Prompt Engineering Advancements & Sophisticated Techniques
Discover the latest advancements in prompt engineering and learn sophisticated techniques for controlling AI outputs. Explore how these innovations are reshaping AI interactions and enhancing the quality of AI-generated content.
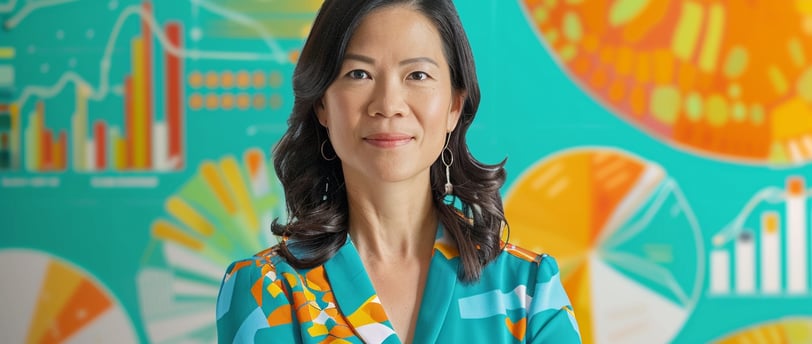
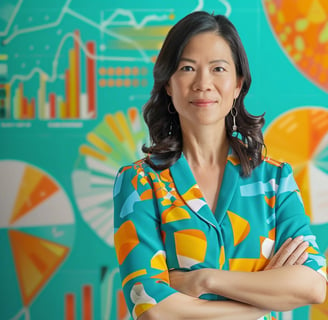
In the rapidly evolving landscape of artificial intelligence (AI), one of the most exciting developments is the field of prompt engineering. This discipline focuses on crafting and optimizing inputs, or "prompts," to guide AI models in producing desired outputs. As AI models become more sophisticated, so too must the techniques used to interact with them. This article will delve into the latest advancements in prompt engineering, exploring how these techniques are enhancing AI capabilities and revolutionizing how we communicate with machines.
Understanding Prompt Engineering
Definition and Importance
Prompt engineering is the process of designing and refining inputs to guide generative AI systems toward producing specific, high-quality outputs. It encompasses a wide range of skills and techniques that are essential for effectively interfacing with and developing large language models (LLMs). The importance of prompt engineering lies in its ability to bridge the gap between human intent and AI understanding, ensuring that the outputs generated are relevant, accurate, and useful. This becomes particularly important as AI becomes more integrated into various applications and research topics, where the quality of the input directly influences the quality of the output 1.
Evolution of Prompt Engineering
The evolution of prompt engineering has been marked by significant milestones. Initially, prompt engineering involved simple trial-and-error methods to get the AI model to output the desired outcome. However, with the advent of more sophisticated AI models like ChatGPT, prompt engineering has emerged as a critical business skill. This shift has led to the development of various prompting techniques and tools that enhance the interaction between humans and AI models 2.
Key Components of Prompt Engineering
Prompt engineering involves several key components:
Prompt Design: Crafting the initial input that guides the AI model. This includes specifying the task, providing context, and setting the tone or style of the output.
Prompt Refinement: Iteratively improving the prompt based on the AI's responses to achieve the desired outcome. This process involves adjusting the language, structure, and details of the prompt.
Prompt Testing: Evaluating the effectiveness of different prompts through systematic testing. This helps in identifying the most effective prompts for specific tasks.
Prompt Validation: Ensuring that the prompts are robust and reliable across different scenarios and use cases. This involves validating the prompts against various criteria such as accuracy, relevance, and bias 1.
Advanced Prompt Engineering Techniques
Chain-of-Thought Prompting
Chain-of-thought prompting (CoT) is an advanced technique that involves breaking down a complex task into intermediate steps, or "chains of reasoning." This approach helps the AI model achieve better language understanding and generate more accurate outputs. By providing step-by-step reasoning, CoT prompts guide the AI through the problem-solving process, making it easier for the model to comprehend and respond to complex queries 3.
