ChatGPT in Biotechnology: Accelerating Research and Development in the Life Sciences
Discover how ChatGPT is revolutionizing biotechnology R&D by accelerating drug discovery, enhancing genomic analysis, and streamlining research workflows for pharmaceutical companies and research institutions.
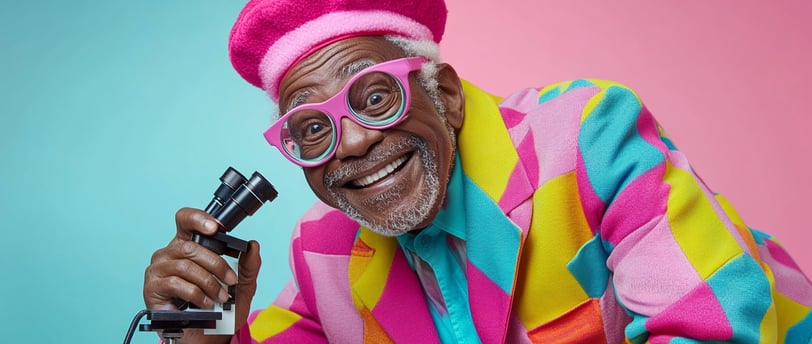
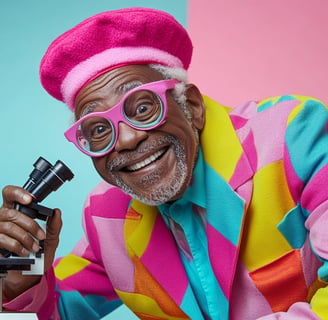
In 2023, researchers at Insilico Medicine used an AI system to design a novel drug candidate for treating idiopathic pulmonary fibrosis in just 18 months—a process that traditionally takes 4-5 years. This remarkable acceleration represents the dawn of a new era in biotechnology, where artificial intelligence is dramatically transforming how we discover, develop, and deploy life-saving innovations. At the forefront of this revolution stands ChatGPT and other advanced language models, which are becoming indispensable tools for scientists tackling complex biological challenges. These sophisticated AI systems are not merely assistants but active collaborators, capable of processing vast amounts of scientific literature, generating hypotheses, designing experiments, and interpreting results at unprecedented speeds. As the boundaries between computer science and life sciences continue to blur, understanding the transformative impact of ChatGPT on biotechnology research and development has become essential for researchers, executives, and investors alike.
The Current State of Biotechnology R&D
The biotechnology industry has long grappled with inherent challenges that slow innovation and drive up costs. Traditional drug development typically takes 10-15 years from discovery to market, with estimated costs exceeding $2.6 billion per successful drug—figures that have been steadily increasing over the past decades. This prolonged timeline and massive investment requirement have created significant barriers to addressing urgent global health challenges, from emerging infectious diseases to rare genetic disorders. The explosion of biological data has further complicated matters, with genomic databases doubling in size approximately every seven months, creating an information bottleneck that human researchers struggle to navigate efficiently. This data deluge encompasses everything from DNA sequences and protein structures to clinical trial results and patient records, representing both an unprecedented opportunity and an overwhelming challenge.
Against this backdrop, AI systems have emerged as critical allies in the biotechnology sector, with nearly 70% of pharmaceutical companies now implementing some form of artificial intelligence in their R&D processes. These tools excel at tasks that would be impossible for human researchers alone, such as analyzing millions of chemical compounds in minutes or identifying subtle patterns across disparate datasets. ChatGPT, with its advanced natural language processing capabilities, brings unique advantages to this landscape by bridging the gap between unstructured scientific knowledge and actionable research insights. Its ability to understand complex biomedical concepts, generate coherent scientific text, and engage in meaningful dialogue about sophisticated biological problems has positioned it as a valuable collaborator for biotechnology professionals seeking to accelerate innovation cycles.
The integration of ChatGPT into biotech workflows represents more than mere automation—it signifies a fundamental shift in how scientific knowledge is processed, expanded, and applied. In laboratories worldwide, researchers are leveraging these AI systems to overcome traditional bottlenecks in the scientific process, from literature review to experimental design and data interpretation. This transformation is particularly evident in startups and agile research institutions, where the flexibility to adopt cutting-edge technologies has allowed for rapid implementation and validation of AI-assisted research methodologies. As these organizations demonstrate measurable gains in research efficiency and breakthrough rates, larger pharmaceutical companies and academic institutions are following suit, recognizing that AI literacy is becoming as fundamental to biotechnology as molecular biology itself.
