Financial Planning and Analysis with ChatGPT
Discover how AI and ChatGPT are revolutionizing financial planning and analysis. Learn practical use cases, implementation strategies, and how to gain competitive advantage in finance with AI tools in 2025.

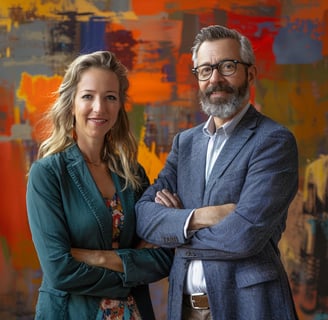
In the rapidly evolving financial landscape, the marriage of artificial intelligence and financial planning has emerged as a game-changing force that's reshaping how businesses forecast, analyze, and make critical financial decisions. Financial professionals who once spent countless hours poring over spreadsheets, creating manual forecasts, and generating reports are now leveraging sophisticated AI tools like ChatGPT to automate these processes and uncover insights that would otherwise remain hidden in vast oceans of financial data. The financial world has always been data-driven, but the sheer volume, velocity, and variety of data available today requires computational power and analytical capabilities that exceed human capacity alone. As organizations face increasing pressure to make faster, more accurate financial decisions in an uncertain global economy, ChatGPT and similar AI technologies are becoming essential components of the modern finance function. This article explores how ChatGPT is transforming financial planning and analysis (FP&A), showcasing real-world use cases that illustrate the revolutionary impact of AI on financial management and decision-making.
The Evolution of Financial Planning and Analysis
The journey of financial planning and analysis has been marked by continuous innovation, from the advent of electronic spreadsheets in the 1980s to today's sophisticated AI-powered systems. Traditional FP&A processes have long been characterized by labor-intensive data collection, manual spreadsheet manipulation, and backward-looking analyses that offered limited predictive value. Finance teams would spend up to 80% of their time collecting and validating data, leaving little bandwidth for the value-added analysis and strategic insights that business leaders desperately need. These conventional methods weren't just time-consuming; they were also prone to human error, inconsistency, and often failed to capture complex relationships between financial variables and external market factors.
The digital transformation wave that swept through finance departments in the early 2000s brought automation to many routine processes, with enterprise resource planning (ERP) systems and specialized FP&A software reducing the manual burden on finance teams. These technologies introduced standardized workflows, real-time data access, and improved reporting capabilities, setting the stage for more advanced analytical approaches. However, even these systems struggled with unstructured data, natural language processing, and the kind of contextual understanding required for truly adaptive financial planning in volatile markets.
The rise of artificial intelligence in financial services, particularly over the past five years, represents a quantum leap forward in capability, with AI-powered systems now able to process massive datasets, identify patterns invisible to human analysts, and generate insights with unprecedented speed and accuracy. According to a 2024 survey by Deloitte, 78% of financial executives report that AI has significantly improved their forecasting accuracy, with a median error reduction of 37% compared to traditional methods. The most sophisticated AI systems can now incorporate external data sources like economic indicators, industry trends, and even social media sentiment to create more holistic and forward-looking financial analyses.
Today's FP&A technologies, epitomized by large language models (LLMs) like ChatGPT, are characterized by their ability to understand and generate human language, interact conversationally with financial professionals, and continuously learn from new data and user interactions. These capabilities have fundamentally altered the role of finance teams, freeing analysts from data drudgery and enabling them to focus on strategic decision support, scenario planning, and discovering opportunities for growth and optimization. As we'll explore in the following sections, ChatGPT represents the convergence of multiple AI technologies that are particularly well-suited to the complex challenges of modern financial planning and analysis.
Understanding ChatGPT's Capabilities for Finance
ChatGPT's natural language processing capabilities make it uniquely positioned to transform financial planning and analysis through several key mechanisms that align with the specific needs of finance professionals. The model's ability to understand and generate human-like text allows finance teams to interact with complex financial data using conversational language rather than specialized query languages or complex formulas. This democratizes access to financial insights across organizations, enabling non-technical stakeholders to ask sophisticated questions about financial performance, forecasts, and scenarios without requiring technical intermediaries. Furthermore, ChatGPT can process and synthesize information from diverse financial documents, including annual reports, financial statements, market analyses, and regulatory filings, extracting relevant insights and presenting them in easily digestible formats.
