ChatGPT's Operator AI Agents Revolutionising Business Processes
Discover how OpenAI's Operator AI is transforming business workflows through autonomous agents that streamline operations, reduce costs, and drive innovation across industries. Learn implementation strategies, benefits, and real-world applications.
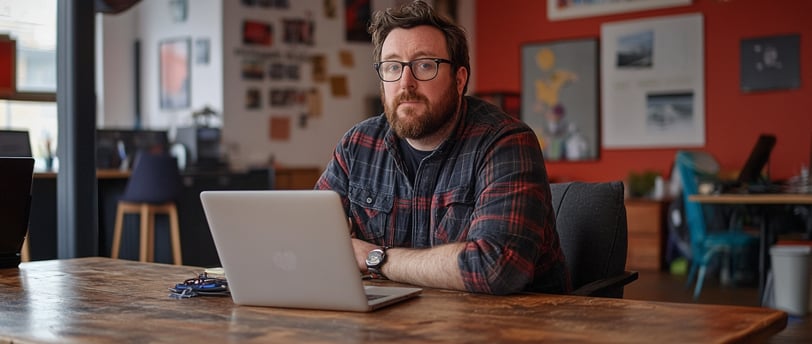
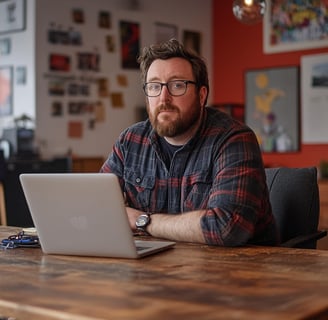
Imagine arriving at work to find your morning reports already compiled, your emails categorized and prioritized, your calendar optimized, and your routine data entry tasks completed—all while you were sleeping. This isn't science fiction; it's the practical reality being created by ChatGPT's Operator AI. The business world stands at the threshold of a productivity revolution powered by autonomous agents capable of performing complex tasks with minimal human supervision. In this comprehensive exploration, we'll dive into how Operator AI is fundamentally changing business processes, examine real-world implementations, and provide actionable insights for organizations looking to harness this transformative technology.
As artificial intelligence continues its rapid evolution, OpenAI's Operator represents a significant leap forward in creating AI systems that can truly operate independently within defined parameters. Unlike traditional automation tools that simply follow rigid scripts, these autonomous agents can understand context, make decisions, adapt to changing circumstances, and execute multi-step processes across various business systems. The implications for operational efficiency, cost reduction, and competitive advantage are profound and far-reaching.
The Evolution of Business Process Automation
From Basic Automation to Intelligent Autonomy
Business process automation has undergone several transformative phases over the decades. It began with simple rule-based systems that could handle repetitive tasks but required explicit programming for every scenario. These early automation tools improved efficiency but were inflexible and unable to adapt to changes without significant reprogramming. The introduction of Robotic Process Automation (RPA) expanded these capabilities, allowing software robots to mimic human interactions with digital systems and execute routine tasks across multiple applications.
However, traditional RPA systems still largely depend on structured data and predefined workflows. They struggle with exceptions, ambiguity, and unstructured information. This is where the evolution to intelligent autonomy becomes crucial. Modern AI systems like Operator can interpret natural language, understand content in context, recognize patterns in unstructured data, and make informed decisions based on complex criteria. This represents a fundamental shift from automation that requires constant human management to autonomy that can operate independently while still aligning with business objectives.
Core Capabilities of Operator AI
OpenAI's Operator represents the cutting edge of autonomous agent technology. Unlike previous AI assistants that primarily respond to direct prompts, Operator can proactively initiate and complete complex workflows across multiple systems. Its core capabilities include:
Natural Language Understanding: Operator can interpret instructions, documents, emails, and other text-based information with nuanced comprehension, enabling it to understand the intent behind requests and prioritize tasks accordingly.
Context-Aware Processing: The system maintains awareness of organizational context, including business rules, priorities, and operational constraints, allowing it to make appropriate decisions within its authority boundaries.
System Integration: Operator can connect with various business systems through APIs, including CRM platforms, project management tools, communication channels, and data repositories.
