems in ways that keyword matching cannot achieve.
The technical architecture behind SearchGPT differs significantly from traditional search infrastructure. While conventional search engines rely on massive indexes of web pages, SearchGPT encodes information within its neural network parameters during training. This "parametric knowledge" allows the model to access and utilize information without explicitly referencing a separate database for every query. Of course, modern implementations often combine this parametric knowledge with retrieval-augmented generation (RAG) techniques that pull in fresh or specialized information from external sources when needed. This hybrid approach helps address one of the key limitations of pure LLMs – their inability to access real-time information or content created after their training cutoff date. By integrating retrieval mechanisms, SearchGPT-type systems can provide more current and verifiable information while still leveraging the language understanding capabilities of the underlying model.
The user experience with SearchGPT differs dramatically from traditional search. Instead of scanning through a list of potential sources, users engage in a conversational interface that feels more like asking a knowledgeable assistant than querying a database. This natural interaction reduces the cognitive load associated with formulating precise search queries and evaluating multiple results. Users can ask follow-up questions, request clarification, or pivot to related topics without starting a new search. The system maintains context throughout the conversation, creating a continuity that traditional search engines struggle to match. This conversational capability makes SearchGPT particularly effective for exploratory searches where users may not have a clear idea of what they're looking for or how to articulate their needs using keywords.
The development path of SearchGPT and similar AI search tools reveals an interesting convergence of multiple AI technologies. These systems combine natural language processing, knowledge representation, retrieval mechanisms, and reasoning capabilities to create a more holistic approach to information access. As the underlying models continue to improve in size, training, and architecture, their ability to serve as information gateways grows accordingly. Recent advancements have focused on reducing hallucinations (the generation of plausible but incorrect information), improving factual accuracy, and enhancing the system's ability to cite sources properly. These improvements address some of the key concerns about using generative AI for search functions while maintaining the conversational, intuitive interface that makes these systems appealing to users seeking more natural ways to discover information.
Comparative Analysis: SearchGPT vs. Traditional Search
When comparing SearchGPT to traditional search engines, one of the most striking differences lies in query interpretation. Traditional search engines treat queries as collections of keywords to be matched against an index, requiring users to think carefully about their word choice and syntax. In contrast, SearchGPT interprets natural language questions, understanding implicit meaning and contextual nuances that keyword matching might miss. This difference becomes particularly apparent with complex or ambiguous queries, where traditional search often returns a broad range of results in hopes that something will be relevant. SearchGPT, with its language understanding capabilities, can parse intent more accurately and provide targeted responses even when queries are vaguely worded. This ability to understand rather than just match significantly reduces the need for query refinement, saving users time and frustration when seeking specific information.
The format of results represents another fundamental difference between these approaches. Traditional search provides a list of links, leaving users to click through multiple sources to find and synthesize the information they need. SearchGPT generates comprehensive answers directly within the interface, often eliminating the need to visit multiple websites. This direct answer approach can be incredibly convenient for straightforward informational queries, though it raises questions about source attribution and verification. Traditional search excels at providing diverse viewpoints and allowing users to evaluate sources directly, while SearchGPT offers a more streamlined experience at the potential cost of transparency. The choice between these approaches often depends on the user's specific needs – quick answers versus in-depth research with verifiable sources.
Performance metrics reveal different strengths for each system. Traditional search engines typically deliver results in milliseconds, leveraging their optimized indexes for nearly instantaneous response times. SearchGPT and other LLM-based systems generally require more processing time, generating responses token by token rather than retrieving pre-indexed content. However, when considering total task completion time – including the time spent visiting multiple sites and synthesizing information – SearchGPT might actually save users time for certain types of queries. Resource efficiency also differs dramatically between these approaches. Traditional search uses massive but relatively static infrastructure, while SearchGPT demands significant computational resources for each query as it generates unique responses. This difference in resource requirements has implications for scaling, cost, and environmental impact that organizations must consider when choosing between approaches.
The information landscape itself influences the effectiveness of each approach. Traditional search excels at finding recent or constantly changing information, as crawlers continuously update their indexes with new content. SearchGPT's parametric knowledge is limited by its training cutoff date, making it less effective for time-sensitive queries without additional retrieval mechanisms. Conversely, SearchGPT often outperforms traditional search for evergreen topics, historical information, and conceptual questions where its training data provides comprehensive coverage. The breadth versus depth tradeoff becomes apparent when comparing these systems – traditional search offers broad coverage of the constantly changing web, while SearchGPT provides deeper understanding of the content within its knowledge cutoff. This complementary nature suggests that the optimal approach might involve leveraging both technologies rather than choosing between them.