The pattern recognition capabilities of ChatGPT enable it to identify trends, anomalies, and correlations in financial data that might escape human analysts. When trained on historical financial data, market conditions, and outcomes, the model can identify subtle relationships between variables and understand how different factors influence financial performance. These capabilities make ChatGPT particularly valuable for scenario planning and sensitivity analysis, allowing finance teams to rapidly model how changes in key variables might impact financial outcomes. For example, a finance team could ask ChatGPT to analyze how a 5% increase in raw material costs combined with a 3% decrease in consumer spending might affect quarterly profit margins across different product lines.
ChatGPT's predictive capabilities extend beyond simple trend extrapolation to encompass more sophisticated forecasting techniques that can incorporate multiple variables and conditions. The model can generate forward-looking financial projections based on historical performance, current market conditions, and strategic initiatives, providing finance teams with a range of potential outcomes and their associated probabilities. Perhaps most importantly, ChatGPT can explain its reasoning process, making its financial predictions more transparent and trustworthy than traditional "black box" AI models. This explainability is crucial in financial contexts where stakeholders need to understand not just what might happen, but why it might happen and what factors are driving the projections.
Despite these powerful capabilities, finance professionals must remain cognizant of ChatGPT's limitations in financial contexts. The model's knowledge has a training cutoff date, requiring supplementation with current financial data and market information for accurate analysis. While ChatGPT excels at identifying patterns and correlations in data, it doesn't inherently understand causation, which is crucial for accurate financial forecasting. Additionally, like all machine learning models, ChatGPT may inherit biases present in its training data, which could potentially skew financial analyses if not carefully monitored and corrected. These limitations underscore the importance of using ChatGPT as an augmentation tool for human financial expertise rather than a replacement for experienced financial professionals.
Key Use Cases of ChatGPT in Financial Planning
Budget Forecasting and Scenario Planning
Budget forecasting has been revolutionized by ChatGPT's ability to rapidly generate multiple scenario projections based on different assumptions and variables. Finance teams can now engage in conversational planning, asking the AI to model scenarios like "What happens to our Q3 cash flow if customer acquisition costs increase by 10% while sales grow by only 5%?" Financial analysts can iterate through dozens of scenarios in the time it would traditionally take to build a single forecast model, enabling more comprehensive risk assessment and strategic planning. The AI can incorporate historical performance data, current market trends, and even competitor movements to create nuanced forecasts that account for both internal and external factors.
Multi-dimensional analysis has become more accessible as ChatGPT can simultaneously consider numerous variables and their potential interactions. For instance, a retail company's finance team might ask ChatGPT to analyze how store location, seasonal factors, economic indicators, and marketing spend collectively impact sales performance, receiving natural language explanations of these complex relationships. This capability allows finance teams to move beyond simplistic "best case/worst case" scenario planning to develop much more nuanced views of possible futures with probabilistic assessments. According to a 2024 study by Gartner, organizations using AI for scenario planning consider 3.7 times more variables and develop 5 times more scenarios than those using traditional methods, resulting in 42% higher forecast accuracy.
Financial Reporting Automation
The automation of financial reporting has dramatically reduced the time finance teams spend on repetitive documentation tasks. ChatGPT can generate comprehensive financial narratives that explain performance trends, variances, and key metrics in clear, accessible language tailored to different stakeholders' needs. What once took finance teams days to compile can now be produced in minutes, with the AI drafting everything from management discussion and analysis (MD&A) sections to executive summaries and board presentations. This automation frees finance professionals to focus on strategic analysis and decision support rather than document preparation.
Anomaly detection and explanation represent another powerful application, as ChatGPT can identify unusual patterns in financial data and automatically generate plain-language explanations of potential causes. For example, if operating expenses spike unexpectedly in a particular department, ChatGPT can flag this anomaly, analyze potential causes based on available data, and generate an explanation for finance leaders to investigate further. This proactive approach to financial monitoring enables finance teams to address issues before they become significant problems. Moreover, ChatGPT can enhance regulatory compliance by drafting consistent, accurate disclosures that adhere to evolving financial reporting standards, reducing the risk of reporting errors and compliance violations.