Task Planning and Execution: Perhaps most impressively, Operator can break down complex objectives into manageable steps, sequence them appropriately, and execute them across different platforms and tools.
Adaptive Learning: Through ongoing interactions and feedback, these agents continuously improve their performance, becoming more aligned with specific business needs and organizational preferences.
Transforming Business Processes Through Autonomous Agents
Customer Service and Support
Customer service represents one of the most immediate and impactful applications of autonomous agents. Operator AI can revolutionize support operations by managing the entire customer journey with minimal human intervention. These agents can field initial inquiries, categorize issues by urgency and complexity, retrieve relevant customer history, and either resolve straightforward problems immediately or route complex cases to appropriate human specialists with comprehensive context.
Beyond simple chatbot interactions, Operator can proactively monitor customer satisfaction signals, identify patterns in support requests that might indicate product issues, and even generate recommendations for service improvements. This proactive approach transforms customer support from a reactive cost center into a strategic function that enhances customer relationships and provides valuable business intelligence.
Companies implementing autonomous agents for customer support have reported resolution time reductions of up to 65% for common issues and significant improvements in customer satisfaction scores. The technology allows organizations to provide consistent 24/7 support without the costs associated with expanding human teams across multiple time zones.
Financial Operations and Reporting
Financial operations involve numerous repetitive yet critical processes that require accuracy, consistency, and compliance with regulatory standards. Operator AI excels in this environment by automating tasks such as invoice processing, payment reconciliation, expense approval workflows, and financial reporting. These agents can extract relevant information from various document formats, validate it against business rules, flag exceptions for human review, and route approvals through appropriate channels.
The impact on month-end close processes has been particularly significant. Companies implementing autonomous agents for financial operations report reducing close cycles by up to 40%, minimizing errors, and freeing finance professionals to focus on strategic analysis rather than data collection and reconciliation. The technology also enhances compliance by ensuring consistent application of policies and maintaining comprehensive audit trails of all transactions and decisions.
Supply Chain and Inventory Management
Supply chain operations represent complex ecosystems with numerous interdependent processes and stakeholders. Autonomous agents can transform these operations by coordinating activities across suppliers, logistics providers, warehouses, and distribution centers. Operator AI can continuously monitor inventory levels, predict demand fluctuations, generate purchase orders based on predefined rules, track shipments, and coordinate delivery schedules—all while adapting to disruptions in real-time.
When exceptions occur, such as supplier delays or unexpected demand spikes, these agents can evaluate alternative options, implement contingency plans, and notify relevant stakeholders with recommendations for addressing the situation. This autonomous coordination significantly reduces the manual effort required to manage supply chain operations while improving responsiveness to changing conditions.
Organizations implementing autonomous agents in supply chain management have achieved inventory reduction of 15-30% while maintaining or improving service levels. The technology has proven particularly valuable in industries with complex supply chains and seasonal demand fluctuations, such as retail, manufacturing, and consumer packaged goods.
Human Resources and Talent Management
HR departments handle numerous process-heavy tasks that can benefit from autonomous agent technology. Operator AI can transform recruitment by screening resumes, scheduling interviews, responding to candidate inquiries, and facilitating onboarding processes. For existing employees, these agents can manage leave requests, answer policy questions, collect performance feedback, and coordinate training activities.
Beyond transactional tasks, autonomous agents can enhance strategic HR functions by analyzing workforce data to identify retention risks, skill gaps, or engagement issues. They can also support managers with reminders for regular check-ins, performance reviews, and development conversations, ensuring consistent application of people management practices across the organization.
Companies implementing autonomous agents in HR functions report reducing administrative workload by up to 40%, allowing HR professionals to focus on strategic initiatives and high-value interactions with employees. The technology also improves the employee experience by providing immediate responses to common questions and streamlining access to services and information.
Implementation Strategies and Best Practices
Assessing Process Readiness for Autonomy
Not all business processes are equally suited for autonomous agent implementation. Organizations should evaluate their processes based on several criteria:
Frequency and Volume: Processes that occur regularly and at high volume typically offer better return on investment for automation and autonomy.