Advantages of SearchGPT
The natural language understanding capabilities of SearchGPT represent one of its most significant advantages over traditional search. Users can phrase queries conversationally, ask questions as they would to another person, and receive responses that address the intent behind their words rather than just matching keywords. This intuitive interaction reduces the cognitive burden of formulating precise search terms and eliminates the need to learn specialized search operators. For users who struggle with traditional search – including children, elderly individuals, or those with limited technical literacy – this conversational approach opens new possibilities for information access. The system's ability to understand synonyms, recognize entities, and interpret ambiguous language means that users no longer need to guess which specific terms will yield the best results. This natural interface aligns with our innate communication preferences, making technology adapt to humans rather than forcing humans to adapt to technology.
The contextual awareness of SearchGPT creates a more coherent search experience across multiple queries. Unlike traditional search, where each query exists in isolation, SearchGPT maintains conversation history and understands references to previous questions. This contextual understanding allows for natural follow-up questions, clarifications, and topic exploration without repeatedly establishing context. A user might ask about climate change, then follow with questions about specific impacts or solutions without needing to specify the topic again. This conversational flow mirrors human dialogue more closely than the disjointed query-by-query approach of traditional search. For complex research tasks that involve multiple related questions, this contextual awareness significantly reduces friction and cognitive load, allowing users to maintain their train of thought throughout the information discovery process.
One of SearchGPT's most powerful features is its ability to synthesize information from multiple sources into coherent, comprehensive responses. Rather than presenting separate links that users must evaluate and integrate themselves, SearchGPT can analyze relationships between concepts, identify patterns, and present unified explanations. This synthesis capability proves particularly valuable for questions that span multiple domains or require connecting disparate pieces of information. For example, a query about the economic and environmental impacts of renewable energy might draw from economics, environmental science, policy research, and technological analyses – connections that users would otherwise need to make manually. By performing this integration automatically, SearchGPT reduces the mental effort required to develop a complete understanding of complex topics.
The ability to handle nuanced reasoning and explanation sets SearchGPT apart from keyword-based search systems. Traditional search excels at finding documents that contain specific terms but struggles with questions that require inference, analysis, or explanation. SearchGPT can walk through logical steps, explain causal relationships, and provide reasoning for its responses in a way that mimics human explanatory capabilities. This makes it particularly effective for educational queries, conceptual questions, and situations requiring not just facts but understanding. A student asking why certain chemical reactions occur, how historical events influenced modern politics, or what factors contribute to economic phenomena will receive explanations that connect causes and effects rather than simply documents containing related keywords. This explanatory dimension transforms search from a document retrieval tool into a knowledge transfer mechanism that more closely resembles human teaching and learning processes.
Limitations and Challenges of AI Search
Despite its impressive capabilities, SearchGPT faces significant challenges related to factual accuracy and hallucinations. Large language models have a tendency to generate plausible-sounding but incorrect information when their parametric knowledge is incomplete or imprecise. Unlike traditional search engines that generally point to external sources without creating new content, SearchGPT actively synthesizes information, introducing opportunities for factual errors. These hallucinations can be particularly problematic because they often appear alongside accurate information and may be delivered with the same confidence, making errors difficult for users to detect. Recent implementations have made progress in addressing this issue through techniques like retrieval-augmented generation, which grounds responses in verified external sources, and improved training methods that reward accuracy. However, the challenge remains significant, especially for specialized domains or questions at the edge of the model's knowledge base where the system might generate seemingly authoritative but incorrect responses.
The lack of transparency presents another serious limitation for AI search systems. Traditional search engines provide links to sources, allowing users to verify information and evaluate credibility directly. SearchGPT-style systems often provide information without clear attribution, making it difficult for users to assess the reliability of responses or investigate further. While recent versions have improved source citation capabilities, the black-box nature of large language models means users cannot easily determine how the system arrived at particular conclusions or which sources influenced the response. This opacity raises concerns about accountability and makes it challenging to identify potential biases or errors in the system's outputs. For applications requiring high levels of trust – such as medical, legal, or financial information – this lack of transparency remains a significant barrier to adoption.
