Industry-Specific ChatGPT from Finance to Manufacturing
Discover how businesses across sectors are creating tailored ChatGPT solutions to address industry-specific challenges, enhance productivity, and drive innovation in 2025.
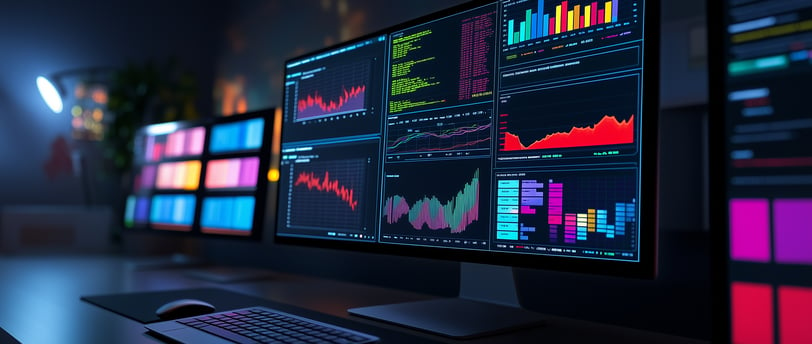
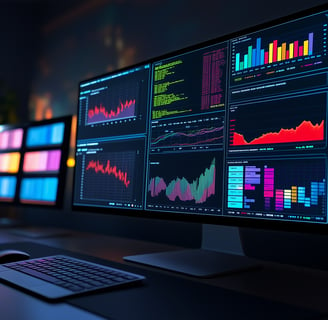
In a Tokyo-based investment firm, an analyst receives real-time market insights during a volatile trading session, not from a human colleague but from an AI assistant trained on proprietary financial data. Meanwhile, in a German automotive plant, production supervisors consult with a specialized AI system that has mastered the intricacies of their manufacturing processes, helping them identify potential bottlenecks before they occur. Across the globe in a Boston hospital, physicians reduce their administrative burden using a healthcare-specific AI that understands medical terminology and regulatory compliance requirements. What connects these diverse scenarios? All leverage industry-specific customizations of ChatGPT, transforming a general-purpose AI system into specialized tools tailored to unique business contexts. The age of one-size-fits-all artificial intelligence is rapidly giving way to precision AI implementations that understand the language, challenges, and opportunities within specific sectors. This evolution represents one of the most significant developments in enterprise AI adoption since ChatGPT's emergence, enabling organizations to capture value that would remain inaccessible with generic AI deployments. In this comprehensive exploration, we'll examine how different industries are customizing ChatGPT to their unique needs, the methodologies driving these implementations, and the competitive advantages they create.
The Evolution of Industry-Specific AI
The journey toward industry-specialized AI didn't begin with ChatGPT but has accelerated dramatically with its arrival and subsequent advancements. Early industry-specific AI applications were often narrow in scope, utilizing rules-based systems for tasks like fraud detection in banking or quality control in manufacturing. These systems excelled at specific, predefined tasks but lacked flexibility and required extensive coding for any modifications. The introduction of machine learning algorithms in the 2010s expanded capabilities but still demanded substantial data science expertise and custom development for each use case. When large language models like ChatGPT emerged, they initially impressed with their general knowledge but fell short when confronted with industry-specific terminology, regulations, or processes. This limitation became evident as organizations deployed these models and found them struggling with specialized vocabulary, misunderstanding industry contexts, or providing generic answers to sector-specific questions.
The true transformation began when enterprises developed methodologies to customize these foundation models for their particular domains. Rather than building AI systems from scratch, organizations discovered they could leverage the vast capabilities of general-purpose models like ChatGPT and adapt them through various techniques including fine-tuning, retrieval-augmented generation, and custom instructions. This approach dramatically reduced the technical barriers to creating specialized AI applications while maintaining the sophisticated capabilities of advanced language models. The result has been a proliferation of industry-tailored AI assistants that combine the linguistic flexibility and reasoning capabilities of general AI with deep domain understanding. These specialized implementations now serve as competitive differentiators, with organizations leveraging custom AI solutions that understand their business language, workflows, and challenges in ways generic systems cannot match. As we'll explore, these customizations aren't merely technical achievements but strategic assets that transform how work gets done across sectors.