Investment Analysis and Portfolio Management
Investment analysts are harnessing ChatGPT to process vast amounts of financial information, from earnings reports and analyst calls to market news and economic indicators. The AI can distill this information into actionable insights, helping analysts identify investment opportunities and risks that might otherwise be overlooked in the deluge of financial information. For example, ChatGPT can analyze earnings call transcripts from hundreds of companies, identifying patterns in executive language that might signal future performance issues or opportunities before they become apparent in financial results. This capability enables more informed investment decisions based on a broader range of information than human analysts could feasibly process alone.
Portfolio optimization has been enhanced by ChatGPT's ability to model complex relationships between different assets and market conditions. Financial advisors can use the technology to generate personalized investment recommendations based on clients' specific goals, risk tolerance, and market conditions. The AI can explain its recommendations in plain language, making complex investment strategies more accessible to clients and enhancing transparency. Additionally, ChatGPT can help investment teams conduct more thorough due diligence by rapidly analyzing company documentation, market position, competitive landscape, and regulatory issues, producing comprehensive assessment reports that highlight key risks and opportunities.
Risk Assessment and Management
Financial risk modeling has become more sophisticated as ChatGPT enables the integration of structured financial data with unstructured information like news events, regulatory changes, and geopolitical developments. This holistic approach to risk assessment provides finance teams with a more complete picture of potential threats to financial performance. For instance, an international company might use ChatGPT to assess how currency fluctuations, supply chain disruptions, and changing consumer preferences might collectively impact profitability across different markets. The model can generate comprehensive risk narratives that explain the interconnections between various risk factors and their potential financial implications.
Stress testing financial models against extreme but plausible scenarios has become more comprehensive with ChatGPT's ability to generate creative yet realistic stress scenarios based on historical events and emerging trends. Rather than testing against a limited set of predetermined scenarios, finance teams can now ask ChatGPT to generate dozens of potential stress scenarios that might affect their business, including combinations of events that might not have been historically observed but are theoretically possible. This approach to stress testing helps organizations prepare for a wider range of potential futures and build more resilient financial strategies.
Fraud Detection and Compliance
The fight against financial fraud has been strengthened by ChatGPT's ability to identify suspicious patterns and anomalies in transaction data that might indicate fraudulent activity. Unlike rule-based fraud detection systems that can be circumvented once their patterns are understood, ChatGPT can continuously adapt to new fraud techniques and identify subtle indicators that might escape traditional detection methods. For example, the AI might notice unusual patterns in the timing, frequency, or descriptions of transactions that collectively suggest potential fraud, even if each individual transaction appears legitimate when viewed in isolation. According to research from the Association of Certified Fraud Examiners, organizations using AI for fraud detection identify fraudulent activities 37% faster and reduce false positives by 63% compared to traditional methods.
Regulatory compliance monitoring has been transformed by ChatGPT's ability to track and interpret changing financial regulations across multiple jurisdictions. The AI can analyze regulatory publications, identify requirements relevant to specific business activities, and generate plain-language summaries of compliance obligations for finance teams. This capability is particularly valuable in the complex world of international finance, where organizations must navigate overlapping and sometimes contradictory regulatory requirements. ChatGPT can also assist in compliance documentation, drafting policies and procedures that align with current regulations and explaining complex compliance concepts in accessible language for staff training and implementation.
Implementation Strategies for Finance Teams
Integration with Existing Financial Systems
Successful implementation of ChatGPT in financial planning and analysis requires thoughtful integration with existing financial systems and data infrastructure. Organizations should begin by mapping their current financial data ecosystem, identifying key systems of record, data flows, and potential integration points for AI capabilities. API-based integrations offer the most flexible approach, enabling ChatGPT to access real-time financial data from ERP systems, financial planning software, CRM platforms, and market data services. This connected ecosystem allows the AI to incorporate the most current information into its analyses and recommendations, ensuring relevance and accuracy in a rapidly changing financial environment.
Data preparation and standardization are critical prerequisites for effective AI implementation, as ChatGPT requires consistent, clean data to generate reliable financial insights. Finance teams should establish data governance procedures that define data quality standards, ownership responsibilities, and validation processes specifically for AI-consumed data. Organizations may need to create intermediate data layers that transform raw financial data into formats more suitable for AI processing, such as tagged and structured financial statements or normalized transaction records. According to implementation experts at ChatGPT Consultancy, organizations that invest in proper data preparation before deploying AI solutions see 3.2 times higher success rates and achieve positive ROI 58% faster than those that rush implementation.