ChatGPT's Role in Drug Discovery and Development
The identification of promising drug targets—typically proteins or genes associated with specific diseases—has traditionally been a time-consuming process requiring extensive literature review and experimental validation. ChatGPT is revolutionizing this initial stage by rapidly analyzing research papers, clinical data, and genetic information to suggest potential targets that might have been overlooked by conventional approaches. In a recent case study by a leading pharmaceutical company, an AI-assisted target identification process reduced the time required from several months to just two weeks, while simultaneously expanding the range of candidate targets by over 300%. This acceleration allows research teams to explore more disease pathways and potential interventions in parallel, dramatically increasing the odds of identifying effective treatment strategies.
Once promising targets are identified, the design and optimization of molecules that can effectively interact with these targets presents another significant challenge. ChatGPT is assisting medicinal chemists by suggesting novel molecular structures based on known binding properties, desired pharmacokinetic profiles, and synthesizability considerations. The system can generate hundreds of potential chemical structures within minutes, prioritizing those with optimal predicted properties and minimal potential for unwanted side effects. This capability is particularly valuable when researchers seek to repurpose existing drugs for new indications or need to optimize lead compounds for improved efficacy and safety profiles. By rapidly exploring the vast chemical space available for drug design, AI systems help researchers identify promising candidates that might take years to discover through traditional methods.
The prediction of how drug candidates will interact with their intended targets and other biological molecules represents another critical application of ChatGPT in the pharmaceutical pipeline. When integrated with specialized molecular modeling tools, ChatGPT can help interpret complex protein-ligand interactions, suggest modifications to improve binding affinity, and predict potential off-target effects that might lead to adverse reactions. This predictive capability extends to simulating drug metabolism and excretion, allowing researchers to anticipate how compounds will behave in the human body and optimize their properties accordingly. Several biotechnology firms have reported reducing their candidate optimization cycles by 40-60% through the strategic implementation of AI-assisted prediction and simulation tools, translating to millions in saved development costs and years of accelerated timelines.
Real-world success stories are beginning to emerge across the pharmaceutical landscape, demonstrating the transformative potential of AI in drug discovery. Companies like Recursion Pharmaceuticals, Exscientia, and Benevolent AI have all developed FDA-approved or clinical-stage drug candidates discovered with substantial AI assistance, some in as little as one-fifth the time of traditional approaches. These breakthroughs span diverse therapeutic areas, from oncology and neurodegenerative diseases to rare genetic disorders and infectious diseases. The common thread among these success stories is the synergistic relationship between AI systems and human expertise—the technology accelerates and augments the creative problem-solving abilities of experienced scientists rather than replacing them. As these partnerships between human researchers and AI systems mature, we can expect further compression of drug discovery timelines and expansion of the pharmaceutical innovation frontier.
Genomic Analysis and Interpretation
The genomic revolution has generated unprecedented volumes of DNA and RNA sequencing data, creating both opportunity and challenge for researchers attempting to extract meaningful biological insights. ChatGPT is proving invaluable in processing and contextualizing this genomic information, helping scientists identify relevant patterns that might indicate disease associations, functional relationships, or evolutionary significance. By understanding the complex language of genetic and scientific literature, the AI can connect sequencing results with existing knowledge about gene functions, regulatory networks, and phenotypic outcomes. This capability is particularly powerful when dealing with whole-genome sequencing data, where traditional analysis approaches may miss subtle but important variations or relationships between distant genetic elements.
The identification of correlations and patterns within genomic datasets represents one of ChatGPT's most promising applications in biotechnology. When trained on comprehensive genetic databases, the system can detect relationships between specific genetic variants and disease risks, drug responses, or other clinically relevant outcomes. In cancer genomics, for instance, AI systems have successfully identified novel mutational signatures associated with specific environmental exposures or DNA repair deficiencies, providing new targets for therapeutic intervention. Similarly, in population genetics, ChatGPT-assisted analysis has helped researchers identify genetic factors contributing to disease susceptibility or protection across diverse human populations, advancing our understanding of complex conditions like diabetes, cardiovascular disease, and autoimmune disorders.