Complexity Balance: Ideal candidates have moderate complexity—too simple, and traditional automation may be sufficient; too complex, and the technology may not yet be ready for fully autonomous operation.
Data Availability: Processes with accessible digital data and clear decision parameters are easier to delegate to autonomous agents than those requiring extensive physical world interaction or highly subjective judgments.
Value Impact: Prioritize processes where improved speed, accuracy, or availability would significantly impact business outcomes or customer experience.
Regulatory Considerations: Evaluate compliance requirements and risk tolerance, particularly for processes in highly regulated industries or involving sensitive data.
A systematic assessment using these criteria helps organizations identify "quick win" opportunities for initial implementation while building a roadmap for more complex applications as the technology matures and organizational capabilities develop.
Building the Technological Foundation
Successful implementation of autonomous agents requires appropriate technological infrastructure. Key components include:
API Ecosystem: Robust APIs that allow the agent to interact with core business systems, including CRM platforms, ERP systems, communication tools, and data repositories.
Identity and Access Management: Secure mechanisms for granting and managing the agent's access to systems, with appropriate limitations and audit capabilities.
Data Integration Layer: Tools for consolidating and standardizing data from various sources to provide the agent with comprehensive context for decision-making.
Monitoring and Analytics: Systems for tracking agent performance, decisions, and outcomes to enable continuous improvement and ensure accountability.
Human-in-the-Loop Interfaces: Well-designed touchpoints for human oversight, intervention, and collaboration with the autonomous system.
Organizations should evaluate their existing technology stack against these requirements and develop a plan to address gaps before implementing autonomous agents at scale. This may involve upgrading legacy systems, implementing integration middleware, or adopting new tools designed specifically for AI-powered automation.
Change Management and Organizational Readiness
Perhaps the most significant challenge in implementing autonomous agents is preparing the organization for this fundamental shift in how work gets done. Effective change management should address:
Leadership Alignment: Ensuring executives and managers understand the technology's capabilities, limitations, and strategic implications.
Workforce Preparation: Communicating transparently about how roles will evolve and providing training for new skills needed to work effectively alongside autonomous systems.
Process Redesign: Thoughtfully reconfiguring workflows to leverage the strengths of both human workers and AI agents, rather than simply automating existing processes.
Governance Framework: Establishing clear policies for agent authority, escalation paths, performance standards, and accountability mechanisms.
Cultural Adaptation: Fostering a culture that embraces technological advancement while maintaining focus on human values and ethical considerations.
Organizations that invest in comprehensive change management significantly increase their success rates with autonomous agent implementation. Those that neglect these human factors often encounter resistance, underutilization, or misalignment with business objectives despite having sound technology.
Statistics & Real-World Impact
Understanding the quantifiable impact of autonomous agents helps organizations build compelling business cases and set realistic expectations. The following data highlights key trends and outcomes from early adopters across industries:
The statistics above illustrate the substantial business impact achieved by organizations implementing Operator AI across different sectors. Professional services and retail industries are seeing the highest ROI and adoption rates, likely due to their combination of standardized processes and significant potential for efficiency gains. Even sectors with more complex regulatory environments, such as healthcare and government, are achieving remarkable improvements in productivity and error reduction.
Challenges and Ethical Considerations
Balancing Autonomy and Oversight
As organizations deploy increasingly capable autonomous agents, they must carefully balance autonomy with appropriate human oversight. This challenge manifests in several dimensions:
Decision Authority: Determining which decisions the agent can make independently versus which require human approval remains a critical governance question. Organizations typically implement tiered authority models where agents have full autonomy for low-risk, routine decisions but require varying levels of human involvement as complexity and risk increase.
Performance Monitoring: While autonomous agents can operate independently, their performance must be continuously monitored to ensure alignment with business objectives and quality standards. Organizations need robust metrics and review processes to evaluate agent decisions and identify areas for improvement.
