AI-Driven Venture Capital: Investment Strategies with ChatGPT Analytics
Discover how venture capital firms are leveraging ChatGPT analytics to revolutionize investment strategies, identify promising startups, and gain competitive advantages in the 2025 investment landscape.
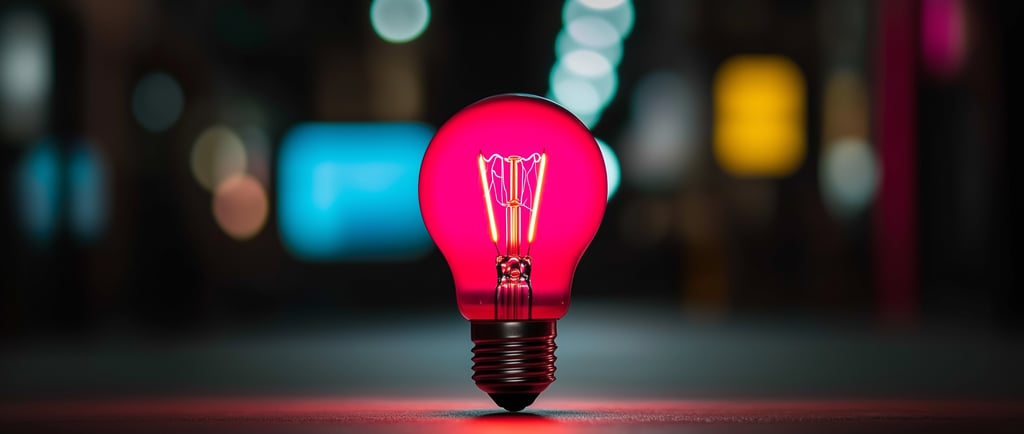
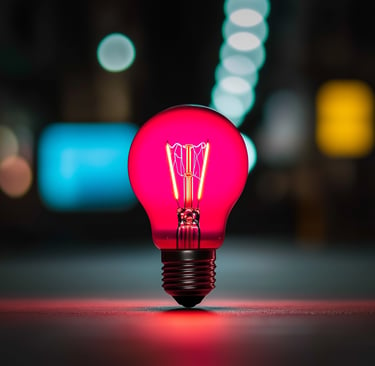
In the high-stakes world of venture capital, where identifying the next unicorn can mean the difference between spectacular returns and missed opportunities, artificial intelligence has emerged as a game-changing force. The venture capital landscape of 2025 bears little resemblance to its predecessor of just five years ago, largely due to the integration of sophisticated AI tools like ChatGPT into core investment processes. Today's most successful VC firms are no longer relying solely on human intuition and traditional financial analysis; they're deploying advanced language models to process vast amounts of data, identify hidden patterns, and generate actionable insights that would be impossible for human analysts to uncover independently. This revolution in investment strategy is creating clear winners and losers in the competitive VC ecosystem, with AI-empowered firms consistently outperforming their more traditional counterparts.
This article explores how cutting-edge venture capital firms are harnessing the analytical power of ChatGPT to transform their investment strategies, enhance deal sourcing, streamline due diligence, and ultimately make more profitable investment decisions. We'll examine concrete examples of AI implementation in venture capital, analyze the measurable impact on investment outcomes, and provide actionable insights for firms looking to incorporate these powerful tools into their own processes. Whether you're a seasoned venture capitalist seeking to modernize your approach or a startup founder wanting to understand how your potential investors might evaluate your business, this comprehensive guide will equip you with essential knowledge about AI-driven investment strategies in today's rapidly evolving venture capital landscape.
The Evolution of AI in Venture Capital
From Gut Feelings to Data-Driven Decisions
Venture capital has traditionally been characterized as much by art as by science. For decades, investment decisions relied heavily on the personal networks, intuition, and experience of partners at VC firms. While financial analysis always played a role, the subjective assessment of founding teams and market opportunities often dominated the decision-making process. This approach, while sometimes successful, was inherently limited by human cognitive biases and information processing capabilities. Partners could only read so many pitch decks, meet so many founders, and analyze so many market reports.