Data Security and Privacy Considerations
Financial data security represents one of the most significant considerations when implementing AI tools like ChatGPT in finance functions. Organizations must establish comprehensive security frameworks that protect sensitive financial information throughout the AI workflow, from data ingestion to insight generation and storage. Encryption for data in transit and at rest, strict access controls based on role and need-to-know principles, and regular security audits should be standard components of any AI implementation in finance. Organizations should also consider whether to implement on-premises or private cloud deployments for highly sensitive financial applications rather than using public API services.
Compliance with financial data regulations such as GDPR, CCPA, and industry-specific requirements needs to be engineered into AI implementations from the beginning rather than addressed as an afterthought. Finance teams should work closely with legal and compliance departments to ensure that AI use cases align with regulatory obligations and corporate data policies. This may include implementing data minimization principles (only processing the minimum necessary financial data for each use case), establishing data retention limits, and creating clear audit trails of how financial data is used by AI systems. Transparency with customers, investors, and regulators about how AI is being used in financial processes builds trust and ensures alignment with emerging regulatory expectations around AI governance.
Training Requirements for Finance Professionals
The successful deployment of ChatGPT in finance functions depends heavily on ensuring finance professionals have the skills and knowledge needed to effectively collaborate with AI systems. Organizations should develop role-specific AI training programs that address both technical and conceptual aspects of working with ChatGPT. For financial analysts, this might include training on prompt engineering (how to craft effective queries to get the best results from the AI), output evaluation (how to assess the quality and reliability of AI-generated financial analyses), and hybrid workflows that combine human expertise with AI capabilities. Financial leaders may need broader education on AI governance, ethics, and strategic applications to effectively oversee AI integration into finance operations.
Fostering an AI-augmented finance culture requires change management approaches that address both skills development and mindset shifts. Finance professionals may initially feel threatened by AI technologies or skeptical of their value, requiring clear communication about how these tools are intended to augment rather than replace human expertise. Organizations should showcase early wins and practical use cases that demonstrate tangible benefits for finance team members, such as elimination of tedious reporting tasks or enhanced analytical capabilities. Creating communities of practice where finance professionals can share experiences, techniques, and lessons learned about working with AI accelerates adoption and encourages innovation in how these tools are applied to financial challenges.
Statistics & Tables: The ROI of AI in Financial Planning
The financial impact of AI implementation in finance functions has been extensively documented across various industries and organization sizes. The following table presents a comprehensive overview of key metrics that demonstrate the return on investment for AI adoption in financial planning and analysis:
Conclusion
The integration of ChatGPT and similar AI technologies into financial planning and analysis represents a transformative shift that is redefining the capabilities, efficiency, and strategic value of finance functions. As we've explored throughout this article, these technologies are not merely automating existing processes but enabling entirely new approaches to financial forecasting, reporting, investment analysis, risk management, and compliance. The ability to process vast amounts of structured and unstructured financial data, identify complex patterns, and generate natural language insights is fundamentally changing how financial decisions are made and how finance teams support broader business strategy.
Organizations that successfully implement AI-powered financial planning gain significant competitive advantages through enhanced forecasting accuracy, faster financial close processes, more comprehensive risk assessments, and the ability to rapidly model complex scenarios. These capabilities enable more agile financial management in uncertain economic environments and allow finance teams to spend less time on routine data processing and more time on strategic analysis and business partnership. As highlighted in our analysis of ROI metrics, the financial benefits of these implementations typically far outweigh the initial investment costs, with most organizations achieving positive returns within 12-18 months of deployment.
Looking forward, the finance profession itself is evolving in response to these technological capabilities, with increasing emphasis on data science skills, AI literacy, and strategic thinking among finance professionals. Rather than diminishing the importance of human expertise, AI tools like ChatGPT are elevating the role of finance teams by augmenting their capabilities and freeing them from routine tasks. The most successful finance organizations will be those that embrace this technological evolution while developing their human talent to work effectively alongside these powerful new tools, creating a symbiotic relationship between human judgment and artificial intelligence.