Exception Handling: Even the most sophisticated agents encounter situations beyond their capabilities. Well-designed systems incorporate clear escalation paths for exceptions, ensuring human experts can intervene when necessary without compromising the overall efficiency gains of the autonomous system.
Ethical Boundaries: Organizations must establish explicit ethical guidelines for autonomous agents, particularly regarding customer interactions, data usage, and decision criteria. These guidelines should reflect the organization's values and comply with relevant regulations.
Finding the right balance between autonomy and oversight requires ongoing calibration based on agent performance, business needs, and evolving technological capabilities. Organizations that thoughtfully navigate this balance can maximize the benefits of autonomous agents while managing associated risks.
Workforce Transformation and Human-AI Collaboration
Implementing autonomous agents inevitably transforms the nature of work within an organization. Managing this transformation effectively requires addressing several key considerations:
Role Evolution: Rather than eliminating jobs, autonomous agents typically transform them by automating routine aspects while creating new responsibilities around agent management, exception handling, and higher-value activities. Organizations should proactively define these evolved roles and create clear career paths in the AI-augmented environment.
Skill Development: Employees need new skills to work effectively alongside autonomous systems, including AI literacy, data analysis, process design, and complex problem-solving. Organizations should invest in comprehensive training programs to help their workforce develop these capabilities.
Collaborative Interfaces: The touchpoints between humans and autonomous agents significantly impact both effectiveness and employee experience. Well-designed interfaces should make agent capabilities transparent, enable smooth handoffs between automated and human work, and empower employees to guide and improve agent performance.
Cultural Adaptation: Successfully integrating autonomous agents requires cultural shifts toward embracing technological augmentation, valuing uniquely human capabilities, and fostering continuous learning. Leadership plays a crucial role in modeling these attitudes and creating psychological safety during the transformation.
Organizations that approach workforce transformation thoughtfully can create environments where humans and AI systems complement each other's strengths, resulting in outcomes superior to what either could achieve alone.
Future-Proofing: The Road Ahead for Autonomous Business Agents
The field of autonomous business agents is evolving rapidly, with several key trends shaping its future development:
Multi-Agent Ecosystems: As individual processes become increasingly automated, organizations will shift toward orchestrating ecosystems of specialized agents that collaborate on complex business objectives. These ecosystems will combine agents with different capabilities, authority levels, and areas of expertise.
Enhanced Learning Capabilities: Next-generation autonomous agents will incorporate more sophisticated learning mechanisms, enabling them to improve their performance based on outcomes, stakeholder feedback, and changing business conditions without explicit reprogramming.
Cross-Boundary Operation: While current implementations typically operate within organizational boundaries, future autonomous agents will increasingly work across organizational boundaries to coordinate activities with customers, suppliers, and partners throughout the business ecosystem.
Expanded Sensory Capabilities: Integration with IoT devices, computer vision, speech recognition, and other sensory technologies will expand the range of inputs available to autonomous agents, enabling them to operate more effectively in environments that blend digital and physical elements.
Organizations should develop their autonomous agent strategies with these emerging capabilities in mind, building flexible foundations that can incorporate new technologies as they mature and become commercially viable.
Conclusion: The Transformative Impact of Operator AI
The emergence of truly autonomous AI agents represents a pivotal moment in business technology evolution. OpenAI's Operator and similar technologies are transforming how organizations approach their operations, creating unprecedented opportunities for efficiency, scalability, and innovation. While conventional automation merely executed predefined processes, autonomous agents fundamentally reimagine what's possible by combining contextual understanding, decision-making capabilities, and cross-system coordination.
The competitive advantages for early adopters are substantial and multifaceted. Organizations implementing autonomous agents are achieving significant cost reductions while simultaneously improving service quality, consistency, and availability. They're liberating their human talent from routine tasks to focus on creative, strategic, and relationship-oriented work that drives greater value. Perhaps most importantly, they're building the organizational capabilities and technological foundations needed to thrive in an increasingly AI-augmented business landscape.
However, successful implementation requires more than just deploying the technology. Organizations must thoughtfully redesign their processes to leverage autonomous capabilities, prepare their workforce for changing roles, establish appropriate governance frameworks, and navigate the ethical considerations that arise with increasingly capable AI systems. Those that approach this transformation holistically will be best positioned to realize the full potential of autonomous agents while managing the associated challenges.