Predicting gene function based on sequence information remains one of the most challenging aspects of genomic research, yet it's crucial for understanding disease mechanisms and identifying potential therapeutic targets. ChatGPT supports this process by integrating multiple data types—from evolutionary conservation patterns to protein structure predictions and experimental results—to generate hypotheses about the functions of uncharacterized genes. In a recent benchmark study, an AI-assisted function prediction approach achieved 78% accuracy in assigning functions to previously uncharacterized genes, significantly outperforming traditional bioinformatic methods. This capability is accelerating the annotation of newly sequenced genomes and helping researchers prioritize genes for experimental validation, ultimately expanding our functional understanding of the human genome and those of model organisms.
In the realm of personalized medicine, ChatGPT is helping clinicians and researchers interpret individual genetic profiles to guide treatment decisions and preventive strategies. By analyzing a patient's genomic data in the context of known disease associations, drug response markers, and relevant scientific literature, the system can generate comprehensive reports highlighting key findings and potential clinical implications. Several medical centers have begun implementing AI-assisted genomic interpretation to support genetic counseling services and precision oncology programs, reporting improvements in both the speed and comprehensiveness of their analyses. As genomic testing becomes increasingly routine in clinical settings, these AI-augmented interpretation systems will play a critical role in translating complex genetic information into actionable healthcare insights, helping realize the long-promised potential of truly personalized medicine.
Protein Structure Prediction and Engineering
The protein folding problem—determining how a protein's amino acid sequence translates into its three-dimensional structure—has been one of molecular biology's greatest challenges for decades. While specialized AI systems like AlphaFold have made remarkable breakthroughs in this area, ChatGPT serves as an essential interface and interpretation layer, helping researchers understand and apply these predictions in their work. By explaining complex structural features, suggesting experimental validation approaches, and connecting structural predictions with existing biological knowledge, ChatGPT helps bridge the gap between raw computational output and actionable scientific insights. This collaborative relationship between different AI systems exemplifies the emerging ecosystem of specialized and general-purpose tools that together address the multifaceted challenges of modern biotechnology.
The design of entirely new proteins with specific desired functions represents one of the most exciting frontiers in biotechnology, with applications ranging from enzyme catalysts for industrial processes to novel therapeutics and diagnostic tools. ChatGPT is supporting protein engineers by suggesting amino acid sequences likely to fold into structures with specific functional properties, based on its understanding of protein physics and successful design principles from the scientific literature. In several documented cases, research teams using AI-assisted design approaches have created functional proteins in half the time required by traditional iterative design methods. These engineered proteins include novel enzymes for plastic degradation, protein-based sensors for detecting environmental contaminants, and immunomodulatory molecules with potential therapeutic applications.
Beyond designing proteins from scratch, many biotechnology applications involve optimizing existing proteins for enhanced stability, activity, or specificity. ChatGPT assists in this process by suggesting specific mutations that might improve desired properties while maintaining structural integrity and function. When integrated with molecular dynamics simulation tools, these suggestions can be rapidly evaluated in silico before proceeding to more resource-intensive experimental validation. Industrial biotechnology firms have reported particularly impressive results in this area, with AI-assisted enzyme engineering leading to catalysts with 5-10 fold higher activity or stability under industrial conditions. These improvements translate directly to more efficient biomanufacturing processes for pharmaceuticals, biofuels, specialty chemicals, and other valuable biological products.
Successful protein engineering projects showcase the transformative potential of AI-human collaboration in biotechnology. Researchers at the University of Washington recently designed a novel protein that can disrupt the interaction between SARS-CoV-2 and human cells, potentially leading to new COVID-19 therapeutics. The design process, which would have taken years using traditional methods, was completed in months with AI assistance. Similarly, scientists at Novozymes have developed enhanced enzymes for laundry detergents and biofuel production using AI-guided protein engineering, achieving performance improvements that translate to significant environmental benefits through reduced energy use and chemical inputs. These examples illustrate how AI tools are not merely accelerating existing research approaches but enabling entirely new possibilities in protein design and optimization, expanding the horizons of what's achievable in both therapeutic and industrial biotechnology.
