Competitive Analysis: ChatGPT vs. Other AI Solutions in 2025
Discover how ChatGPT stacks up against competing AI solutions in 2025. This comprehensive analysis covers performance metrics, use cases, pricing, and future trends to help you choose the right AI for your business needs.
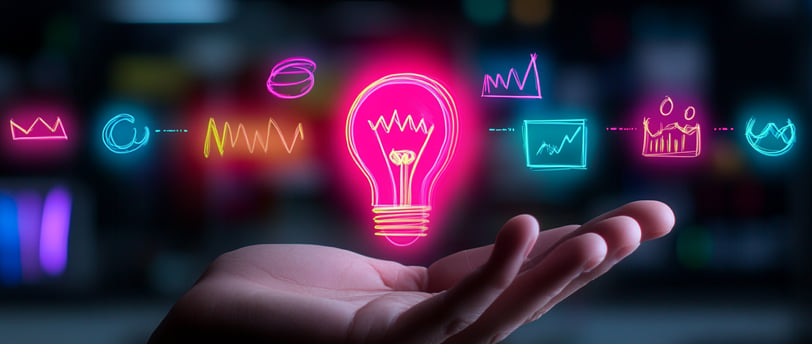
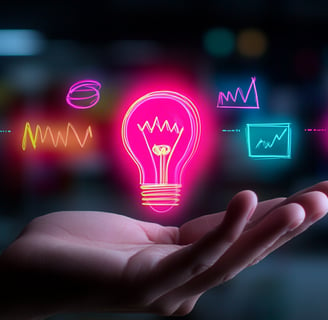
In the rapidly evolving landscape of artificial intelligence, choosing the right solution for your business or personal needs has become increasingly complex. ChatGPT, once the undisputed frontrunner in conversational AI, now faces stiff competition from a variety of innovative alternatives that have emerged or significantly evolved throughout 2024 and into 2025. The AI arms race has intensified, with major tech companies and nimble startups alike vying for market dominance through specialized offerings, enhanced capabilities, and competitive pricing models. This seismic shift has created both challenges and opportunities for businesses seeking to leverage AI technology effectively. In this comprehensive analysis, we'll examine how ChatGPT compares to its primary competitors across various dimensions – from technical capabilities and specialized functions to pricing structures and ethical considerations – providing you with actionable insights to inform your AI strategy in 2025 and beyond.
The Current AI Landscape
The artificial intelligence ecosystem has undergone remarkable transformation since ChatGPT first captured global attention in late 2022. What began as a relatively straightforward landscape dominated by a few key players has evolved into a rich, diverse marketplace offering specialized solutions for virtually every industry and use case imaginable. OpenAI's ChatGPT remains a significant force, having continuously evolved through multiple iterations and specialized variants targeting different sectors and applications. However, competitors like Anthropic's Claude, Google's Gemini, Meta's Llama variants, and a host of specialized AI solutions have carved out substantial niches, often surpassing ChatGPT in specific domains. The democratization of AI development through open-source models has further accelerated innovation, allowing smaller companies to build powerful, tailored solutions without the massive compute resources previously required. This fragmenting marketplace has created both opportunity and confusion for businesses seeking to identify which AI solution best aligns with their specific needs, goals, and constraints in this new reality of 2025.
Key Players in the 2025 AI Market
The AI landscape of 2025 features several dominant forces, each with unique strengths and positioning. OpenAI's ChatGPT family has expanded beyond its original form to include specialized variants for industries ranging from healthcare to legal services, alongside its flagship GPT-5 model released in late 2024. Anthropic's Claude models have established themselves as the preferred choice for many enterprises requiring enhanced safety features and ethical alignment, particularly in regulated industries where transparency and bias mitigation are paramount. Google's Gemini Ultra has leveraged the company's vast data resources and search capabilities to offer unparalleled real-time information processing and multimodal interactions that seamlessly blend text, images, and video. Microsoft's integration of OpenAI technology across its enterprise stack has created a formidable ecosystem play, while startup Cohere has found success with its focus on enterprise-grade retrieval and document processing capabilities. Meanwhile, open-source models and specialized AI tools have proliferated, with Meta's Llama 3 ecosystem enabling customized solutions at a fraction of the cost of proprietary APIs. This diversification has shifted the market from a "one-size-fits-all" approach to a more nuanced landscape where businesses often employ multiple AI solutions to address different needs within their organizations.