Data recency represents a fundamental limitation for pure LLM-based search systems. SearchGPT's knowledge is effectively frozen at its training cutoff date, making it unable to provide information about recent events or developments without additional retrieval mechanisms. Traditional search engines continuously crawl the web, updating their indexes to include new content as it appears online. This difference creates a significant advantage for conventional search when dealing with time-sensitive or rapidly evolving topics. While retrieval-augmented approaches help address this limitation by incorporating fresh information from external sources, these hybrid systems introduce additional complexity and potential points of failure. The challenge of balancing the depth of understanding provided by parametric knowledge with the recency offered by retrieval remains an active area of development for AI search platforms.
The resource-intensive nature of AI search raises questions about scalability and accessibility. Running inference on large language models requires significant computational resources, making each query substantially more expensive than traditional search operations. This cost difference has implications for who can deploy such systems and how broadly they can be used. While traditional search engines can serve billions of queries daily with highly optimized infrastructure, the computational demands of SearchGPT-style systems currently limit their deployment at similar scales. These resource requirements also translate to environmental concerns, as the energy consumption associated with large language model inference exceeds that of traditional search operations. As these systems become more widely used, addressing efficiency challenges through model optimization, caching strategies, and more sustainable computing infrastructure will be essential to minimize their environmental impact while maintaining their advanced capabilities.
Real-World Applications and Use Cases
In enterprise environments, SearchGPT-style systems are revolutionizing knowledge management by making internal information more accessible and actionable. Organizations accumulate vast repositories of documents, communications, and specialized knowledge that traditional search often struggles to navigate effectively. AI-powered search tools can understand the relationships between different pieces of company information, recognize domain-specific terminology, and provide contextually relevant answers to employee questions. A financial analyst might ask about previous market analyses during similar economic conditions, a product manager might seek information about customer feedback across multiple channels, or a new employee might need guidance on company policies and procedures. In each case, AI search can synthesize relevant information from across the organization's knowledge base, saving valuable time and improving decision-making. This enterprise application is particularly valuable because it operates within a bounded domain where factual accuracy can be more easily verified and the stakes of incorrect information are well understood.
Consumer applications showcase how AI search can transform everyday information discovery. From shopping assistants that understand nuanced product preferences to travel planners that can develop comprehensive itineraries based on conversational inputs, these systems are creating more intuitive interfaces for common tasks. Traditional search often requires multiple queries and significant manual filtering to accomplish what AI search can handle in a single conversational interaction. For example, planning a family vacation with specific constraints like budget, accessibility needs, and children's interests might require dozens of separate searches using traditional tools. An AI search assistant can process these requirements holistically, suggesting appropriate destinations, accommodations, and activities while explaining the reasoning behind recommendations. This ability to handle complex, multi-faceted queries with contextual understanding makes SearchGPT particularly valuable for tasks that previously required extensive research or professional assistance.
Educational settings present some of the most promising applications for AI search technology. SearchGPT can adapt explanations to different learning levels, provide step-by-step walkthroughs of complex concepts, and make connections between related topics that might not be immediately apparent. A student struggling with calculus can receive personalized explanations that address their specific points of confusion, while a researcher might use the system to identify interdisciplinary connections or generate novel hypotheses based on existing literature. The conversational nature of these systems allows for an iterative learning process where users can ask follow-up questions, request clarification, or explore tangential topics as their understanding evolves. Traditional search supports education by providing access to resources, but AI search goes further by actively participating in the knowledge transfer process itself, functioning more like a tutor than a library catalog.
Specialized domain applications highlight how AI search can be fine-tuned for specific knowledge areas where traditional search often falls short. In legal research, for example, SearchGPT-style systems can understand complex legal queries, interpret statutes and case law in context, and explain the reasoning behind legal conclusions. Medical professionals can use similar systems to stay updated on research, evaluate treatment options based on patient-specific factors, and understand rare conditions that might not be covered in their direct experience. Scientific researchers can leverage AI search to navigate the exponentially growing body of research literature, identify relevant cross-disciplinary connections, and generate hypotheses based on patterns across multiple studies. These specialized applications often combine the general knowledge of large language models with domain-specific training and retrieval from authoritative sources, creating hybrid systems that balance broad understanding with deep expertise in particular fields.