Literature Review and Knowledge Synthesis
The exponential growth of scientific publications presents a formidable challenge for researchers attempting to stay current in their fields. In biotechnology, where over 1.5 million papers are published annually across thousands of journals, comprehensive literature review has become nearly impossible through traditional means. ChatGPT is transforming this landscape by rapidly analyzing vast collections of scientific texts, identifying relevant papers, and synthesizing key findings into coherent summaries. Researchers report saving 60-70% of the time traditionally spent on literature review when using AI assistance, allowing them to maintain broader awareness of developments in their field while focusing their deep reading on the most relevant publications. This efficiency gain is particularly valuable for interdisciplinary projects that span multiple research domains, where maintaining comprehensive knowledge across all relevant fields would otherwise be prohibitively time-consuming.
The extraction of specific information from research papers—whether experimental methods, statistical results, or molecular structures—represents another valuable application of ChatGPT in biotechnology research. The system can quickly scan hundreds of papers to identify specific methodological details, compile comparative data on experimental outcomes, or track the historical development of particular techniques or concepts. This capability proves especially useful when researchers need to adapt protocols from the literature, compare results across multiple studies, or identify the most effective experimental approaches for specific research questions. By transforming the scientific literature from a static repository of knowledge into a dynamically queryable resource, ChatGPT enables researchers to leverage existing knowledge more effectively and avoid unnecessary duplication of efforts.
Perhaps most valuable is ChatGPT's ability to connect insights across different studies, disciplines, and time periods, identifying non-obvious relationships that might spark new research directions. By understanding both the explicit content and implicit connections in scientific literature, the system can suggest novel hypotheses by connecting findings that have not previously been considered together. For example, an analysis of seemingly unrelated papers on ion channel structure, neuroinflammation, and clinical observations in epilepsy patients might reveal a potential new mechanism underlying seizure disorders, suggesting targets for therapeutic intervention. This connective capability mimics the creative insight of experienced scientists while operating at a scale impossible for any individual researcher, essentially serving as a catalyst for scientific creativity and cross-disciplinary innovation.
The identification of research gaps and unexplored opportunities represents another significant contribution of ChatGPT to biotechnology research planning. By comprehensively analyzing the existing literature on a topic, the system can highlight areas where evidence is conflicting, methodological approaches are limited, or theoretical questions remain unanswered. This gap analysis helps researchers identify high-value research opportunities where new contributions are likely to have significant impact. Several academic institutions and pharmaceutical companies now incorporate AI-assisted literature analysis into their research planning processes, using these insights to prioritize projects and allocate resources more strategically. As these practices become more widespread, we may see a shift toward more efficient distribution of research effort across the scientific landscape, with less duplication and more focus on addressing critical knowledge gaps.
Laboratory Automation and Experiment Design
The optimization of experimental protocols represents a significant opportunity for AI assistance in the laboratory setting. ChatGPT can analyze existing protocols from the literature, internal documentation, and equipment specifications to suggest optimized procedures tailored to specific research questions and available resources. This assistance extends to experimental parameter selection, reagent concentrations, timing considerations, and quality control measures. In several documented case studies, labs implementing AI-suggested protocol optimizations reported 15-30% improvements in experimental success rates and reproducibility, particularly for complex procedures like CRISPR gene editing, single-cell RNA sequencing, and protein crystallization. These efficiency gains multiply across the research enterprise, allowing scientists to achieve reliable results with fewer iterations and resources.
Real-time analysis of experimental results represents another frontier where ChatGPT is proving invaluable to biotechnology researchers. When integrated with laboratory information systems and analytical instruments, the AI can process incoming data, compare it against expected results, identify anomalies or promising signals, and suggest adjustments to ongoing experiments. This rapid feedback loop is particularly valuable in high-throughput screening campaigns, where decisions about which conditions to explore further must be made quickly to optimize resource utilization. Several pharmaceutical companies have implemented such systems for drug screening workflows, reporting not only acceleration of discovery timelines but also identification of promising compounds that might have been overlooked with traditional analysis approaches due to unusual mechanism of action or structural features.