As we look to the future, the capabilities of autonomous business agents will continue to expand, enabling ever more sophisticated applications across industries. Organizations that begin their journey now—starting with well-defined use cases and building both technological capabilities and organizational readiness—will develop the experience and expertise needed to maintain their competitive edge as this transformative technology evolves.
The question for business leaders is no longer whether to implement autonomous agents, but how quickly and effectively they can harness this technology to transform their operations while preparing their organizations for an increasingly autonomous future.
Frequently Asked Questions
What is Operator AI and how does it differ from traditional automation? Operator AI is OpenAI's autonomous agent technology that can independently complete complex tasks across multiple systems. Unlike traditional automation that follows rigid scripts, Operator AI understands context, makes decisions, adapts to changing circumstances, and executes multi-step processes with minimal human supervision.
What business processes are best suited for Operator AI implementation? Processes with high frequency, moderate complexity, available digital data, and significant business impact are ideal for Operator AI. Examples include customer service, financial operations, supply chain management, and HR administrative tasks.
What ROI can businesses expect from implementing Operator AI? Based on early implementation data, businesses can expect ROI ranging from 195% to 325% annually, depending on the industry. Professional services and retail sectors show the highest returns, while government and healthcare sectors demonstrate more moderate but still substantial ROI.
How much does it cost to implement Operator AI in a business? Implementation costs typically range from $90,000 to $420,000, varying by industry complexity and scope. These costs include software licensing, system integration, training, and change management processes.
Does Operator AI eliminate the need for human workers? No, Operator AI transforms roles rather than eliminating them. It automates routine tasks, allowing employees to focus on higher-value work requiring creativity, emotional intelligence, strategic thinking, and complex problem-solving—areas where humans still excel over AI.
How secure is Operator AI when handling sensitive business data? Operator AI incorporates multiple security measures including robust encryption, access controls, audit logging, and compliance with industry standards. Enterprise implementations can operate in private cloud environments with customized security protocols to meet specific regulatory requirements.
How long does it take to implement Operator AI in an organization? Implementation timelines range from 3-6 months for initial use cases to 12-18 months for enterprise-wide deployment. The timeline depends on organizational readiness, system complexity, integration requirements, and change management processes.
Can Operator AI integrate with existing enterprise systems? Yes, Operator AI is designed to integrate with existing enterprise systems through APIs and middleware. It can connect with CRM platforms, ERP systems, communication tools, project management software, and custom applications, though some systems may require additional integration work.
What industries are seeing the fastest adoption of Operator AI? Professional services, retail, and financial services industries are leading in Operator AI adoption, with implementation rates of 89%, 85%, and 78% respectively. These sectors benefit from process standardization opportunities and clear ROI cases.
How can businesses prepare their workforce for Operator AI implementation? Businesses should invest in comprehensive change management including clear communication about how roles will evolve, training programs for new skills needed to work alongside AI, involvement of employees in process redesign, and fostering a culture that values both technological advancement and human expertise.
Additional Resources
For readers interested in exploring Operator AI and autonomous agents further, the following resources provide valuable insights and guidance:
"The Autonomous Enterprise: How AI Agents are Transforming Business Operations" by Harvard Business Review Press (2025) - A comprehensive examination of how AI agents are reshaping organizational structures and processes.
OpenAI's Official Operator Documentation and Implementation Guide - The authoritative technical resource for understanding Operator's capabilities and implementation requirements.
McKinsey Global Institute: "The Business Value of Autonomous AI" (March 2025) - Research report analyzing the economic impact of autonomous agents across industries and business functions.
"Working with AI: Human-Agent Collaboration in the Digital Workplace" by MIT Press (2024) - Explores effective approaches for designing collaborative relationships between human workers and AI systems.
World Economic Forum: "The Future of Jobs in the Age of Autonomous AI" (2025) - Analysis of how autonomous agents are transforming labor markets and skill requirements across the global economy.