Frequently Asked Questions
How does ChatGPT improve financial forecasting accuracy?
ChatGPT enhances forecasting accuracy by analyzing historical financial data alongside external factors like market trends, economic indicators, and industry developments. The AI can identify complex patterns and relationships that traditional forecasting methods might miss. Studies show that AI-augmented forecasting reduces median error rates by 30-40% compared to traditional methods, particularly for volatile markets and complex product portfolios.
What financial data security measures should be in place when implementing ChatGPT?
Organizations should implement end-to-end encryption, strict access controls, data anonymization where appropriate, and comprehensive audit trails. Consider private cloud or on-premises deployments for sensitive financial applications rather than public API services. Regular security assessments and compliance reviews are essential, especially for organizations handling regulated financial information.
How can finance teams validate the accuracy of ChatGPT's financial analyses?
Finance teams should establish validation frameworks that compare AI-generated insights against known historical outcomes, expert judgment, and alternative analytical methods. Implementing "human-in-the-loop" processes for critical financial analyses ensures appropriate oversight. Organizations should also maintain transparency about the data sources, assumptions, and methodologies used by the AI to facilitate effective validation.
What skills do finance professionals need to effectively work with ChatGPT?
Finance professionals increasingly need a blend of traditional financial expertise with data literacy, basic understanding of AI capabilities and limitations, effective prompt engineering skills, and critical evaluation of AI outputs. Strategic thinking and business partnering abilities become more important as routine analytical tasks are automated. Many organizations are investing in training programs that develop these hybrid skill sets.
How does ChatGPT handle financial data privacy regulations?
ChatGPT implementations must be configured to comply with relevant regulations like GDPR, CCPA, and industry-specific requirements. This typically involves data minimization (only processing necessary information), clear consent mechanisms, defined retention periods, and documented data processing activities. Organizations should work with legal and compliance teams to ensure AI use cases align with regulatory obligations.
Can ChatGPT help with regulatory compliance and financial reporting?
Yes, ChatGPT can assist with regulatory compliance by monitoring changing regulations, generating compliant disclosure language, and flagging potential compliance issues in financial data. For financial reporting, the AI can automate narrative generation, explain variances, and ensure consistency across reporting periods, significantly reducing the time finance teams spend on documentation.
What's the typical implementation timeline for ChatGPT in financial planning?
Implementation timelines vary based on organizational complexity and use case sophistication, but typically range from 3-6 months for initial deployment and up to 12-18 months for full integration across multiple finance functions. Organizations often start with focused use cases to demonstrate value before expanding to enterprise-wide implementations.
How does ChatGPT integrate with existing financial planning systems?
ChatGPT typically integrates through APIs with ERP systems, financial planning software, data warehouses, and market data services. Some organizations develop custom middleware that transforms financial data into formats suitable for AI processing. Cloud-based financial systems generally offer the most straightforward integration paths through established connectors and data pipelines.
What are the limitations of using ChatGPT for financial analysis?
ChatGPT may have limitations in understanding company-specific financial terminology or processes without additional training. The model's knowledge cutoff date means it requires supplementation with current financial data. It excels at identifying correlations but may not inherently understand causation in financial contexts. Organizations should view ChatGPT as an augmentation tool for human expertise rather than a replacement.
How are leading financial institutions measuring ROI from ChatGPT implementations?
Leading institutions measure ROI through metrics including time saved on routine financial processes, improved forecast accuracy, faster financial close cycles, reduced compliance incidents, and enhanced decision quality. Some organizations also track employee satisfaction improvements as finance professionals shift from data processing to more strategic work. Comprehensive ROI frameworks typically consider both tangible cost savings and strategic value creation.
Additional Resources
The Rise of AI and ChatGPT for Finance Leaders - A comprehensive guide for finance executives on strategic AI implementation.
Using ChatGPT for Real-Time Fraud Detection in Finance - Detailed exploration of AI-powered fraud prevention strategies.
ChatGPT Business Intelligence Strategy - Framework for integrating ChatGPT into broader business intelligence initiatives.
AI Strategy and Roadmap for Finance Organizations - Step-by-step guidance for developing comprehensive AI strategies.
Financial Fraud Detector with GPT - Technical implementation guide for fraud detection systems.