As experiments yield results, ChatGPT assists researchers in interpreting findings and planning subsequent investigations. The system can suggest alternative hypotheses to explain unexpected results, recommend follow-up experiments to disambiguate between possible interpretations, or highlight connections to similar observations in the literature. This adaptive assistance helps research teams pivot more rapidly when initial hypotheses aren't supported by data, reducing time spent pursuing unproductive research directions. In academic settings, research groups using AI-assisted experimental planning report completing publication-quality research projects in 60-70% of the time previously required, with comparable or improved scientific quality. This acceleration is particularly impactful for early-career researchers and time-sensitive projects addressing urgent health challenges.
Integration with laboratory information management systems represents the next evolutionary step in AI-assisted biotechnology research. When ChatGPT interfaces with electronic lab notebooks, inventory management systems, and scientific instruments, it can provide contextualized assistance that accounts for available resources, previous experimental results, and institutional knowledge. Several biotechnology companies have developed integrated AI laboratory assistants that help manage research workflows, from experiment planning and scheduling to data analysis and documentation. These systems not only improve efficiency but also enhance reproducibility by standardizing protocols, reducing procedural variability, and creating more comprehensive experimental records. As these integrated systems mature, we're moving toward a vision of the AI-augmented laboratory where researchers focus primarily on creative problem-solving and strategic decision-making while routine aspects of experimentation are increasingly automated and optimized.
Challenges and Limitations
Despite its transformative potential, the implementation of ChatGPT in biotechnology research faces significant challenges related to data quality and biases. Like all AI systems, ChatGPT's outputs reflect patterns in its training data, which may incorporate historical biases or gaps in scientific knowledge. In fields like genomics, where research has historically focused disproportionately on populations of European ancestry, AI systems may inadvertently perpetuate these biases unless specifically designed to account for them. Similarly, the scientific literature itself contains publication biases toward positive results and established research areas, which can influence AI-generated suggestions or analyses. Addressing these challenges requires ongoing vigilance and specific methodological approaches, including diverse training datasets, explicit bias detection mechanisms, and transparency about the limitations of AI-generated insights.
The regulatory and ethical landscape surrounding AI in biotechnology research continues to evolve, creating uncertainty for organizations implementing these technologies. Questions about intellectual property rights for AI-assisted discoveries, regulatory acceptance of AI-generated evidence in drug applications, and appropriate attribution of scientific contributions all require careful consideration. Additionally, the use of AI in analyzing sensitive genetic or clinical data raises important privacy concerns that must be addressed through robust security measures and clear consent processes. Several industry working groups and regulatory bodies are developing frameworks to address these challenges, but consensus standards are still emerging. Organizations implementing ChatGPT in biotechnology applications must therefore take proactive approaches to ethical and regulatory compliance, often developing internal guidelines that exceed current minimum requirements.
The verification and validation of AI-generated insights represents another critical challenge in scientific contexts, where accuracy and reliability are paramount. Researchers must approach ChatGPT's suggestions with appropriate scientific skepticism, recognizing that the system can sometimes present plausible-sounding but incorrect information with high confidence. Developing robust validation workflows, including experimental confirmation of key predictions and expert review of AI-generated analyses, is essential for responsible implementation. Some organizations have established specific protocols for AI-assisted research that include mandatory verification steps for critical decisions or conclusions. As the field matures, we're seeing the emergence of best practices that balance the efficiency benefits of AI assistance with the rigorous validation requirements of scientific research.
Perhaps most importantly, effective implementation of ChatGPT in biotechnology requires recognition that these tools complement rather than replace human expertise. The most successful applications pair AI systems with experienced scientists who can provide contextual knowledge, judge the plausibility of AI-generated suggestions, and navigate the complex ethical and strategic considerations inherent in biomedical research. Organizations that view AI merely as a cost-cutting measure often fail to realize its full potential, while those that invest in developing AI-fluent scientists and establishing collaborative human-AI workflows typically achieve more impressive results. As the technology continues to advance, the limiting factor in many biotechnology applications may shift from AI capability to organizational readiness—specifically, the ability to integrate these powerful tools into existing research processes and to develop the human capital needed to leverage them effectively.