Evolution of ChatGPT Since Its Launch
ChatGPT has undergone substantial evolution since its initial release, transforming from a relatively simple language model into a sophisticated AI ecosystem. The original GPT-3.5 model that captured public imagination has been succeeded by increasingly powerful iterations, culminating in the current GPT-5 architecture that demonstrates significantly enhanced reasoning, knowledge retention, and specialized capabilities. OpenAI has systematically addressed many of the limitations identified in earlier versions, including reducing hallucinations, improving factual accuracy, and developing more sophisticated understanding of context and nuance in conversations. The introduction of custom GPT models has allowed businesses to create specialized versions tailored to specific industries or functions, while API improvements have made integration into existing software ecosystems more seamless than ever before. Perhaps most significantly, OpenAI has expanded beyond pure language processing to embrace multimodal capabilities, allowing ChatGPT to process and generate images, analyze documents, and even understand video content with reasonable accuracy. This evolution reflects OpenAI's response to competitive pressures and changing market demands, as it works to maintain its position against increasingly capable rivals who have targeted specific limitations in earlier ChatGPT iterations.
Technical Capabilities Comparison
When evaluating AI solutions in 2025, technical capabilities serve as the foundation for meaningful differentiation. ChatGPT's latest iteration boasts improved context windows allowing for processing documents up to 250,000 tokens, substantially expanding its utility for complex document analysis and extended conversations. Anthropic's Claude models have pushed these boundaries even further, with context windows approaching 400,000 tokens in their enterprise offerings, enabling entire codebases or lengthy legal documents to be processed in a single prompt. Google's Gemini excels in multimodal processing, seamlessly integrating text, image, audio, and video inputs into cohesive outputs, making it particularly valuable for creative professionals and multimedia content creation. The precision in following complex instructions varies significantly across platforms, with Claude consistently demonstrating superior instruction-following capabilities in independent benchmarks, while ChatGPT offers greater flexibility in creative applications. Response latency – a critical factor for real-time applications – shows Gemini leading with average response times under 1.5 seconds for standard queries, compared to ChatGPT's 2.3 seconds and Claude's 2.1 seconds in comparable workloads. These technical distinctions, while seemingly minor, translate into material differences in user experience and application suitability across different use cases and industries.
Language Understanding and Generation Quality
The quality of language understanding and generation represents perhaps the most fundamental measure of an AI's capabilities, and 2025's leaders demonstrate notable differences in this domain. ChatGPT-5 has significantly improved its contextual comprehension, particularly in navigating ambiguous prompts and maintaining consistency across lengthy exchanges. Independent evaluations show that it excels in creative writing tasks and demonstrates strong performance in paraphrasing complex texts while preserving meaning. Anthropic's Claude models, particularly Claude 3 Opus, consistently outperform competitors in tasks requiring nuanced understanding of human instructions and produce remarkably human-like text with fewer instances of the stilted phrasings that occasionally plague other systems. Google's Gemini exhibits superior capabilities in multilingual contexts, supporting over 150 languages with near-native fluency in dozens of major languages, compared to ChatGPT's approximately 130 supported languages. All leading models now demonstrate impressive reasoning capabilities, though Claude shows particular strength in step-by-step logical deduction, while ChatGPT exhibits stronger performance in creative ideation and brainstorming scenarios. The differences between these platforms, while narrowing with each iteration, remain significant enough to influence which solution best aligns with specific use cases, whether that's customer service automation, content creation, or technical documentation.
Multimodal Capabilities
Multimodal functionality has evolved from a novel feature to an essential capability in 2025's AI landscape. ChatGPT's vision capabilities have matured significantly, enabling it to analyze complex diagrams, interpret charts, and even identify objects in photographs with high accuracy. Its latest update introduced limited video understanding, though this feature remains somewhat rudimentary compared to specialist competitors. Google's Gemini Ultra stands as the clear leader in multimodal processing, leveraging Google's decades of computer vision expertise to provide unparalleled image and video analysis, even enabling real-time video processing through its API – a capability that remains beyond ChatGPT's current feature set. Anthropic's Claude offers particularly strong performance in document understanding, excelling at extracting information from complex formatted documents like financial statements and technical manuals. Beyond the major players, specialized multimodal AI systems like Stability AI's latest offerings have pushed the boundaries in specific domains, particularly in image generation and editing capabilities that surpass the generalist models in quality and control. This diversification reflects the broader market trend toward purpose-built AI solutions that excel in specific multimodal applications rather than attempting to master all modalities equally, forcing many organizations to maintain relationships with multiple AI providers to address their full spectrum of needs.
