GDPR's effect on scientific research and statistical analysis
Explore how GDPR regulations have transformed scientific research methodologies and statistical analysis practices while balancing data protection with innovation in the modern research landscape.
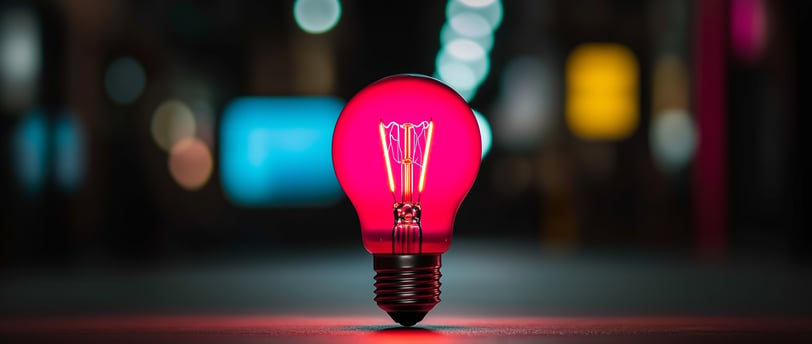
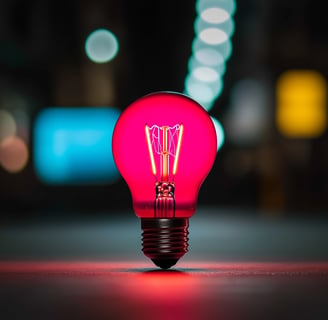
The digital revolution has unleashed unprecedented access to data, transforming scientific research and statistical analysis into powerful engines of discovery. However, with great data comes great responsibility. The General Data Protection Regulation (GDPR), implemented by the European Union in 2018, represents one of the most significant regulatory frameworks governing data usage in recent history. For researchers and statisticians accustomed to relatively unrestricted data access, the introduction of GDPR created ripples that continue to reshape the research landscape. This tension between data protection and scientific advancement raises a critical question: How can we protect individual privacy rights while ensuring that research essential to human progress continues unimpeded? This article explores the multifaceted impact of GDPR on scientific research and statistical analysis, examining both the challenges and opportunities that have emerged from this regulatory framework.
Understanding GDPR's Framework for Research
The GDPR recognizes the importance of scientific research and statistical analysis by providing specific provisions to facilitate legitimate research activities. Under Article 89, the regulation allows certain exemptions for research purposes, acknowledging that scientific investigation often requires processing personal data beyond its original collection purpose. However, these exemptions come with important qualifications that researchers must carefully navigate to maintain compliance.
GDPR establishes several key principles for researchers, including purpose limitation, data minimization, and storage limitations. Researchers must now clearly define and justify their data needs before collection begins, focusing only on information essential to their study objectives. Additionally, the regulation emphasizes the implementation of appropriate technical and organizational safeguards to protect the rights of data subjects. These safeguards may include pseudonymization, encryption, or data anonymization techniques that reduce identifiability while preserving analytical value.
The foundation of these requirements stems from the purpose of GDPR - safeguarding individual privacy while enabling responsible data use. For research organizations, understanding these foundational principles is crucial for building compliant research methodologies that respect both regulatory requirements and scientific integrity. While navigating these requirements may initially seem daunting, they ultimately promote more methodologically sound research by encouraging researchers to carefully consider their data needs and protection strategies from the project's inception.
The Informed Consent Challenge
Perhaps the most significant change GDPR introduced to the research landscape is its stringent requirements for informed consent. Under GDPR, consent must be freely given, specific, informed, unambiguous, and indicated through clear affirmative action. This represents a considerable departure from previous research practices where broad, general consent was often deemed sufficient for wide-ranging research purposes.
For longitudinal studies or research involving large data sets, obtaining GDPR-compliant consent presents substantial challenges. Researchers must now provide detailed information about how data will be used, how long it will be stored, and who will have access to it. Additionally, participants must be informed of their right to withdraw consent at any time, potentially compromising the integrity of ongoing research if significant numbers exercise this right. This has forced many research institutions to completely redesign their consent processes and documentation.