Multimodal Prompt Engineering
The integration of multimodal capabilities in AI models has opened new frontiers in prompt engineering. Multimodal models can process and respond to prompts that include a mix of text, images, and even audio inputs. This advancement is significant as it paves the way for more comprehensive AI applications that can understand and interact in a way that more closely mimics human perception and communication. For example, a multimodal AI model can generate descriptions of images, translate text into different languages, and even create audio responses based on textual inputs 4.
Real-Time Prompt Optimization
Advancements in real-time prompt optimization technology have enabled AI models to provide instant feedback on the effectiveness of prompts. This technology assesses the prompt's clarity, potential for bias, and alignment with the desired outcome, offering suggestions for improvement. Real-time guidance is invaluable for both novice and experienced users, streamlining the process of crafting effective prompts. This technology is particularly useful in domains where prompt engineering is integrated with domain-specific AI models, ensuring that the prompts are tailored to the specific requirements of the domain 4.
Automatic Prompt Engineering
Automatic prompt engineering involves using one AI model to compose prompts for another AI model. This approach leverages the capabilities of large language models (LLMs) to generate prompts that are optimized for specific tasks. The automatic prompt engineer algorithm uses a beam search over prompts, systematically exploring different prompt variations to identify the most effective ones. This method reduces the manual effort required in prompt engineering and enhances the efficiency of the process 2.
Domain-Specific Prompt Engineering
Domain-specific prompt engineering involves tailoring prompts to the specific requirements of a particular domain or application. This approach ensures that the AI model's outputs are relevant and accurate within the context of the domain. For example, in the healthcare domain, prompts can be designed to guide AI models in generating diagnostic reports, treatment plans, and patient communication. Similarly, in the finance domain, prompts can be crafted to help AI models generate financial reports, investment recommendations, and risk assessments 4.
Retrieval-Augmented Generation (RAG)
Retrieval-Augmented Generation (RAG) is a technique that combines the strengths of retrieval-based and generative models. RAG involves retrieving relevant information from a large dataset and using it to augment the generation process. This approach ensures that the AI model's outputs are grounded in factual information, enhancing the accuracy and reliability of the generated content. RAG is particularly useful in applications where the AI model needs to generate content based on a large corpus of data, such as in legal research, scientific writing, and customer support 5.
AI Agents and Prompt Engineering
The development of AI agents has introduced new dimensions to prompt engineering. AI agents are autonomous systems that can interact with users and other AI models to perform specific tasks. Prompt engineering for AI agents involves designing prompts that guide the agents in understanding user intent, retrieving relevant information, and generating appropriate responses. This approach requires a deep understanding of the AI agent's capabilities and the context in which it operates 5.
Applications of Prompt Engineering
Natural Language Processing
Prompt engineering plays a crucial role in natural language processing (NLP) applications. By crafting effective prompts, AI models can generate human-like text, translate languages, summarize documents, and even engage in conversations. For example, in customer service, prompts can be designed to help AI models generate responses to customer queries, providing quick and accurate information. In content creation, prompts can guide AI models in generating articles, reports, and creative writing, enhancing productivity and creativity 2.
Image and Audio Generation
With the advent of multimodal AI models, prompt engineering is now being applied to image and audio generation. Prompts can be designed to guide AI models in generating images based on textual descriptions, creating audio content from text, and even translating audio content into different languages. This opens up new possibilities in fields such as art, music, and entertainment, where AI can assist in creating and enhancing creative content 4.
Decision Support Systems
In decision support systems, prompt engineering helps AI models generate insights and recommendations based on data analysis. Prompts can be crafted to guide AI models in analyzing data, identifying patterns, and generating reports that support decision-making. For example, in healthcare, prompts can help AI models generate diagnostic reports and treatment plans based on patient data. In finance, prompts can assist AI models in generating investment recommendations and risk assessments based on financial data 4.