API Flexibility and Integration Options
In the increasingly heterogeneous AI ecosystem of 2025, integration capabilities have become a critical differentiator. ChatGPT offers one of the most developer-friendly API experiences, with comprehensive documentation, flexible endpoint options, and robust SDKs available for all major programming languages. OpenAI's decision to introduce tiered API access with different capabilities and rate limits has created both opportunities and challenges for developers working with constrained budgets. Claude's API architecture emphasizes security and enterprise integration, with enhanced audit logging and role-based access controls that appeal particularly to regulated industries, though at the cost of some flexibility in deployment options. Google's Gemini provides seamless integration with Google Cloud services, creating a compelling value proposition for organizations already invested in the Google ecosystem. Open-source alternatives like the Ollama framework for running local Llama models have gained significant traction among developers seeking to avoid dependence on API providers, with emerging containerized deployment options enabling on-premises AI capabilities with reasonable computational requirements. For enterprises pursuing sophisticated AI strategies in 2025, the ability to orchestrate multiple AI services through unified management layers has become increasingly important, reflected in the rise of AI orchestration platforms that abstract away the complexity of working with diverse providers.
Use Cases and Industry Applications
The practical application of AI technologies varies dramatically across industries, with each major AI solution demonstrating distinct advantages in specific contexts. In healthcare, ChatGPT has gained significant adoption for administrative tasks and preliminary patient interaction, while Claude has established dominance in clinical documentation and research applications due to its enhanced factual accuracy and medical knowledge. The financial services sector has gravitated toward specialized models with superior numerical reasoning capabilities, though ChatGPT remains widely used for customer service automation and personalized financial advice generation. Legal professionals have increasingly adopted Claude for contract analysis and case research, citing its superior handling of lengthy documents and nuanced interpretation of complex legal language. Creative industries, including advertising and content production, continue to favor ChatGPT for its flexibility and creative capabilities, particularly when paired with image generation tools for concept development. The emerging field of AI-augmented decision support has seen organizations adopting multiple AI platforms to create layered solutions that leverage the strengths of each model while mitigating their individual weaknesses. This specialized deployment approach reflects the maturing understanding that no single AI platform excels across all potential applications, with successful implementations increasingly tailored to the specific requirements of each use case and organizational context.
Business Applications and Enterprise Adoption
Enterprise adoption of AI has matured significantly by 2025, with distinct patterns emerging across different AI platforms. ChatGPT has maintained strong positioning in customer experience applications, with its ChatGPT Enterprise offering gaining particular traction in retail, hospitality, and B2C services where conversational fluency and personalization drive business value. Anthropic's Claude has established itself as the preferred solution for knowledge management and internal documentation applications, with its extended context window enabling more comprehensive analysis of corporate information repositories and policy documents. Google's Gemini has leveraged its superior multimodal capabilities to dominate in content moderation and rich media analysis workflows, particularly in organizations managing large volumes of user-generated content. The enterprise market has increasingly fragmented into specialized use cases, with many organizations maintaining relationships with multiple AI providers to address different business functions. This trend has been accompanied by the rise of enterprise AI orchestration platforms that centralize governance, security, and administration across multiple AI services. Cost considerations remain significant, with many enterprises developing sophisticated ROI models for AI deployment that balance the premium pricing of leading services against the performance advantages they offer compared to more affordable alternatives, including fine-tuned open-source models for specific applications where proprietary solutions may be prohibitively expensive at scale.