Finance and Banking: Precision in a Regulated Environment
The financial services industry presents a perfect case study in ChatGPT customization, balancing the need for innovation with stringent regulatory requirements. Major financial institutions have created specialized versions of ChatGPT that understand complex financial instruments, regulatory frameworks like Basel III and Dodd-Frank, and institution-specific policies and procedures. These systems assist investment analysts by synthesizing market reports, earnings calls, and proprietary research to generate actionable insights with appropriate risk disclosures. For wealth management, customized ChatGPT implementations create personalized client communications that maintain compliance with regulations governing financial advice while explaining complex investment concepts in accessible language. Perhaps most impressively, these systems can interpret and compare financial regulations across multiple jurisdictions, helping global institutions navigate the complex web of requirements they face worldwide.
The technical approach to these customizations often combines multiple methods. Many financial institutions utilize retrieval-augmented generation, connecting ChatGPT to private knowledge bases containing proprietary research, compliance guidelines, and historical market analyses. This allows the AI to reference and cite specific internal documents when providing answers. Fine-tuning on financial datasets helps the model understand specialized terminology related to derivatives, structured products, and other complex financial instruments. Custom instruction sets establish guardrails ensuring the AI acknowledges its limitations and directs users to human compliance officers for certain types of questions. Leading banks report substantial efficiency gains, with one global institution documenting a 37% reduction in time spent on regulatory research and a 42% improvement in client query response time. Beyond these operational improvements, financial institutions note that customized AI systems enable more personalized client experiences while maintaining consistent adherence to compliance requirements—a balance that has historically been difficult to achieve at scale.
Healthcare: Balancing Clinical Precision with Human Care
The healthcare sector presents unique challenges for AI customization, requiring exceptional accuracy while navigating sensitive patient information and complex medical terminology. Healthcare-specific ChatGPT implementations now assist medical professionals with clinical documentation, medical coding, treatment plan development, and literature reviews. These systems understand thousands of medical conditions, pharmaceutical names, procedural terminology, and regulatory frameworks like HIPAA in the United States or GDPR in Europe. Leading medical institutions have developed AI assistants that can analyze patient records (with appropriate privacy protections) to suggest potential diagnoses for physician consideration, identify possible medication interactions, and summarize patient histories before appointments. Unlike general AI models that might provide dangerously incomplete medical information, properly customized healthcare AI systems recognize their limitations and refer clinicians to authoritative sources when certainty is insufficient.
The customization of these systems typically involves careful fine-tuning on medical literature, clinical guidelines, and de-identified patient records. Many healthcare implementations utilize retrieval-augmented generation to connect the AI system with medical knowledge bases, ensuring recommendations align with the latest research and institutional protocols. Custom instruction sets establish appropriate clinical boundaries, ensuring the AI clearly differentiates between evidence-based recommendations and areas requiring physician judgment. Healthcare organizations report significant efficiency improvements, with one hospital network documenting a 28% reduction in documentation time and a 34% increase in coding accuracy. Physicians note that these AI systems help them focus more on patient care by handling administrative burdens and providing quick access to relevant medical information. Importantly, well-designed healthcare AI implementations recognize the irreplaceable value of human clinical judgment and position themselves as assistive tools rather than autonomous decision-makers, preserving the essential human element of healthcare while enhancing efficiency and accuracy.
Manufacturing: From Generalist to Production Specialist
The manufacturing sector has embraced ChatGPT customization to address challenges ranging from production optimization to quality control and supply chain management. Industry leaders have created specialized AI systems that understand manufacturing processes, equipment specifications, material properties, and production workflows specific to their operations. These systems assist engineers with troubleshooting by analyzing equipment performance data and maintenance histories to suggest potential causes for production issues. For production planning, customized ChatGPT implementations help optimize schedules by considering material availability, equipment capacity, labor constraints, and order priorities. Quality assurance teams leverage AI assistants that understand quality standards, testing procedures, and statistical process control methods to identify potential quality issues before they affect finished products. This sector demonstrates how general-purpose AI can transform into systems with deep specialized knowledge of physical production environments.