The Future of Search Technology
The future of search likely lies in the convergence of traditional and AI-powered approaches, combining the strengths of both while addressing their respective limitations. We're already seeing this integration in today's most advanced search systems, where conventional indexing and retrieval mechanisms are enhanced with natural language understanding and generative capabilities. This hybrid approach leverages traditional search's strengths in recency, breadth, and source attribution while incorporating AI search's natural language understanding and synthesis abilities. Users might receive direct answers generated by AI models for straightforward factual queries while still having access to diverse source links for topics requiring multiple perspectives or deeper research. The technical architecture supporting this convergence will likely include both massive web indexes and large language models, with sophisticated orchestration layers determining which approach best serves each specific query type. This integrated paradigm represents not just an incremental improvement but a fundamental reimagining of how search interfaces should function.
Multimodal search capabilities will dramatically expand the types of information we can discover and how we interact with search systems. Future search technologies will seamlessly handle text, images, audio, video, and even interactive content, allowing users to search with whatever input modality is most natural for their query. Someone might upload an image to find similar products, hum a melody to identify a song, or combine a sketch with text to search for design inspiration. SearchGPT-style systems are particularly well-positioned for this multimodal future, as large language models are increasingly being integrated with vision, audio, and other perceptual systems. Traditional search engines are also developing multimodal capabilities, but the generative approach of AI search offers unique advantages for synthesizing information across different modalities. As these capabilities mature, our concept of "searching" will expand beyond text matching to include finding patterns, similarities, and relationships across all forms of digital information.
Personalized, adaptive search experiences will become increasingly sophisticated as systems develop deeper understanding of individual user contexts, preferences, and needs. Future search technologies will consider factors like the user's expertise level in the topic, their previous interactions with similar information, and even their current goals and tasks when determining how to present results. A medical professional and a patient searching for information about the same condition might receive entirely different responses tailored to their respective knowledge levels and needs. This personalization extends beyond just the content of results to include the format, detail level, and even the interface itself. AI search systems are particularly well-suited for this adaptation, as their generative nature allows them to reformulate information for different audiences rather than simply filtering pre-existing content. This capability raises important questions about filter bubbles and information diversity that must be addressed through thoughtful design and transparent user controls.
The integration of search with broader AI agent ecosystems represents perhaps the most transformative potential future direction. Rather than functioning as isolated query-response systems, search technologies will increasingly operate as components within larger AI frameworks that can take actions on behalf of users. We might ask a personal AI assistant to research vacation options, compare prices, check our calendar for available dates, and even make reservations – all through a single conversational interface connected to multiple specialized systems. This evolution transforms search from a discrete activity into a continuous background process that supports our digital experiences. The boundaries between search, recommendation, and action will blur as these systems become more capable of understanding our intentions and carrying them out across multiple services and platforms. This agent-based future represents a significant shift in how we conceptualize search – from finding information to accomplishing goals through AI-mediated interaction with the digital world.
Statistics & Tables: The Data Behind Search Evolution
For a detailed comparison of search technologies, let's examine the key metrics and trends that highlight the differences between traditional search engines and AI-powered alternatives like SearchGPT.
Impact on Information Literacy and Society
The rise of SearchGPT-style systems is transforming not just how we find information but how we relate to knowledge itself. Traditional search engines have shaped a generation of digital citizens who learned to evaluate sources, cross-reference information, and develop critical thinking skills through the process of sifting through results. The shift toward AI-generated direct answers creates both opportunities and challenges for information literacy. On one hand, these systems democratize access to knowledge by removing barriers related to search literacy, making information more accessible to those who struggle with traditional search interfaces or lack the background knowledge to formulate effective queries. On the other hand, the black-box nature of these systems and their tendency to present information with authority can discourage critical evaluation, potentially leading to over-reliance on AI-generated content without appropriate skepticism. Educational institutions now face the challenge of teaching students how to effectively use and critically evaluate both traditional and AI-powered search tools, developing new forms of digital literacy appropriate for this hybrid information landscape.
The societal implications extend beyond individual information seeking to how communities and institutions function. Search technology shapes which voices are heard, which perspectives are represented, and how consensus forms around important issues. Traditional search engines have been criticized for reinforcing existing biases and creating filter bubbles through their personalization algorithms. AI search systems introduce different concerns, as their training data may encode historical biases or overrepresent dominant perspectives. The conversational interface of SearchGPT might create a more personal relationship with information sources, potentially increasing user trust regardless of whether that trust is warranted. As these technologies become increasingly embedded in our information infrastructure, careful consideration of their design, training, evaluation, and governance becomes essential to ensure they serve the broader public interest rather than reinforcing existing power imbalances or creating new ones.