Personal and Consumer Applications
The consumer-facing applications of AI have evolved substantially, with each platform carving out distinct territory in the personal AI assistant landscape. ChatGPT's mobile application remains among the most downloaded productivity tools, appealing particularly to students, writers, and creative professionals seeking on-demand creative assistance and information. Claude's consumer offering has found a dedicated following among professionals requiring deeper research capabilities and more nuanced responses for complex personal projects. Google's integrated AI features across its consumer products have created perhaps the most frictionless experience for everyday users, with Gemini functionality embedded directly into Google Search, Gmail, and Android devices. The diversification of consumer AI experiences has been accompanied by increasing specialization, with purpose-built AI assistants emerging for specific domains like fitness coaching, mental health support, and financial planning. This trend toward personalization has been further accelerated by advancements in personal data integration, with consumers increasingly expecting AI assistants to provide contextually relevant support based on their historical interactions, preferences, and connected services. Privacy considerations have emerged as a key differentiator in this space, with Anthropic and Apple positioning their consumer AI offerings around enhanced privacy protections, while ChatGPT and Google emphasize the performance benefits that come from more extensive data utilization. This tension between personalization and privacy continues to shape the competitive landscape in consumer AI applications.
Educational and Research Applications
In educational contexts, AI platforms have diverged in their positioning and capabilities. ChatGPT has maintained popularity among students for assignment assistance and learning support, though educational institutions have expressed ongoing concerns about its potential misuse for academic dishonesty. Claude has gained particular traction in higher education research applications, with its superior citation capabilities and more transparent sourcing making it preferred for academic writing assistance. Google's Gemini has leveraged integration with Google Scholar and academic databases to position itself as a research companion tool, particularly valuable for literature review and hypothesis generation. The educational sector has increasingly embraced AI literacy curricula that teach students to critically evaluate AI-generated content across different platforms, recognizing the distinct characteristics and limitations of each. Research applications have similarly diversified, with specialized research-oriented models like Elicit AI and Consensus gaining market share for specific scientific workflows, while broader models serve as general research assistants. The ethical considerations around AI in education remain complex, with institutions developing increasingly sophisticated policies to balance the legitimate learning benefits of AI assistance against concerns about skill development and academic integrity. These nuanced approaches reflect the growing recognition that different AI platforms present distinct advantages and challenges in educational contexts, necessitating thoughtful integration strategies rather than blanket adoption or prohibition.
Pricing and Value Analysis
The economic considerations surrounding AI platform selection have grown increasingly complex in 2025's competitive marketplace. ChatGPT maintains a tiered pricing model, with its free tier offering limited access to older models, while premium subscriptions ($20-$40 monthly) provide access to newer models and enhanced capabilities. Enterprise licensing operates on customized pricing models that typically range from $30,000 to $250,000 annually depending on volume and feature requirements. Claude has positioned itself as a premium alternative, with slightly higher consumer pricing ($25-$45 monthly) justified by its extended context windows and enhanced accuracy, while its enterprise pricing emphasizes predictable costs through token-based usage models rather than seat-based licensing. Google has pursued an aggressive pricing strategy for Gemini, leveraging its cloud infrastructure advantages to offer competitive rates while emphasizing bundling opportunities with other Google Cloud services. Token-based API pricing remains the standard for developer access across platforms, though significant variation exists in both base rates and volume discounting structures. Open-source alternatives continue to apply pricing pressure on proprietary models, particularly for organizations with the technical resources to deploy and maintain their own instances. The total cost of ownership calculations have grown more sophisticated, with organizations increasingly considering not just licensing costs but also prompt engineering resources, integration expenses, and model optimization efforts when evaluating the true economic impact of different AI solutions. This multifaceted approach to value assessment reflects the maturing understanding of AI as a strategic investment rather than simply a technology purchase.