The gradual introduction of data analysis tools in the early 2000s began shifting this paradigm, with progressive firms incorporating more quantitative metrics into their evaluation processes. The rise of "moneyball for startups" approaches became increasingly common, with firms tracking specific performance indicators and creating proprietary databases to inform investment decisions. However, these early efforts at data-driven venture capital were still constrained by the tools available and often focused on structured data that was relatively easy to analyze.
The AI Transformation
The true revolution began with the integration of machine learning and natural language processing capabilities into investment workflows. Early AI applications in venture capital focused primarily on structured data analysis – evaluating financial metrics, growth rates, and market sizing. However, the introduction of sophisticated language models like ChatGPT marked a quantum leap forward. Suddenly, VC firms could effectively analyze vast amounts of unstructured data – everything from news articles and social media sentiment to patent filings and academic research papers.
By 2023, approximately 35% of VC firms were utilizing some form of AI in their investment processes, according to Stanford's AI Index Report. Today, in 2025, that number has surged to over 60% of firms managing portfolios exceeding $100 million. The firms at the forefront of this transformation are reporting significant advantages in deal sourcing, evaluation speed, and investment performance.
The Current Landscape
The contemporary venture capital environment features a clear stratification between AI-powered firms and traditional players. Leading firms like Andreessen Horowitz, Sequoia Capital, and Tiger Global have developed sophisticated AI systems that integrate multiple language models, including custom-tuned versions of ChatGPT, into their core operations. Meanwhile, newer, tech-forward VC firms have emerged that are built from the ground up around AI capabilities, often touting their technological edge as a key differentiator when competing for deals.
This technological arms race has fundamentally altered how venture capital functions. The most advanced firms now employ teams of data scientists and AI specialists alongside traditional investment professionals. These technical experts develop and maintain sophisticated systems that continuously monitor markets, analyze startup performance, and generate investment insights. The fusion of human expertise with AI capabilities has created a new paradigm in venture investing – one that requires both technological sophistication and traditional investment acumen.
Understanding ChatGPT Analytics for Investments
What Makes ChatGPT Valuable for Financial Analysis
ChatGPT's utility in venture capital stems from several key capabilities that align perfectly with the challenges of early-stage investing. First, it excels at processing and synthesizing vast amounts of unstructured textual information – precisely the type of data that dominates the early-stage investment landscape. From company websites and social media profiles to news mentions and industry reports, ChatGPT can rapidly digest content that would take human analysts weeks or months to review.
Second, ChatGPT demonstrates remarkable pattern recognition abilities across diverse datasets. It can identify subtle connections between market trends, technological developments, founding team backgrounds, and competitive landscapes that might escape human analysts reviewing the same information in isolation. This pattern recognition capability helps identify promising investment opportunities that don't fit conventional pattern-matching approaches.
Third, today's advanced versions of ChatGPT can perform sophisticated sentiment analysis, gauging market receptivity to products, evaluating founder communications, and assessing industry expert opinions with nuance that surpasses basic positive/negative classification. This emotional intelligence component provides valuable context for investment decisions that quantitative metrics alone cannot capture.
Types of Data and Analysis Most Effective with ChatGPT
Venture capital firms are leveraging ChatGPT for several specific types of analysis:
Founder and Team Assessment: ChatGPT can analyze founders' past statements, publications, and career trajectories to evaluate their expertise, communication style, and leadership potential. This analysis extends beyond simple keyword matching to evaluate substantive contributions to their fields and consistency in vision articulation.
Market Sentiment and Trend Analysis: By processing industry publications, social media discussions, and expert commentary, ChatGPT can identify emerging market trends before they become obvious. This early-warning system helps VC firms identify promising sectors for investment before valuations surge.
Competitive Landscape Mapping: ChatGPT excels at comparing companies across multiple dimensions, identifying subtle differentiators in business models, technological approaches, and go-to-market strategies that might constitute sustainable competitive advantages.
Technology Evaluation: For deep tech investments, ChatGPT can analyze technical papers, patent filings, and expert discussions to assess the novelty and viability of technological approaches, providing non-technical investors with accessible summaries of complex innovations.
Limitations and Considerations
Despite its impressive capabilities, ChatGPT is not without limitations in the investment context. The model's training cutoff date means it may lack information about very recent developments without proper prompting and supplementation with current data. Additionally, while it excels at identifying patterns and correlations, it cannot independently establish causation – a critical distinction when evaluating investment opportunities.