The economics of information is also evolving with these new search paradigms. Traditional search engines built their business models around advertising, creating an incentive structure that prioritized user engagement and commercial relevance. SearchGPT and similar AI systems have yet to establish sustainable business models that align with their technical capabilities and user needs. The significantly higher computational cost per query creates pressure for subscription or per-use pricing, potentially limiting access to those who can afford to pay. Meanwhile, the ability of these systems to synthesize and present information directly threatens the traffic-based revenue models of many content creators and publishers who currently rely on search engines for discovery. Finding economic models that fairly compensate content creators while making AI search widely accessible represents a significant challenge that will shape the future information ecosystem.
Perhaps most profound is the philosophical shift in our relationship with information. Traditional search positioned us as hunters and gatherers in a vast information landscape, developing skills to find, evaluate, and synthesize knowledge ourselves. SearchGPT repositions us as conversational partners with an AI system that does much of this work on our behalf. This shift raises fundamental questions about the role of human judgment, the value of the search process itself (not just its results), and how our cognitive processes might evolve as we outsource more intellectual tasks to AI systems. As we navigate this transition, maintaining a balance that leverages the efficiency and accessibility of AI search while preserving human agency and critical thinking will be essential to realizing the potential of these technologies while mitigating their risks.
Conclusion
The comparison between SearchGPT and traditional search engines reveals not merely two competing technologies but two distinct paradigms for human-information interaction. Traditional search has evolved over decades into a sophisticated system that connects users with the vast expanse of online content through optimized indexing and retrieval mechanisms. SearchGPT and similar AI-powered systems represent a fundamentally different approach that emphasizes natural language understanding, conversational interaction, and direct answer generation. Each approach brings unique strengths and faces particular challenges that make them complementary rather than purely competitive technologies. The future of search likely lies not in choosing between these paradigms but in thoughtfully integrating them to create more powerful, accessible, and trustworthy information discovery experiences.
The data presented throughout this article points to a nuanced picture where different search technologies excel in different contexts. Traditional search engines maintain advantages in recency, source transparency, resource efficiency, and factual verification. SearchGPT demonstrates superior capabilities in natural language understanding, complex query processing, information synthesis, and conversational interaction. User preferences reflect this divide, with satisfaction rates varying significantly depending on query type and information needs. The market trends suggest a gradual shift toward AI-powered and hybrid approaches, though traditional search remains dominant and continues to serve critical functions that generative systems cannot yet replace. This diversity of capabilities argues for an ecosystem of search technologies rather than a winner-takes-all outcome.
As these technologies continue to evolve, the line between them grows increasingly blurred. Traditional search engines are incorporating more AI-powered natural language understanding and direct answer capabilities, while SearchGPT-style systems are improving their retrieval mechanisms and source attribution. This convergence suggests that the future search experience will likely combine elements of both approaches – maintaining the breadth, transparency, and recency of traditional search while adding the conversational interface, contextual awareness, and synthesis capabilities of AI systems. The technical challenges of achieving this integration remain significant, but the potential benefits for users justify the continued investment and innovation in this space.
The ongoing transformation of search technology represents more than just a technical evolution – it reflects changing expectations about how we interact with information and technology itself. Users increasingly expect systems that understand them rather than requiring them to understand the system. They seek not just access to information but assistance in making sense of an increasingly complex and overwhelming digital landscape. The shift from keyword matching to conversational search mirrors broader trends toward more natural, intuitive human-computer interaction across all domains. As search continues to evolve, maintaining focus on human needs, values, and capabilities will be essential to ensuring these technologies truly enhance our relationship with information rather than simply changing how we access it.
Additional Resources
For readers interested in exploring the topics covered in this article in greater depth, the following resources provide valuable insights from diverse perspectives:
The Future of Search: AI Augmentation vs. Replacement - A comprehensive analysis of how AI technologies are being integrated into search ecosystems and the implications for users, publishers, and platform providers.
Retrieval-Augmented Generation: Bridging Traditional and AI Search - An in-depth technical explanation of how modern AI search systems combine parametric knowledge with retrieval mechanisms to address limitations of pure generative approaches.
Evaluating Search Quality: Beyond Click-Through Rates - A research-backed discussion of emerging metrics and methodologies for assessing search quality in both traditional and AI-powered systems.
Information Literacy in the Age of AI - An educational perspective on how search technologies are changing how we teach critical thinking and information evaluation skills.
The Environmental Impact of Search Technologies - A detailed assessment of the resource requirements and environmental footprint of different search approaches, with recommendations for more sustainable practices.