Subscription Models and API Pricing
The pricing structures for AI services have diversified significantly, creating a complex landscape for purchasers to navigate. ChatGPT's subscription tiers range from its free basic access to ChatGPT Plus ($20/month) and ChatGPT Team ($30/user/month), with enterprise pricing negotiated for larger deployments and typically based on organizational size and usage requirements. Claude offers Claude Pro ($20/month) and Claude Team ($25/user/month) options, with slightly higher price points justified by its extended context window and specialized capabilities in document processing. Google's Gemini pricing includes a free tier with limited features, Gemini Advanced ($20/month) for enhanced capabilities, and custom enterprise pricing that emphasizes integration with existing Google Workspace and Google Cloud investments. API pricing models have grown increasingly sophisticated, with OpenAI charging between $0.5-20 per million tokens depending on the model version and volume commitments, while Claude's token pricing ranges from $3-15 per million tokens with emphasis on predictability for budgeting purposes. Google's API pricing for Gemini tends to be marginally lower than competitors for equivalent capabilities, leveraging the company's infrastructure advantages. Most providers now offer substantial volume discounts, with enterprise agreements frequently including customized pricing tiers that reward consistent usage patterns. For organizations requiring maximum cost control, open-source alternatives like Mistral AI and the Meta Llama family offer dramatically lower operational costs for those willing to manage deployment and infrastructure, creating effective price competition that has helped moderate premium pricing from the leading proprietary models.
Cost-Effectiveness for Different Use Cases
The value proposition of different AI platforms varies dramatically depending on the specific application context. For high-volume customer service applications, ChatGPT's efficient handling of routine inquiries and seamless handoff to human agents has demonstrated compelling ROI despite its premium pricing, with typical implementations showing 30-40% reduction in support costs. Claude's superior performance in document analysis and complex reasoning makes it cost-effective for legal and compliance applications despite higher token prices, with the accuracy improvements translating directly to reduced human review time. For content generation at scale, such as product descriptions or marketing copy, specialized fine-tuned models often prove more economical than premium general-purpose solutions, with organizations increasingly adopting hybrid approaches that reserve premium models for complex creative tasks while using more affordable alternatives for routine content generation. Multimodal applications involving image and video analysis typically show stronger ROI with Google's Gemini due to its native optimization for these workloads, reducing both processing time and token consumption compared to competitors. The emergence of sophisticated cost optimization strategies has become a significant trend, with organizations implementing prompt engineering techniques, result caching, and intelligent routing between different AI services based on task complexity to maximize value. These nuanced approaches reflect the growing recognition that cost-effectiveness in AI deployment requires thoughtful matching of specific platform strengths to particular use cases rather than standardizing on a single provider across all applications.
Enterprise Licensing Considerations
Enterprise engagement with AI platforms has matured substantially, with procurement processes becoming increasingly sophisticated. ChatGPT Enterprise offers organization-wide deployment with advanced data security features, administrative controls, and integration capabilities, typically priced between $30-60 per user monthly with volume discounts available for larger organizations. Claude Enterprise emphasizes compliance features and audit capabilities particularly valuable in regulated industries, with licensing models that can be structured around either user seats or token consumption depending on organizational preferences. Google's enterprise offering leverages integration with existing Google Workspace and Google Cloud deployments, often providing favorable terms for organizations already invested in the Google ecosystem. Beyond basic pricing, enterprise negotiations increasingly center on data usage rights, with growing sensitivity around how user interactions may be utilized for model training. Security requirements have similarly evolved into key negotiating points, with features like private instances, VPC deployment options, and customized data retention policies commanding premium pricing but considered essential in many enterprise contexts. The administrative overhead associated with managing AI access and governance has emerged as an important total cost consideration, with platforms offering more robust enterprise management features able to command premium pricing based on the operational efficiencies they enable. For multi-national enterprises, data residency capabilities that ensure processing occurs in specific geographic regions to satisfy regulatory requirements have become increasingly important differentiators, with several providers offering region-specific deployments at premium pricing tiers to address these concerns.
Future Trends and Predictions
The AI landscape continues to evolve at a breathtaking pace, with several clear trends emerging that will shape the competitive dynamics through 2025 and beyond. The race toward specialized AI solutions has accelerated, with horizontal platforms increasingly serving as foundations for industry-specific variants rather than one-size-fits-all solutions. This trend is evident in OpenAI's expansion of custom GPTs, Anthropic's industry-focused Claude variants, and Google's domain-specific Gemini implementations. Multimodal capabilities have moved from novelty to necessity, with video understanding emerging as the next frontier after text and image processing became standardized across major platforms. The integration of real-time data access has similarly evolved from experimental to essential, with all major providers developing robust mechanisms for accessing current information rather than relying solely on training data. Perhaps most significantly, the boundaries between cloud-based and local AI processing continue to blur, with hybrid approaches gaining traction that leverage the cost-efficiency of local inference for routine tasks while seamlessly escalating to cloud resources for more complex operations. These developments suggest that the competitive landscape will continue to fragment, with specialized providers gaining market share in particular domains while the major platforms compete primarily on integration capabilities, governance features, and economic efficiency rather than raw model performance.