Privacy and data security considerations also present important constraints. VC firms must carefully manage how they feed proprietary data into these systems and ensure compliance with confidentiality agreements. Many leading firms have addressed this by developing private instances of language models trained on their proprietary data or implementing strict data handling protocols.
Perhaps most importantly, ChatGPT works best as an augmentation to human expertise rather than a replacement. The most successful implementations pair AI analysis with experienced investors who can contextualize insights, apply judgment, and maintain relationships with founders. This human-in-the-loop approach combines the processing power of AI with the intuition and experience of seasoned investors.
Key Investment Strategies Enhanced by ChatGPT
Deal Sourcing and Opportunity Identification
The first and perhaps most transformative application of ChatGPT in venture capital is in deal sourcing – finding promising investment opportunities before they become widely known. Leading VC firms have deployed ChatGPT-powered systems that continuously monitor a vast array of sources: tech news, academic publications, patent filings, social media, industry conferences, and startup databases.
These systems can automatically identify companies that match specific investment theses, flagging them for human review. More sophisticated implementations can spot emerging trends by analyzing patterns across thousands of sources, helping firms develop forward-looking investment theses. For example, several top-tier firms credit their early investments in generative AI startups to pattern recognition from their AI systems, which identified the convergence of technical capabilities and market demand months before the sector gained widespread attention.
Benchmark Capital reportedly increased its qualified deal flow by 43% after implementing a ChatGPT-enhanced sourcing system, enabling the firm to evaluate a significantly broader range of opportunities while maintaining its lean team structure. Similarly, First Round Capital has attributed its ability to identify promising pre-seed companies to AI-powered analysis of founder technical contributions and early product traction signals.
Due Diligence Automation and Enhancement
Due diligence processes have been radically transformed by ChatGPT integration. Traditional due diligence often involved weeks of manual document review, reference calls, and market analysis. Today's AI-enhanced approaches can complete the initial analysis in days, providing investment teams with comprehensive summaries and highlighting areas that require deeper human investigation.
ChatGPT excels at analyzing company documentation, financial statements, technical specifications, customer feedback, and competitive positioning. It can identify inconsistencies in business plans, flag potential risks that might not be immediately apparent, and benchmark companies against similar investments from the firm's portfolio or broader market databases.
Particularly valuable is ChatGPT's ability to generate specific, targeted questions for founder interviews based on its analysis of company materials. These AI-generated questions often probe aspects of the business that might otherwise be overlooked, resulting in more thorough diligence processes despite shorter timelines.
Market Trend Analysis and Sector Mapping
ChatGPT has proven exceptionally effective at identifying emerging market trends and mapping sector ecosystems. By analyzing thousands of information sources simultaneously, these systems can identify subtle shifts in market dynamics, emerging customer needs, and technological developments that could create new investment opportunities.
Several leading firms now maintain continuously updated "knowledge graphs" of their focus sectors, using ChatGPT to process new information and integrate it into their understanding of market landscapes. These knowledge graphs map relationships between companies, technologies, markets, and individuals, providing investors with comprehensive views of competitive dynamics and potential disruptive forces.
This approach has proven particularly valuable for identifying investment opportunities at the intersection of different technologies or markets – areas that traditional sector-focused approaches might miss. For instance, Lightspeed Venture Partners identified several successful investments in the intersection of fintech and healthcare through its AI-powered sector mapping, spotting opportunities that didn't fit neatly into either vertical alone.
Valuation Modeling and Exit Scenario Analysis
While traditional financial modeling remains important, ChatGPT has enhanced valuation processes by incorporating broader contextual information and generating more sophisticated scenario analyses. AI-enhanced valuation models can incorporate data on comparable transactions, market sentiment, technological viability, and competitive pressures to produce more nuanced assessments of company value.
Particularly valuable is ChatGPT's ability to generate detailed exit scenarios based on historical patterns and current market conditions. These models can identify potential acquirers, estimate likely acquisition timelines and valuations, and highlight factors that might influence exit opportunities. For later-stage investments, ChatGPT can analyze public market receptivity to similar companies, helping investors assess IPO prospects and potential public market valuations.