The technical approach in manufacturing often emphasizes integration with existing operational systems. Many implementations connect ChatGPT to manufacturing execution systems, enterprise resource planning tools, and IoT sensor networks to incorporate real-time production data into AI responses. Fine-tuning on equipment manuals, maintenance records, and production logs helps the AI understand the specific machinery and processes used in a facility. Custom instruction sets establish parameters for recommendations, ensuring the AI considers practical constraints like available equipment capacity or maintenance schedules. Manufacturing organizations report significant operational improvements, with one automotive component manufacturer documenting a 22% reduction in production downtime and a 17% improvement in first-pass yield after implementing a customized ChatGPT solution. Beyond these efficiency metrics, manufacturers note that AI systems enable knowledge democratization, giving less experienced staff access to institutional expertise that would otherwise take years to develop. This capability proves particularly valuable amid demographic shifts in the manufacturing workforce as experienced personnel retire and new workers enter the field.
Retail and E-commerce: Personalization at Scale
The retail sector has leveraged ChatGPT customization to enhance customer experiences, optimize merchandising decisions, and streamline operations across physical and digital channels. Leading retailers have developed specialized AI systems that understand product catalogs, seasonal trends, customer segmentation models, and omnichannel fulfillment options. These systems assist merchandising teams by analyzing sales data, social media trends, and competitor activities to recommend assortment changes and pricing strategies. For customer service, customized ChatGPT implementations handle product inquiries, sizing assistance, and post-purchase support with knowledge of specific product lines and return policies. Marketing teams utilize AI assistants that understand target customer segments and brand voice to generate consistent content across channels while adapting messaging to different platforms and customer needs. The retail applications demonstrate how general AI can evolve into systems that understand complex product taxonomies and consumer behavior patterns.
The customization approach in retail often emphasizes frequent model updates to account for rapidly changing inventory and seasonal shifts. Many implementations connect ChatGPT to product information management systems, customer relationship management platforms, and inventory systems to provide accurate, real-time information about product availability and specifications. Fine-tuning on customer service transcripts, product descriptions, and marketing materials helps the AI understand brand-specific language and product attributes. Custom instruction sets establish tone and style requirements, ensuring the AI maintains consistent brand voice across customer interactions. Retail organizations report substantial business impact, with one department store chain documenting a 45% reduction in customer service escalations and a 27% increase in conversion rate for AI-assisted online shopping sessions. Beyond these direct metrics, retailers note that customized AI systems allow them to provide personalized service at scale, recreating elements of high-touch in-store experiences in digital channels where personal attention would otherwise be impossible to maintain consistently across thousands of simultaneous customer interactions.
Legal Services: Precision in a Precedent-Based Domain
The legal industry presents a fascinating case study in ChatGPT customization, requiring exceptional precision in a field governed by precedent, jurisdiction-specific regulations, and complex procedural requirements. Leading law firms and legal departments have created specialized AI systems that understand legal terminology, citation formats, procedural rules, and jurisdiction-specific statutes. These systems assist attorneys with legal research by analyzing case law, identifying relevant precedents, and summarizing key holdings that might apply to current matters. For contract review, customized ChatGPT implementations help identify non-standard clauses, compare language against approved templates, and flag potential risks for attorney review. Litigation teams leverage AI assistants that understand evidentiary rules, filing requirements, and procedural timelines to help manage complex case workflows. The legal applications demonstrate how general-purpose AI can transform into systems with the precision required in high-stakes professional environments where errors carry significant consequences.
The technical approach in legal settings often emphasizes authoritative sources and clear limitations. Many implementations utilize retrieval-augmented generation, connecting ChatGPT to legal databases containing statutes, regulations, case law, and internal knowledge bases. This ensures the AI can reference and cite specific legal authorities when providing information. Fine-tuning on legal documents, court opinions, and jurisdiction-specific materials helps the model understand specialized terminology and proper citation formats. Custom instruction sets establish appropriate boundaries, ensuring the AI clearly indicates when issues require professional judgment and avoids providing definitive conclusions on complex legal questions. Legal organizations report meaningful efficiency improvements, with one corporate legal department documenting a 32% reduction in time spent on initial contract review and a 29% improvement in response time for internal legal queries. Beyond these operational metrics, legal professionals note that customized AI systems enable them to focus on strategic aspects of their work by handling routine research and document analysis tasks that previously consumed substantial time but added limited strategic value.