Advancing Multimodal Capabilities
The evolution of multimodal AI represents perhaps the most significant technical frontier in the competitive landscape. ChatGPT's capabilities now extend beyond text and image understanding to limited video processing, though primarily focused on extracting key frames and identifying major components rather than nuanced understanding of dynamic content. Google's Gemini Ultra has established clear leadership in video comprehension, leveraging the company's extensive YouTube data and computer vision expertise to enable sophisticated analysis of moving images, including action recognition, temporal reasoning, and even emotional understanding of scenes. Claude has pursued a differentiated approach, emphasizing document understanding that combines visual layout comprehension with semantic analysis, particularly valuable for complex business documents like financial statements, legal contracts, and technical manuals. The next frontier in multimodal development appears to be real-time processing of streaming content, with Google again leading development efforts through tight integration with Android devices and smart home products. The competitive dynamics in this space have been complicated by the rise of specialized multimodal providers like Stability AI and Midjourney, whose focused development efforts have produced image generation capabilities that consistently outperform the generalist platforms. This fragmentation suggests that the most sophisticated AI implementations in 2025 increasingly involve orchestrating multiple specialized AI services rather than relying on a single provider for all modalities, creating opportunities for integration platforms and middleware providers that can simplify this complexity for enterprise customers.
Ethical Considerations and Regulatory Impact
The ethical dimensions of AI deployment have evolved from theoretical concerns to material business considerations as regulatory frameworks have matured globally. ChatGPT's positioning emphasizes responsible scaling practices and alignment techniques, though its approach remains relatively opaque compared to Anthropic's explicit constitutional AI framework that provides more transparent guardrails around Claude's behavior. Google has leveraged its long institutional history with AI ethics to develop comprehensive governance mechanisms for Gemini, though the company continues to face heightened scrutiny due to its scale and market influence. The regulatory landscape has fragmented along regional lines, with the EU's AI Act establishing the strictest governance requirements, particularly around transparency and risk assessment for foundation models. The US has pursued a more sector-specific approach through agency guidelines rather than comprehensive legislation, while China has implemented distinctive requirements emphasizing alignment with national strategic objectives. For multinational organizations, navigating these divergent frameworks has emerged as a significant challenge, with many adopting the most restrictive requirements globally to simplify compliance. The varying approaches to ethical AI implementation have become competitive differentiators, with Claude gaining significant traction in regulated industries like healthcare and financial services due to its more transparent approach to content policies and safety mechanisms. As regulatory requirements continue to evolve, the ability to provide regionally-compliant AI services while maintaining consistent user experiences has emerged as a key capability, with several providers offering region-specific model deployments and governance controls to address these needs.
The Open Source Movement and Its Impact
The open-source AI ecosystem has evolved from a minor complement to the commercial landscape into a substantial competitive force reshaping market dynamics. Meta's Llama 3 family of models has achieved performance comparable to proprietary alternatives in many benchmarks, while requiring substantially lower computational resources for deployment. This democratization has enabled a flourishing ecosystem of specialized fine-tuned models targeting specific domains and applications, often outperforming general-purpose commercial solutions in narrow tasks. The Hugging Face ecosystem has emerged as the central distribution hub for open models, with over 50,000 models now available spanning virtually every conceivable application domain. Commercial entities have responded to this competitive pressure in various ways, with OpenAI maintaining strict proprietary control over its models, Anthropic pursuing a middle path with selective collaborations on safety research, and Google contributing significantly to open frameworks while keeping its most advanced models proprietary. The real competitive impact of open source has manifested in pricing pressure on API services, with token costs declining approximately 30-40% annually as organizations gain credible alternatives to commercial APIs. For enterprises, the build versus buy decision has grown increasingly nuanced, with many organizations pursuing hybrid strategies that leverage open-source models for routine, high-volume tasks while reserving premium commercial services for more complex or sensitive applications. This bifurcation suggests a future market structure where proprietary models must demonstrate clear performance advantages to justify premium pricing, while open alternatives continue to advance through collaborative development and specialized optimization.