Particularly challenging are situations where researchers wish to conduct secondary analyses on previously collected data. Many historical data sets were gathered under consent terms that do not meet GDPR standards, creating uncertainty about whether such data can continue to be used. This has prompted some research institutions to undertake massive re-consent exercises, while others have explored alternative legal bases for processing, such as "legitimate interests" or "public interest" provisions. Understanding consent in GDPR has become essential for research organizations that must balance scientific objectives with respect for individual rights.
Data Minimization and Research Scope
The principle of data minimization stands at the core of GDPR compliance for scientific research. This principle requires that personal data processing be limited to what is necessary for the specific research purpose, creating a fundamental tension with exploratory research approaches that have traditionally collected broad data sets to discover unexpected relationships or generate new hypotheses.
Researchers must now justify each data element collected in relation to specific research questions, developing more focused research designs that clearly connect data needs to research objectives. This approach compels investigators to think critically about their data requirements, potentially leading to more efficient and targeted research methodologies. However, it also limits the serendipitous discoveries that sometimes emerge from analyses of seemingly unrelated variables.
To address these limitations, researchers have developed creative approaches to maintain compliance while preserving research flexibility. These include tiered consent models that allow participants to authorize varying levels of data use, and the implementation of data minimization strategies that reduce personal data exposure while maintaining scientific utility. Additionally, some institutions have established data governance committees that review proposed secondary analyses to ensure they align with original consent parameters or qualify for exemptions under Article 89.
Anonymization and Pseudonymization in Research Data
GDPR differentiates between anonymous data, which falls outside its scope, and pseudonymized data, which remains subject to regulation but with potential exemptions for research. This distinction has profound implications for how research data is processed and stored. True anonymization, meaning the irreversible removal of all identifying elements, offers researchers greater freedom but may significantly reduce data utility for certain types of studies.
Pseudonymization—replacing identifying information with codes while maintaining a separate key to re-identify subjects when necessary—has emerged as a critical technique for maintaining both data protection and research value. However, researchers must implement robust technical and organizational safeguards to protect these identification keys. The rise of sophisticated data mining techniques has complicated this landscape, as data once considered anonymous may become identifiable when combined with other available information sources.
To navigate these challenges, researchers have developed advanced techniques for data protection, including privacy-preserving deep learning techniques that can be adapted for scientific research, statistical disclosure control methods, and synthetic data generation. These approaches aim to balance analytic utility with privacy protection, allowing meaningful statistical analysis while minimizing re-identification risks. Research ethics committees and data protection officers increasingly require formal privacy impact assessments before approving studies involving sensitive personal data.
International Collaboration Challenges
Scientific research frequently transcends national boundaries, with international collaboration forming the backbone of many major research initiatives. GDPR's restrictions on data transfers to countries outside the European Economic Area create significant complications for such collaborations. When transferring data to countries without an adequacy decision from the European Commission, researchers must implement appropriate safeguards through mechanisms such as Standard Contractual Clauses or binding corporate rules.
These requirements have created new administrative burdens for international research consortia, necessitating complex data transfer agreements and compliance verification processes. Some non-EU research institutions have reported declining collaboration opportunities with European partners due to these complications. In fields requiring large, diverse datasets—such as genomics, epidemiology, or global climate research—these barriers can significantly impede scientific progress.
The challenges of international data transfers and standard contractual clauses have prompted the development of innovative solutions, including federated analysis approaches where algorithms travel to data rather than data traveling to researchers, thereby avoiding cross-border transfers entirely. Additionally, some international research organizations have established data processing hubs within the EU to facilitate compliance while maintaining global collaboration networks. Understanding GDPR's impact on international data transfers has become crucial for research organizations operating across borders.
GDPR's Impact on Statistical Analysis Methods
Beyond altering research design and data collection practices, GDPR has influenced the statistical methodologies employed in data analysis. Traditional statistical approaches often assumed relatively unrestricted access to raw data; now, analysts must adapt their methods to work with data that may be incomplete, aggregated, or transformed to protect privacy.