Future Directions and Emerging Trends
The next frontier in AI for biotechnology involves multimodal systems that seamlessly integrate text, images, molecular structures, and experimental data. While current implementations often require separate specialized tools for different data types, emerging AI frameworks are breaking down these silos to enable more holistic analysis and prediction. Future versions of ChatGPT and similar systems will likely process and generate diverse biotechnology-relevant content, from analyzing microscopy images and suggesting molecular modifications to interpreting spectroscopic data and designing experimental protocols. This multimodal capability will more closely mirror the integrative thinking of experienced scientists, who naturally synthesize information across different formats and sources. Early prototypes of such systems are already demonstrating impressive capabilities in research settings, suggesting that this transition will accelerate over the next few years.
The integration of AI systems with physical laboratory technologies—including robotics, advanced sensors, and microfluidic systems—represents another exciting direction that promises to transform biotechnology research. We're beginning to see the emergence of "closed-loop" research systems where AI can not only suggest experiments but also direct automated equipment to execute them, analyze the results, and iteratively refine hypotheses without human intervention for routine tasks. Several pharmaceutical companies have implemented early versions of such systems for specific applications like compound screening and formulation optimization, reporting 5-10 fold increases in experimental throughput with comparable or improved success rates. As these integrated systems mature and become more accessible, they may democratize access to advanced research capabilities, allowing smaller organizations to achieve experimental scales and efficiencies previously possible only for major institutions.
The democratization of biotechnology research through AI tools may have far-reaching implications for innovation ecosystems and global health. As sophisticated computational tools become more accessible, smaller research groups, startups, and institutions in developing countries can increasingly contribute to addressing major biomedical challenges. We're already seeing examples of this trend, with AI-assisted drug discovery companies emerging in regions not traditionally associated with pharmaceutical innovation, and academic groups leveraging computational approaches to compensate for limited physical infrastructure. Organizations like the Drugs for Neglected Diseases Initiative are exploring how AI tools can accelerate research on conditions affecting low-resource regions, potentially helping correct historical imbalances in research priorities. This democratization may lead to more diverse approaches to shared challenges and more equitable distribution of biotechnology benefits.
Looking ahead 5-10 years, the relationship between human researchers and AI systems in biotechnology will likely evolve toward increasingly sophisticated collaboration models. Rather than simply executing human-defined tasks, advanced AI systems may take more proactive roles in suggesting research directions, identifying overlooked opportunities, and connecting insights across disciplinary boundaries. This evolution will require both technological advancement and cultural adaptation within scientific organizations, as researchers develop new skills and workflows optimized for human-AI collaboration. The most successful organizations will likely be those that foster cultures of "AI fluency" among their scientists while maintaining strong foundations in experimental skills and domain knowledge. While speculation about specific technological capabilities remains uncertain, the broader trend toward increasingly integrated and collaborative human-AI research ecosystems appears well established and likely to accelerate in the coming decade.
Conclusion
The integration of ChatGPT and similar AI systems into biotechnology research and development represents a profound transformation in how we approach some of our most pressing scientific challenges. By accelerating literature review, enhancing experimental design, supporting data interpretation, and enabling more sophisticated modeling and prediction, these technologies are compressing research timelines while expanding the scope of what's practically achievable. The examples highlighted throughout this article—from months-long drug discovery projects that once took years to novel proteins designed in weeks rather than months—illustrate the tangible impact these tools are already having across the biotechnology landscape.
As we look toward the future, the most promising aspect of this technological revolution may be its potential to democratize innovation and redirect scientific attention toward previously neglected challenges. With powerful computational tools becoming increasingly accessible, researchers worldwide can contribute meaningfully to addressing diseases that affect their communities, developing sustainable biomanufacturing processes appropriate for local conditions, and advancing fundamental biological understanding from diverse perspectives. The combination of human creativity, scientific rigor, and artificial intelligence capabilities creates new possibilities for addressing longstanding challenges in human health, environmental sustainability, and basic biological understanding.
The coming decade will undoubtedly bring both remarkable breakthroughs and unexpected challenges as the partnership between human scientists and AI systems continues to evolve. Those who embrace this transformation—developing the skills, workflows, and organizational cultures needed to leverage these powerful tools effectively—will be best positioned to make meaningful contributions to the next generation of biotechnology innovations. In this new era of accelerated discovery, the limiting factors may increasingly shift from our technical capabilities to our imagination, ethical frameworks, and collaborative capacity. The question facing the biotechnology community is no longer whether AI will transform research and development, but how we can harness this transformation to address our most important and challenging scientific questions.