The Technical Foundations of Industry Customization
Behind these diverse industry implementations lie several key technical approaches that transform general AI into specialized tools. The first approach, fine-tuning, involves additional training of the base model on industry-specific datasets. This process helps the model learn specialized vocabulary, standard practices, and common patterns within a particular domain. For example, healthcare implementations often fine-tune models on medical literature and de-identified clinical notes to improve understanding of medical terminology. The second approach, retrieval-augmented generation (RAG), connects the AI to external knowledge bases containing authoritative information. Rather than relying solely on information learned during training, the system retrieves relevant documents from these knowledge bases to inform its responses. This approach proves particularly valuable for domains with rapidly changing information or organization-specific knowledge that wouldn't be included in general training data.
Custom instructions represent a third approach, establishing parameters for how the AI should respond in different scenarios. These instructions might define tone requirements, information boundaries, escalation criteria, or citation standards. For instance, financial implementations often include instructions to maintain a conservative tone when discussing investment risks and to cite specific regulations when addressing compliance questions. The fourth approach, supervised learning from human feedback, involves human experts reviewing AI responses and providing corrections that help the system improve over time. This approach enables continuous refinement based on real-world usage patterns and expert input. Most sophisticated implementations combine multiple techniques, using fine-tuning for domain understanding, RAG for access to current information, custom instructions for consistent behavior, and human feedback for ongoing improvement. This multi-faceted approach creates AI assistants that not only understand industry terminology but also operate according to domain-specific best practices, follow appropriate information boundaries, and continuously improve through expert guidance.
Implementation Challenges and Best Practices
Despite the compelling benefits, implementing industry-specific AI customizations presents significant challenges that organizations must navigate. Data quality and availability often pose the first hurdle, as effective customization requires substantial high-quality, industry-specific training data. Organizations frequently discover that their internal documents contain inconsistencies, outdated information, or implicit knowledge that human experts understand but isn't explicitly documented. Addressing these issues typically requires dedicated data curation efforts before customization can begin. Governance frameworks present another challenge, as organizations must establish clear policies regarding what the AI can access, how it should handle sensitive information, and when human review is required. Developing these frameworks often involves collaboration across legal, compliance, IT, and business units to ensure appropriate safeguards while maintaining usability.
Integration with existing systems presents technical challenges, requiring secure connections between the AI and various enterprise applications while maintaining appropriate access controls. Organizations must also address workforce concerns, as employees may worry about job displacement or resist adoption if they perceive the AI as threatening rather than assistive. Successful implementations typically follow several best practices to overcome these challenges. First, they begin with high-value, well-defined use cases rather than attempting broad deployment immediately. This focused approach allows organizations to demonstrate value while refining their customization methodology. Second, they invest in comprehensive change management, including training programs that help employees understand how to work effectively with AI assistants. Third, they establish clear metrics to evaluate success, tracking both technical performance (accuracy, response relevance) and business outcomes (time savings, quality improvements). Finally, successful implementations create feedback loops that capture expert input for continuous improvement, recognizing that customization is an ongoing process rather than a one-time project. This structured approach helps organizations navigate the complexities of industry-specific AI implementation while maximizing return on investment.
Conclusion
The evolution of ChatGPT from general-purpose assistant to industry-specific expert represents one of the most significant developments in enterprise AI adoption. As we've explored across finance, healthcare, manufacturing, retail, and legal services, these customizations transform generic AI capabilities into specialized tools that understand the unique language, challenges, and opportunities within specific sectors. The competitive advantages created by these implementations extend beyond operational efficiency, enabling organizations to enhance customer experiences, improve decision quality, accelerate innovation, and preserve institutional knowledge in unprecedented ways. The organizations gaining the greatest advantage are those treating AI customization not as a technical project but as a strategic capability that evolves alongside their business needs. They recognize that the goal isn't merely to deploy AI but to develop systems that truly understand their business context and can participate meaningfully in their most valuable workflows.