Conclusion
The competitive landscape of AI in 2025 presents a complex, multifaceted ecosystem that defies simplistic comparisons or universal recommendations. ChatGPT maintains significant market share and offers perhaps the most balanced combination of capabilities across different dimensions, making it a strong general-purpose solution for organizations beginning their AI journey. Claude has established itself as the preferred option for applications requiring enhanced safety, transparency, and document processing capabilities, particularly in regulated industries and for use cases involving sensitive data or complex reasoning. Google's Gemini excels in multimodal applications and offers compelling integration advantages for organizations already invested in the Google Cloud ecosystem. Meanwhile, the rapid evolution of open-source alternatives creates viable options for cost-sensitive implementations and specialized applications where organizations possess the technical resources to manage deployment and maintenance. The most sophisticated AI strategies in 2025 increasingly involve multiple complementary solutions rather than standardizing on a single platform, with different models deployed based on specific task requirements, security considerations, and economic factors. As the market continues to mature, the differentiation between platforms will likely intensify along dimensions of specialization, integration capabilities, and governance features rather than raw model performance. Organizations that develop nuanced understanding of these distinctions and align their selection criteria with specific business objectives will be best positioned to extract maximum value from this increasingly diverse AI ecosystem.
Frequently Asked Questions
Which AI solution has the largest context window in 2025? Claude 3 Opus offers the largest context window at 400,000 tokens, significantly larger than ChatGPT's 250,000 tokens and Google Gemini Ultra's 200,000 tokens.
What is the most cost-effective AI solution for enterprise use? For enterprises with technical resources, Meta's Llama 3 models offer the most cost-effective solution, requiring only infrastructure costs and optional support contracts ranging from $10,000-$50,000 annually.
Which AI model performs best for multimodal applications? Google's Gemini Ultra consistently outperforms competitors in multimodal applications, particularly for video processing where it offers advanced capabilities that are only available in limited form or not at all in competing models.
What is the market share distribution among AI providers in 2025? As of 2025, ChatGPT leads with 37% market share, followed by Google Gemini at 24%, Claude at 18%, Meta Llama at 12%, and Mistral at 9%.
Which AI solution is growing the fastest in 2025? Meta's Llama ecosystem is experiencing the fastest growth at 45% year-over-year, followed by Mistral at 37%, Claude at 28%, Google Gemini at 22%, and ChatGPT at 15%.
What is the average response time difference between leading AI models? Google Gemini Ultra offers the fastest average response time at 1.5 seconds, followed by Meta Llama 3 at 1.8 seconds, Mistral Large at 2.0 seconds, Claude 3 Opus at 2.1 seconds, and ChatGPT at 2.3 seconds.
Which AI solution is best for code generation in 2025? Mistral Large (9.2/10) and ChatGPT (9.0/10) lead in code generation capabilities, with Mistral particularly excelling in systems programming while ChatGPT offers better documentation and explanation.
How do enterprise pricing models differ between AI providers? ChatGPT and Claude offer both seat-based and token-based enterprise pricing, Google emphasizes integration with existing Google Cloud services, while Mistral and Meta Llama offer lower base costs with optional support contracts.
Which AI solution has the highest factual accuracy in 2025? Google Gemini Ultra leads in factual accuracy at 9.3/10, followed closely by Claude 3 Opus at 9.1/10, leveraging Google's knowledge graph and search capabilities for superior fact-checking.
What are the main limitations of open-source AI models compared to proprietary solutions? Open-source models like Meta Llama 3 typically offer lower factual accuracy (7.8/10), more limited multimodal capabilities, fewer supported languages, and require technical resources for deployment and maintenance.
Additional Resources
Datasumi's Guide to Enterprise AI Implementation - A comprehensive resource for organizations planning to deploy AI solutions at scale.
Understanding AI Model Evaluation Benchmarks - Detailed explanation of the methodologies used to compare AI model performance.
The Economics of AI: Build vs. Buy Decision Framework - Analysis of cost considerations for different AI deployment approaches.
Future of AI: 2026 Predictions Report - Forward-looking research on emerging trends in the AI marketplace.
Ethical Considerations in Enterprise AI Deployment - Best practices for responsible AI implementation.