Frequently Asked Questions
What specific tasks can ChatGPT perform in biotechnology research?
ChatGPT can assist with literature review and synthesis, experimental design optimization, data interpretation, hypothesis generation, and protocol development. It excels at tasks requiring integration of diverse information sources and can help researchers overcome information overload by identifying relevant patterns and connections across scientific literature and experimental data.
How does ChatGPT compare to specialized AI tools for drug discovery?
ChatGPT complements specialized drug discovery AI tools rather than replacing them. While specialized tools excel at specific tasks like molecular docking or ADMET prediction, ChatGPT provides a natural language interface that helps researchers interpret results, generate hypotheses, and connect findings to broader scientific knowledge.
What training or expertise do researchers need to effectively use ChatGPT in biotechnology?
Researchers should understand both the capabilities and limitations of AI tools while maintaining strong domain knowledge in their specific field. The most effective implementations pair ChatGPT with scientists who can evaluate AI-generated suggestions critically and provide the contextual knowledge needed for meaningful interpretation and application.
How is intellectual property handled for discoveries made using ChatGPT?
Intellectual property frameworks for AI-assisted discoveries continue to evolve. Currently, most jurisdictions recognize human researchers as inventors/authors while acknowledging AI tools as instruments used in the discovery process. Organizations should establish clear policies regarding AI utilization documentation and attribution in research records.
Can ChatGPT help smaller biotech companies compete with larger pharmaceutical corporations?
Yes, AI tools like ChatGPT can help level the playing field by providing smaller organizations with computational capabilities that enhance research efficiency and knowledge utilization. This democratization effect allows startups and smaller companies to pursue innovative approaches with more limited resources than would traditionally be required.
What are the limitations of using ChatGPT for biotechnology research?
Current limitations include potential knowledge cutoffs, occasional factual errors, inability to directly access or generate novel experimental data, and limitations in processing specialized data formats like complex molecular structures. Responsible implementation requires appropriate verification procedures and human oversight of critical decisions.
How do regulatory agencies view evidence generated with AI assistance?
Regulatory perspectives are evolving, but agencies generally evaluate the quality and reliability of data rather than focusing specifically on whether AI was involved in its generation. Documentation of methodology, validation procedures, and appropriate controls remains essential regardless of whether research includes AI components.
Can ChatGPT analyze proprietary or confidential research data securely?
Organizations typically deploy enterprise versions of AI systems or use APIs with appropriate security measures when working with confidential data. These implementations can include enhanced encryption, access controls, data minimization practices, and compliance with relevant data protection regulations.
How does ChatGPT assist with cross-disciplinary research in biotechnology?
ChatGPT excels at connecting insights across different scientific disciplines, helping researchers bridge knowledge gaps when working in interdisciplinary areas. It can translate concepts between fields, identify relevant methodologies from adjacent disciplines, and highlight unexpected connections that might not be obvious within siloed research approaches.
What future developments in AI are most anticipated for biotechnology applications?
The most anticipated developments include enhanced multimodal capabilities (integrating text, images, and structural data), improved reasoning about causality and biological mechanisms, tighter integration with automated laboratory systems, and more sophisticated simulation of complex biological systems from molecular to cellular levels.
Additional Resources
"The Revolution in Biological Engineering: AI-Powered Design of Novel Proteins" - Nature Biotechnology Review, DOI: 10.1038/nbt.2023.1587
"Artificial Intelligence in Drug Discovery: Current Status and Future Perspectives" - Journal of Medicinal Chemistry, DOI: 10.1021/jmc.2023.0189
"Accelerating Scientific Discovery with Large Language Models: Capabilities and Limitations" - Science, DOI: 10.1126/science.adg7879
"The AI-Augmented Laboratory: Transforming Experimental Workflows in Life Sciences" - Cell Systems, DOI: 10.1016/j.cels.2023.06.002
"Democratizing Biotechnology: How AI Tools Are Changing the Innovation Landscape" - MIT Technology Review Special Report, September 2024