Differential privacy has emerged as a particularly important mathematical framework in this context. This approach adds calibrated noise to statistical outputs, providing formal privacy guarantees while preserving the validity of aggregate insights. Major research institutions and statistical agencies have begun implementing differential privacy into their analysis pipelines, though this adoption requires significant technical expertise and careful calibration to balance privacy protection with statistical utility.
Other methodological adaptations include federated learning systems that enable model training across decentralized data sources without sharing raw data, secure multi-party computation allowing analysis across separate datasets without revealing their contents, and privacy-aware machine learning algorithms designed to minimize exposure of sensitive information during model training. These exploring differential privacy approaches can be adapted from AI contexts to scientific research settings.
While these techniques allow research to continue under GDPR constraints, they often require more sophisticated computational resources and statistical expertise, potentially disadvantaging smaller research institutions with limited technical capabilities. This has led to calls for more accessible tools and frameworks that democratize privacy-preserving analysis methods.
Documentation and Accountability Requirements
GDPR's accountability principle requires organizations to demonstrate compliance through comprehensive documentation of their data processing activities. For researchers, this translates to maintaining detailed records of data collection, processing, sharing, and protection measures. These documentation requirements extend throughout the research lifecycle, from initial project planning through data collection, analysis, publication, and eventual archiving or destruction.
Research institutions have responded by developing standardized documentation frameworks, including data management plans, processing activity records, and impact assessments. While initially viewed primarily as administrative burdens, these documentation practices have yielded unexpected benefits by encouraging more thoughtful research design and promoting transparency about methodological choices. The accountability principle in GDPR has thus transformed from a compliance exercise into a driver of research quality.
Data Protection Impact Assessments (DPIAs) have become particularly important tools for high-risk research involving sensitive data categories or vulnerable populations. These structured risk assessments help researchers identify and mitigate potential privacy hazards before data collection begins. Demystifying DPIAs is essential for research organizations to effectively integrate these assessments into their research planning processes. Additionally, many research institutions have appointed dedicated Data Protection Officers who collaborate with ethics committees to ensure research protocols meet both ethical and legal requirements.
Subject Rights in Research Contexts
GDPR grants data subjects several rights regarding their personal information, including the right to access, rectification, erasure, and data portability. Implementing these rights in research contexts presents unique challenges, particularly when exercising certain rights might compromise study integrity or statistical validity. For example, selective withdrawal of participants could introduce bias into research findings if those withdrawing share particular characteristics.
Research exemptions under Article 89 allow for certain limitations on subject rights when necessary for research integrity, provided appropriate safeguards are implemented. However, these exemptions are not automatic and must be justified on a case-by-case basis. Researchers must carefully balance respect for individual autonomy with scientific objectives, developing protocols for responding to subject rights requests without compromising research validity.
Many research institutions have established dedicated processes for handling data subject access requests (DSARs) in research contexts, including procedures for providing participants with information about their data while protecting the confidentiality of analytical methods and other participants' information. Understanding the right to data portability and other subject rights has become essential for researchers working with personal data.
GDPR and Research Ethics: A Symbiotic Relationship
While initially perceived as potentially restrictive, GDPR has sparked renewed attention to fundamental research ethics principles that have long underpinned responsible science. The regulation's emphasis on transparency, purpose limitation, and data minimization aligns closely with ethical principles of respect for persons, beneficence, and justice that guide research ethics frameworks worldwide.
Research ethics committees have integrated GDPR considerations into their review processes, creating more comprehensive evaluation frameworks that consider both traditional ethical concerns and data protection requirements. This integration has fostered closer collaboration between data protection officers, ethics committees, and researchers, promoting a more holistic approach to responsible research design.
Rather than viewing GDPR simply as a compliance exercise, forward-thinking research institutions have embraced it as an opportunity to strengthen trust with research participants. By demonstrating robust data protection practices and respect for individual rights, researchers can build stronger relationships with study populations, potentially improving recruitment and retention—particularly in sensitive research areas. GDPR has thus reinforced the importance of addressing ethical considerations in AI deployment and research methodologies more broadly.