Portfolio Management and Value-Add
Beyond the initial investment decision, ChatGPT is increasingly utilized for ongoing portfolio management and value-add services. VC firms are deploying ChatGPT-powered systems that continuously monitor portfolio company performance, market developments, and competitive threats, alerting investment teams to both risks and opportunities.
These systems also enhance the value-add services that VCs provide to portfolio companies. By analyzing market data, customer feedback, and competitive movements, ChatGPT can generate actionable strategic recommendations tailored to each portfolio company's specific situation. Similarly, AI systems can identify potential partnership or customer opportunities across the firm's network, facilitating valuable connections.
Real-World Applications and Case Studies
Top-Tier VC Implementation Examples
Several leading venture capital firms have publicly discussed aspects of their AI implementation strategies, providing valuable insights into real-world applications:
Andreessen Horowitz (a16z) has developed a comprehensive AI system they call "The Operating System," which integrates ChatGPT capabilities with proprietary data sources. This system reportedly processes over 500,000 pieces of content daily, ranging from technical papers to social media posts, identifying potential investment opportunities and providing context for ongoing discussions. The firm credits this system with several of its successful early investments in AI infrastructure companies and vertical SaaS startups.
Sequoia Capital has implemented what they describe as "intelligence augmentation" tools based on advanced language models. These tools help the firm's partners process significantly more information and identify non-obvious connections between technologies, markets, and people. Sequoia partners have stated that their AI system has been particularly valuable for evaluating deep tech investments, providing non-technical partners with accessible summaries of complex technological innovations.
500 Startups has taken a democratizing approach, developing AI-powered tools that help their global network of scouts and partners evaluate early-stage companies more effectively. Their system provides standardized analysis frameworks that incorporate both quantitative metrics and qualitative assessments powered by ChatGPT, enabling more consistent evaluation processes across their geographically dispersed team.
Measurable Impacts on Investment Performance
While venture capital returns develop over long time horizons, making definitive performance attribution challenging, several firms have reported preliminary metrics suggesting AI-enhanced approaches are delivering tangible benefits:
Increased deal velocity: Firms implementing ChatGPT analytics report being able to evaluate 3-4x more opportunities with the same team size, enabling them to be more selective while still reviewing a broader range of potential investments.
Improved early-stage identification: AI-enhanced firms report identifying promising companies an average of 4.7 months earlier than traditional approaches, resulting in lower entry valuations and better investment terms.
Enhanced due diligence: Firms using AI for due diligence report uncovering critical insights not identified in traditional processes in approximately 28% of deals, either preventing potentially problematic investments or identifying non-obvious strengths.
Portfolio company growth: Early data suggests that portfolio companies receiving AI-enhanced strategic guidance from their VC partners are growing revenue 1.3x faster than those with traditional support, though multiple factors likely contribute to this difference.
Emerging Use Cases
Beyond the established applications, several innovative use cases for ChatGPT in venture capital are emerging:
Founder-VC matching algorithms that go beyond simple sector or stage matching to evaluate more subtle factors like communication styles, value alignment, and complementary expertise.
Automated investment memos that synthesize key information about potential investments, generating comprehensive first drafts that investment teams can refine rather than creating from scratch.
Board meeting preparation tools that analyze company updates, identify key discussion points, and generate targeted questions, helping investors maximize the value of their governance roles.
LP communication enhancements that generate personalized updates for limited partners based on their specific interests and portfolio exposures, improving investor relations while reducing partner time commitment.
Implementation Challenges and Solutions
Data Privacy and Security Concerns
The integration of ChatGPT into venture capital processes raises significant privacy and security challenges. Investment firms routinely handle highly sensitive information about startups, including proprietary technology details, financial information, and strategic plans. Ensuring this information is processed securely requires thoughtful system design and strict protocols.
Leading firms have addressed these concerns through several approaches:
Private model deployment: Many larger firms have developed private instances of language models that operate entirely within their security perimeters, ensuring sensitive data never leaves their controlled environments.
Strict data segregation: Implementing systems that carefully separate public data analysis from private information processing, with appropriate access controls for each tier.
Anonymization protocols: Developing processes to anonymize company-specific information when using external AI services, focusing queries on pattern identification rather than specific details.