Challenges in Prompt Engineering
Precision and Scalability
Despite its advancements, prompt engineering faces challenges in precision and scalability. Crafting prompts that are precise enough to generate accurate outputs while being scalable across different applications and domains remains a significant challenge. This requires continuous refinement and validation of prompts to ensure their effectiveness and reliability 5.
Bias and Fairness
Another challenge in prompt engineering is ensuring that the prompts are free from bias and fairness issues. Biased prompts can lead to biased outputs, which can have serious implications in sensitive applications such as healthcare and finance. Addressing this challenge requires a deep understanding of the data and the context in which the AI model operates, as well as the development of techniques to minimize bias in prompts 6.
Domain-Specific Limitations
Prompt engineering also faces domain-specific limitations. Crafting prompts that are effective across different domains requires a deep understanding of the domain's specific requirements and constraints. This can be challenging, as the knowledge and expertise required to craft effective prompts may vary significantly across domains. Overcoming this challenge requires the development of domain-specific prompt engineering techniques and tools 4.
Conclusion
Prompt engineering has emerged as a critical discipline in the field of AI, offering sophisticated techniques for controlling AI outputs. From chain-of-thought prompting to multimodal and real-time prompt optimization, these advancements are enhancing the quality and relevance of AI-generated content. As AI continues to evolve, prompt engineering will play an increasingly important role in shaping how we interact with and benefit from these powerful tools. Embracing these techniques and staying informed about the latest developments will be key to harnessing the full potential of AI in various applications.
FAQ Section
Q: What is prompt engineering?
A: Prompt engineering is the process of designing and refining inputs to guide generative AI systems toward producing specific, high-quality outputs. It involves crafting prompts that guide AI models in generating relevant and accurate responses.
Q: Why is prompt engineering important?
A: Prompt engineering is important because it bridges the gap between human intent and AI understanding, ensuring that the outputs generated are relevant, accurate, and useful. It plays a crucial role in enhancing the quality of AI-generated content and improving the interaction between humans and AI models.
Q: What are some advanced prompt engineering techniques?
A: Advanced prompt engineering techniques include chain-of-thought prompting, multimodal prompt engineering, real-time prompt optimization, automatic prompt engineering, and domain-specific prompt engineering. These techniques help in crafting effective prompts that guide AI models in generating high-quality outputs.
Q: How does chain-of-thought prompting work?
A: Chain-of-thought prompting involves breaking down a complex task into intermediate steps, or "chains of reasoning." This approach helps the AI model achieve better language understanding and generate more accurate outputs by providing step-by-step reasoning.
Q: What is multimodal prompt engineering?
A: Multimodal prompt engineering involves designing prompts that include a mix of text, images, and audio inputs. This approach enables AI models to generate responses based on multimodal inputs, enhancing the comprehensiveness and interactivity of AI applications.
Q: What is real-time prompt optimization?
A: Real-time prompt optimization is a technique that provides instant feedback on the effectiveness of prompts. It assesses the prompt's clarity, potential for bias, and alignment with the desired outcome, offering suggestions for improvement in real-time.
Q: What is automatic prompt engineering?
A: Automatic prompt engineering involves using one AI model to compose prompts for another AI model. This approach leverages the capabilities of large language models (LLMs) to generate prompts that are optimized for specific tasks, reducing the manual effort required in prompt engineering.
Q: What is domain-specific prompt engineering?
A: Domain-specific prompt engineering involves tailoring prompts to the specific requirements of a particular domain or application. This approach ensures that the AI model's outputs are relevant and accurate within the context of the domain.
Q: What are some applications of prompt engineering?
A: Prompt engineering is applied in various fields such as natural language processing, image and audio generation, and decision support systems. It helps AI models generate human-like text, translate languages, summarize documents, create images and audio content, and generate insights and recommendations based on data analysis.
Q: What are the challenges in prompt engineering?
A: Challenges in prompt engineering include precision and scalability, bias and fairness, and domain-specific limitations. Crafting prompts that are precise, unbiased, and effective across different domains requires continuous refinement and validation.