Looking ahead, we can expect the boundary between general and specialized AI systems to continue blurring. Advanced customization techniques will likely become more accessible, enabling even smaller organizations to develop industry-tailored AI implementations without extensive technical resources. Integration between AI systems and enterprise applications will deepen, creating more cohesive digital environments where AI assistants understand not just industry context but also organization-specific workflows and objectives. As these developments unfold, the question for business leaders will shift from whether to implement industry-specific AI to how quickly they can develop this strategic capability relative to competitors. Organizations that establish effective methodologies for AI customization today are building foundations for sustained competitive advantage in an economy increasingly shaped by artificial intelligence. The age of generic AI is giving way to an era of precision AI implementation, where understanding specific business contexts becomes as important as technological sophistication.
Frequently Asked Questions
What makes industry-specific ChatGPT implementations different from the standard version? Industry-specific implementations are customized with specialized knowledge, terminology, and practices relevant to particular sectors. These customizations typically include fine-tuning on industry data, connection to proprietary knowledge bases, and custom instructions that guide the AI to operate according to industry best practices and regulatory requirements.
How long does it typically take to develop an industry-specific ChatGPT implementation? Development timelines vary widely based on complexity and scope, ranging from 4-6 weeks for narrowly focused implementations to 6-12 months for comprehensive enterprise solutions. The most time-intensive aspects usually involve data preparation, integration with existing systems, and governance framework development rather than the technical customization itself.
What types of data are needed to effectively customize ChatGPT for a specific industry? Effective customization typically requires industry glossaries, internal documentation, process descriptions, compliance guidelines, product information, and examples of expert responses to common questions. Many organizations also include anonymized records of human expert interactions to help the AI learn from actual problem-solving approaches.
How do organizations measure the return on investment for industry-specific AI customization? Organizations typically measure both efficiency metrics (time savings, increased throughput, reduced errors) and strategic value metrics (improved decision quality, enhanced customer experience, knowledge preservation). The most sophisticated measurement approaches also consider opportunity costs avoided and new capabilities enabled by AI assistance.
What governance considerations should organizations address when implementing customized ChatGPT? Key governance considerations include data privacy controls, information access boundaries, response approval workflows for sensitive topics, escalation criteria for human review, compliance verification processes, and audit trails for AI-assisted decisions. These frameworks should align with industry regulatory requirements and organizational risk policies.
Can small and medium-sized businesses effectively implement industry-specific ChatGPT solutions? Yes, with the emergence of specialized implementation partners and increasingly accessible customization tools, SMBs can develop industry-specific implementations by focusing on clearly defined, high-value use cases. Industry consortium approaches can also help smaller organizations share development costs while maintaining competitive differentiation in implementation.
How do organizations maintain and update their customized ChatGPT implementations? Effective maintenance typically involves regular review cycles, feedback collection mechanisms from users, monitoring for drift between AI responses and current best practices, and structured update processes for incorporating new information and capabilities. Many organizations establish dedicated AI governance teams to oversee this ongoing maintenance.
What are the most common challenges organizations face when customizing ChatGPT for their industry? Common challenges include data quality issues, integration complexities with legacy systems, establishing appropriate trust boundaries, addressing workforce concerns about AI adoption, and developing governance frameworks that balance innovation with risk management. Organizations often underestimate the change management aspects of implementation.
How do industry-specific implementations handle confidential or proprietary information? Sophisticated implementations typically employ multiple protection mechanisms including data access controls, information boundary enforcement, pattern recognition to identify and protect sensitive content, appropriate anonymization techniques, and clear policies regarding information the AI should not reproduce or reference even if accessed accidentally.
What skills and roles are needed to successfully implement and maintain industry-customized ChatGPT solutions? Successful teams typically combine industry domain experts who understand sector-specific requirements, AI engineers who implement technical customizations, integration specialists who connect AI with existing systems, governance experts who establish appropriate guidelines, and change management professionals who facilitate adoption. The most critical role is often the "translator" who bridges industry expertise and technical capabilities.
Additional Resources
ChatGPT Enterprise Features and Benefits - An in-depth guide to enterprise-grade ChatGPT features that support industry customization.
ChatGPT for Consulting - Explore how consulting firms are leveraging customized ChatGPT solutions for client engagements.
ChatGPT Implementation - A practical guide to implementing ChatGPT solutions in enterprise environments.
Prompt Engineering Guide - Learn about advanced prompt engineering techniques that enhance industry-specific AI applications.
Enterprise AI Integration and ChatGPT Enterprise Features - Comprehensive overview of integration approaches for enterprise AI implementations.