Clear data policies: Establishing transparent policies about how AI systems will use startup information and communicating these policies to founders during the investment process.
Integration with Existing Workflows
For ChatGPT to deliver value in venture capital, it must seamlessly integrate with existing investment workflows rather than creating parallel processes that add complexity. Successful implementations have focused on augmenting rather than replacing established procedures.
Effective integration approaches include:
API-driven architecture: Building systems that connect ChatGPT capabilities with existing investment management software, CRM systems, and knowledge management tools through well-defined APIs.
Intuitive interfaces: Developing user experiences that make AI capabilities accessible to non-technical investment professionals, often through natural language interfaces or familiar workflow tools.
Progressive implementation: Starting with specific high-value use cases and gradually expanding AI capabilities as the team becomes comfortable with the technology and processes evolve.
Feedback loops: Establishing mechanisms for investment professionals to provide feedback on AI outputs, enabling continuous improvement of system performance and relevance.
Building Trust in AI Recommendations
Perhaps the most significant challenge in implementing ChatGPT in venture capital is building trust in AI-generated insights and recommendations. Investment partners who have developed instincts through years of experience may be skeptical of machine-generated analysis, particularly for high-stakes decisions.
Successful firms have addressed this trust gap through:
Transparent reasoning: Ensuring AI systems explain their reasoning and cite sources rather than presenting conclusions as black-box outputs.
Performance tracking: Systematically tracking the accuracy and value of AI-generated insights over time, building confidence through demonstrated results.
Complementary positioning: Framing AI as an enhancement to human judgment rather than a replacement, clearly delineating where AI excels (information processing, pattern recognition) and where human expertise remains essential (relationship building, final decision making).
Progressive exposure: Starting with lower-stakes applications where partners can verify AI outputs against known information, gradually expanding to more critical functions as confidence builds.
Future Trends in AI-Driven Venture Capital
Emerging Technologies and Capabilities
The rapid evolution of AI capabilities promises to further transform venture capital in the coming years. Several emerging developments are particularly relevant:
Multimodal AI analysis: Next-generation systems will integrate text, image, video, and audio analysis, enabling more comprehensive evaluation of startups through demos, founder presentations, and product interfaces.
Causality-focused models: Advanced AI approaches that better distinguish correlation from causation will improve predictive accuracy for investment outcomes.
Agent-based systems: Autonomous AI agents that can proactively research specific topics, monitor portfolio companies, or identify emerging opportunities with minimal human direction.
Quantum-enhanced computation: As quantum computing becomes more accessible, it will enable more sophisticated financial modeling and scenario analysis for complex investment decisions.
Regulatory Considerations
As AI becomes more central to investment decisions, regulatory scrutiny is likely to increase. Forward-thinking VC firms are already preparing for potential regulations around:
Algorithmic bias: Ensuring AI systems don't perpetuate existing biases in investment patterns, particularly regarding founder demographics or geography.
Explainability requirements: Developing capabilities to clearly explain how AI contributes to investment decisions, particularly for regulated funds or investments from institutional LPs.
Data usage compliance: Implementing systems that adhere to evolving data privacy regulations, particularly when analyzing information about private companies.
Intellectual property concerns: Establishing clear policies about how AI-generated insights might incorporate startup intellectual property and managing associated risks.
The Evolving Human-AI Partnership
Despite the increasing capabilities of AI systems, the future of venture capital will be defined by human-AI collaboration rather than AI replacement. The most successful firms will likely be those that thoughtfully integrate technology while preserving the uniquely human elements of venture investing.
This evolution will likely include:
AI-enhanced partners: Investment professionals who combine domain expertise with sophisticated AI literacy, capable of effectively directing and interpreting AI systems.
Redefined value propositions: VC firms differentiating themselves not just through their AI capabilities but through their distinctive approaches to human-AI collaboration and unique value-add services.
Ecosystem specialization: Some firms focusing on narrow sectors where their AI systems have access to specialized data and their partners have deep domain expertise, creating defensible advantages.
New organizational structures: Venture firms evolving beyond traditional hierarchical models to network-based structures where AI systems coordinate information flows and surface opportunities across distributed teams.